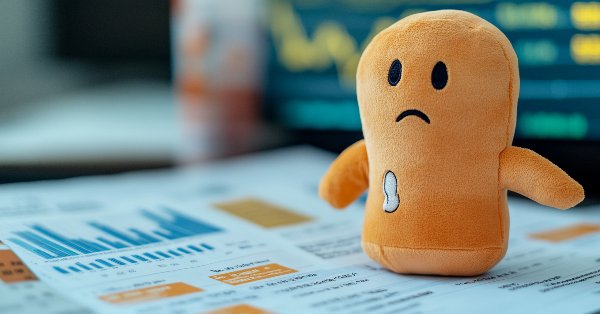
Data Science and ML (Part 37): Using Candlestick patterns and AI to beat the market
Candlestick patterns help traders understand market psychology and identify trends in financial markets, they enable more informed trading decisions that can lead to better outcomes. In this article, we will explore how to use candlestick patterns with AI models to achieve optimal trading performance.
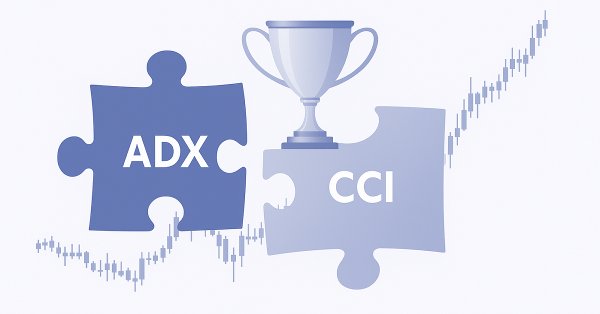
MQL5 Wizard Techniques you should know (Part 61): Using Patterns of ADX and CCI with Supervised Learning
The ADX Oscillator and CCI oscillator are trend following and momentum indicators that can be paired when developing an Expert Advisor. We look at how this can be systemized by using all the 3 main training modes of Machine Learning. Wizard Assembled Expert Advisors allow us to evaluate the patterns presented by these two indicators, and we start by looking at how Supervised-Learning can be applied with these Patterns.
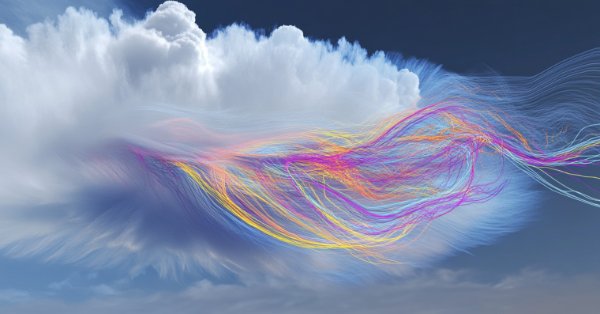
Atmosphere Clouds Model Optimization (ACMO): Practice
In this article, we will continue diving into the implementation of the ACMO (Atmospheric Cloud Model Optimization) algorithm. In particular, we will discuss two key aspects: the movement of clouds into low-pressure regions and the rain simulation, including the initialization of droplets and their distribution among clouds. We will also look at other methods that play an important role in managing the state of clouds and ensuring their interaction with the environment.
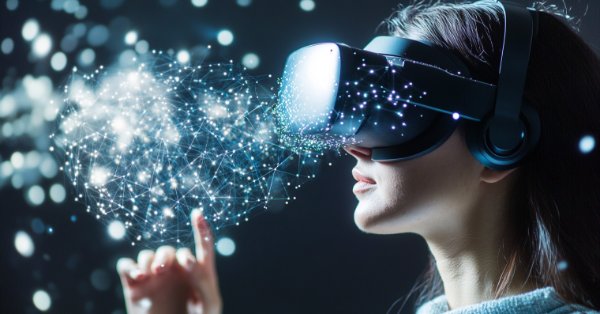
Neural Networks in Trading: Exploring the Local Structure of Data
Effective identification and preservation of the local structure of market data in noisy conditions is a critical task in trading. The use of the Self-Attention mechanism has shown promising results in processing such data; however, the classical approach does not account for the local characteristics of the underlying structure. In this article, I introduce an algorithm capable of incorporating these structural dependencies.
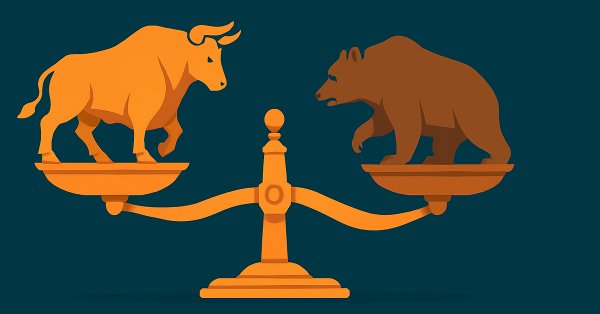
Data Science and ML (Part 36): Dealing with Biased Financial Markets
Financial markets are not perfectly balanced. Some markets are bullish, some are bearish, and some exhibit some ranging behaviors indicating uncertainty in either direction, this unbalanced information when used to train machine learning models can be misleading as the markets change frequently. In this article, we are going to discuss several ways to tackle this issue.
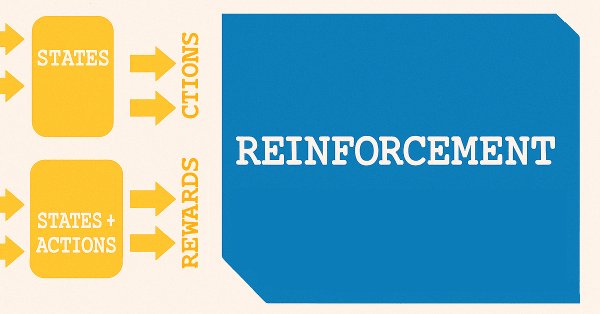
MQL5 Wizard Techniques you should know (Part 60): Inference Learning (Wasserstein-VAE) with Moving Average and Stochastic Oscillator Patterns
We wrap our look into the complementary pairing of the MA & Stochastic oscillator by examining what role inference-learning can play in a post supervised-learning & reinforcement-learning situation. There are clearly a multitude of ways one can choose to go about inference learning in this case, our approach, however, is to use variational auto encoders. We explore this in python before exporting our trained model by ONNX for use in a wizard assembled Expert Advisor in MetaTrader.
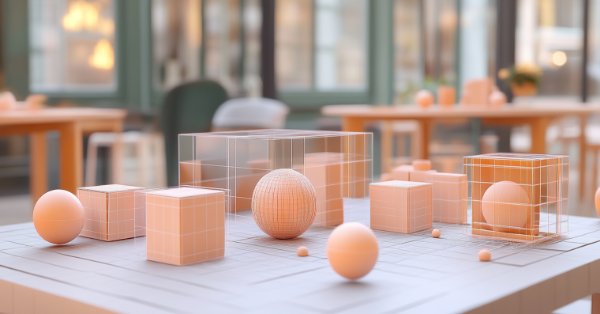
Neural Networks in Trading: Scene-Aware Object Detection (HyperDet3D)
We invite you to get acquainted with a new approach to detecting objects using hypernetworks. A hypernetwork generates weights for the main model, which allows taking into account the specifics of the current market situation. This approach allows us to improve forecasting accuracy by adapting the model to different trading conditions.
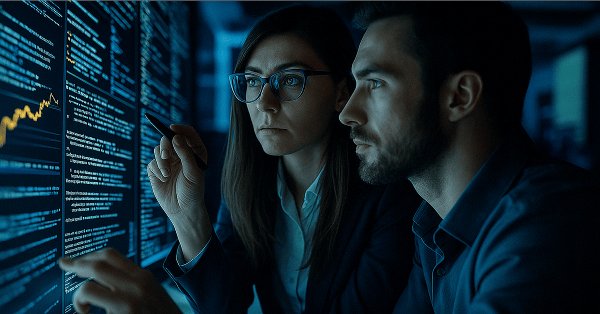
Reimagining Classic Strategies (Part 14): High Probability Setups
High probability Setups are well known in our trading community, but regrettably they are not well-defined. In this article, we will aim to find an empirical and algorithmic way of defining exactly what is a high probability setup, identifying and exploiting them. By using Gradient Boosting Trees, we demonstrated how the reader can improve the performance of an arbitrary trading strategy and better communicate the exact job to be done to our computer in a more meaningful and explicit manner.
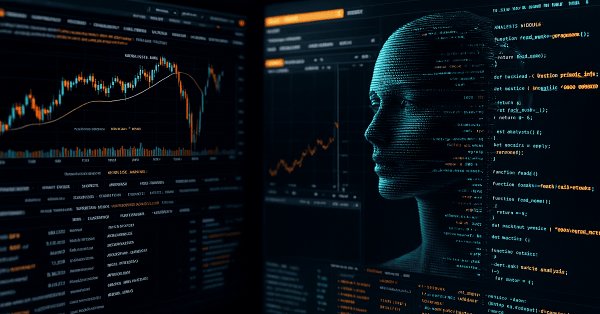
Integrating AI model into already existing MQL5 trading strategy
This topic focuses on incorporating a trained AI model (such as a reinforcement learning model like LSTM or a machine learning-based predictive model) into an existing MQL5 trading strategy.
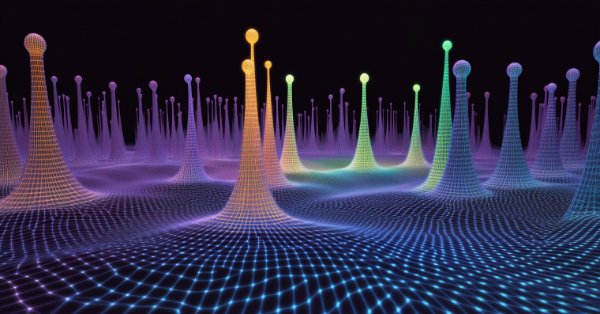
Neural Networks in Trading: Transformer for the Point Cloud (Pointformer)
In this article, we will talk about algorithms for using attention methods in solving problems of detecting objects in a point cloud. Object detection in point clouds is important for many real-world applications.
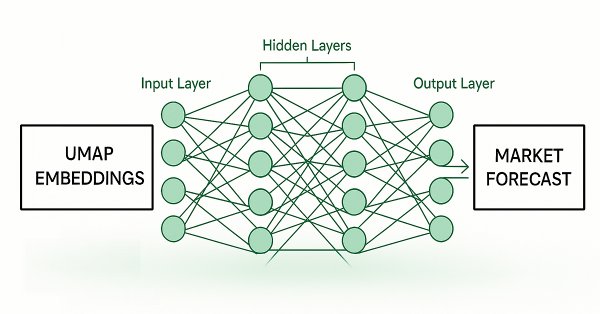
Feature Engineering With Python And MQL5 (Part IV): Candlestick Pattern Recognition With UMAP Regression
Dimension reduction techniques are widely used to improve the performance of machine learning models. Let us discuss a relatively new technique known as Uniform Manifold Approximation and Projection (UMAP). This new technique has been developed to explicitly overcome the limitations of legacy methods that create artifacts and distortions in the data. UMAP is a powerful dimension reduction technique, and it helps us group similar candle sticks in a novel and effective way that reduces our error rates on out of sample data and improves our trading performance.
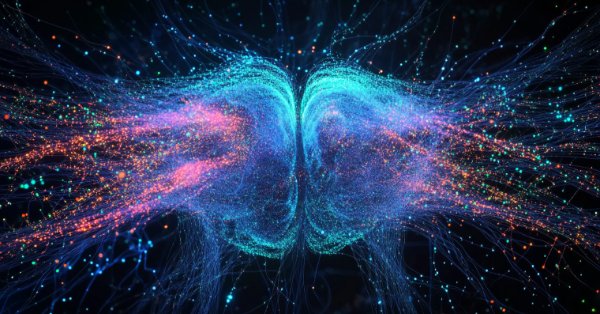
Neural Networks in Trading: Hierarchical Feature Learning for Point Clouds
We continue to study algorithms for extracting features from a point cloud. In this article, we will get acquainted with the mechanisms for increasing the efficiency of the PointNet method.
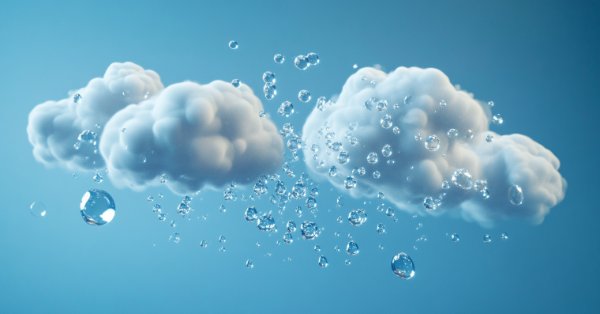
Atmosphere Clouds Model Optimization (ACMO): Theory
The article is devoted to the metaheuristic Atmosphere Clouds Model Optimization (ACMO) algorithm, which simulates the behavior of clouds to solve optimization problems. The algorithm uses the principles of cloud generation, movement and propagation, adapting to the "weather conditions" in the solution space. The article reveals how the algorithm's meteorological simulation finds optimal solutions in a complex possibility space and describes in detail the stages of ACMO operation, including "sky" preparation, cloud birth, cloud movement, and rain concentration.
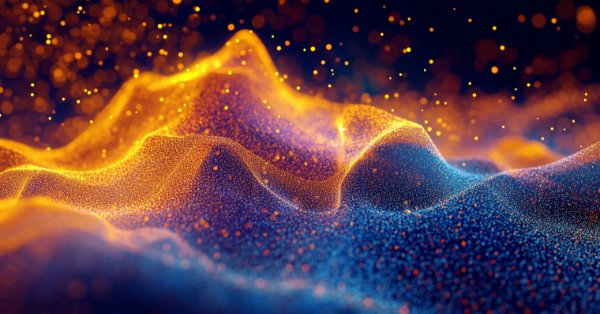
Neural Networks in Trading: Point Cloud Analysis (PointNet)
Direct point cloud analysis avoids unnecessary data growth and improves the performance of models in classification and segmentation tasks. Such approaches demonstrate high performance and robustness to perturbations in the original data.
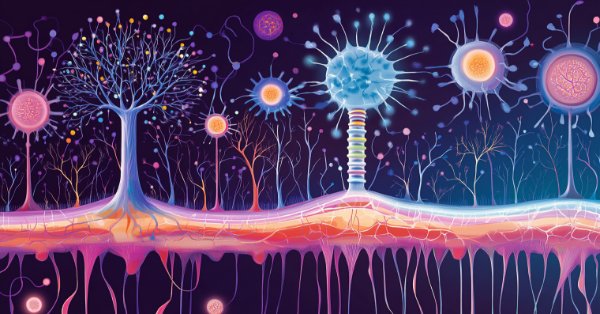
Neural Networks in Trading: Hierarchical Vector Transformer (Final Part)
We continue studying the Hierarchical Vector Transformer method. In this article, we will complete the construction of the model. We will also train and test it on real historical data.
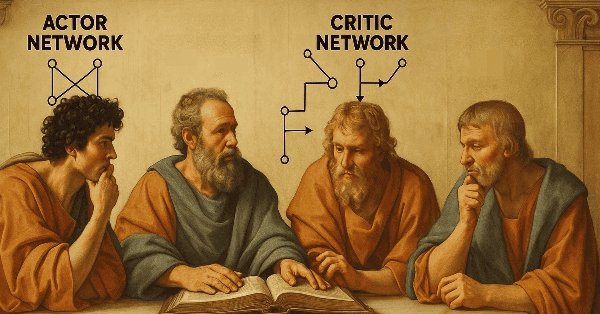
MQL5 Wizard Techniques you should know (Part 59): Reinforcement Learning (DDPG) with Moving Average and Stochastic Oscillator Patterns
We continue our last article on DDPG with MA and stochastic indicators by examining other key Reinforcement Learning classes crucial for implementing DDPG. Though we are mostly coding in python, the final product, of a trained network will be exported to as an ONNX to MQL5 where we integrate it as a resource in a wizard assembled Expert Advisor.
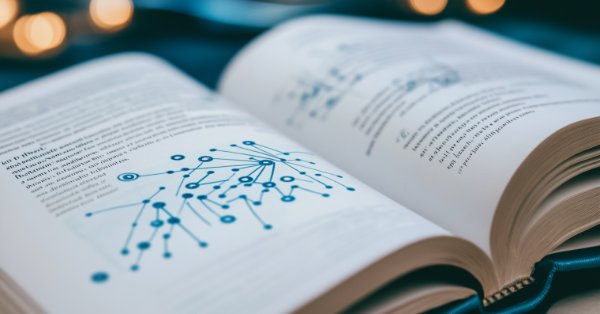
Neural Network in Practice: The First Neuron
In this article, we'll start building something simple and humble: a neuron. We will program it with a very small amount of MQL5 code. The neuron worked great in my tests. Let's go back a bit in this series of articles about neural networks to understand what I'm talking about.
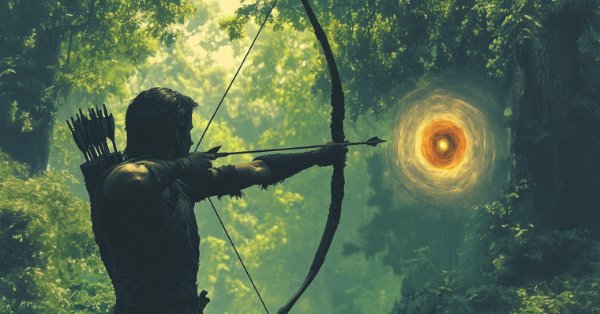
Archery Algorithm (AA)
The article takes a detailed look at the archery-inspired optimization algorithm, with an emphasis on using the roulette method as a mechanism for selecting promising areas for "arrows". The method allows evaluating the quality of solutions and selecting the most promising positions for further study.
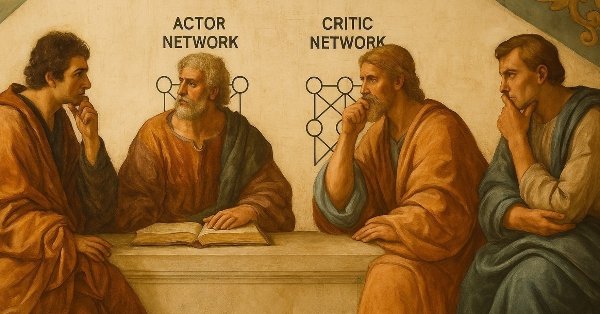
MQL5 Wizard Techniques you should know (Part 58): Reinforcement Learning (DDPG) with Moving Average and Stochastic Oscillator Patterns
Moving Average and Stochastic Oscillator are very common indicators whose collective patterns we explored in the prior article, via a supervised learning network, to see which “patterns-would-stick”. We take our analyses from that article, a step further by considering the effects' reinforcement learning, when used with this trained network, would have on performance. Readers should note our testing is over a very limited time window. Nonetheless, we continue to harness the minimal coding requirements afforded by the MQL5 wizard in showcasing this.
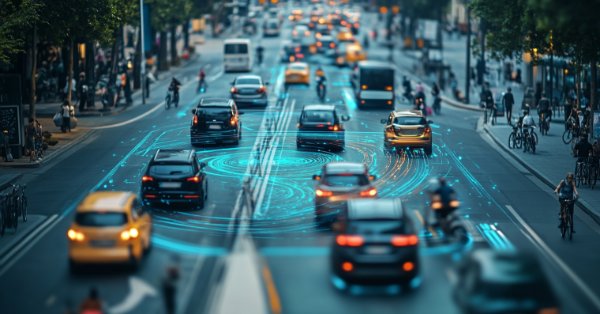
Neural Networks in Trading: Hierarchical Vector Transformer (HiVT)
We invite you to get acquainted with the Hierarchical Vector Transformer (HiVT) method, which was developed for fast and accurate forecasting of multimodal time series.
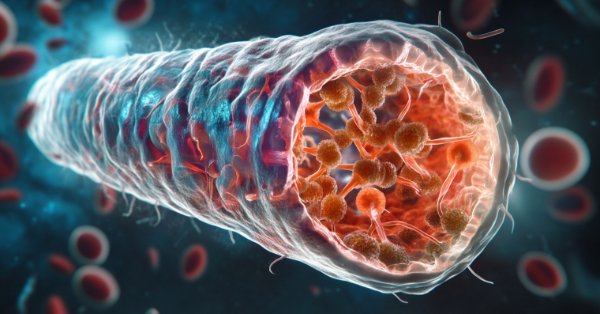
Bacterial Chemotaxis Optimization (BCO)
The article presents the original version of the Bacterial Chemotaxis Optimization (BCO) algorithm and its modified version. We will take a closer look at all the differences, with a special focus on the new version of BCOm, which simplifies the bacterial movement mechanism, reduces the dependence on positional history, and uses simpler math than the computationally heavy original version. We will also conduct the tests and summarize the results.
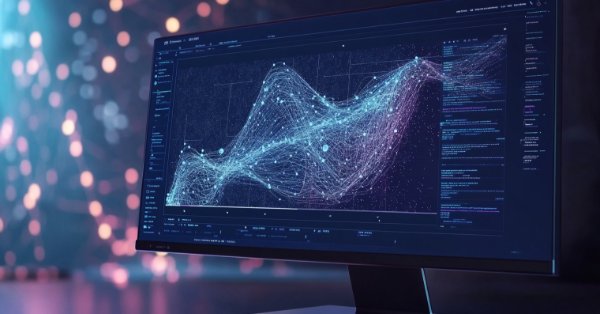
Neural Networks in Trading: Unified Trajectory Generation Model (UniTraj)
Understanding agent behavior is important in many different areas, but most methods focus on just one of the tasks (understanding, noise removal, or prediction), which reduces their effectiveness in real-world scenarios. In this article, we will get acquainted with a model that can adapt to solving various problems.
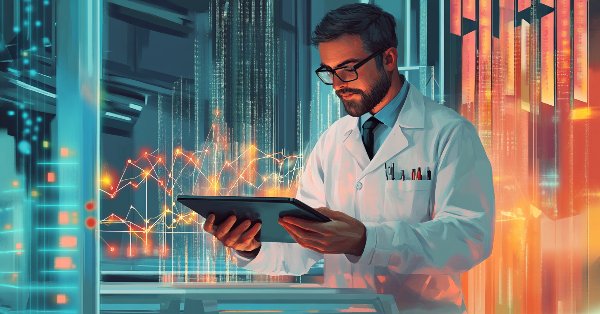
Data Science and ML (Part 35): NumPy in MQL5 – The Art of Making Complex Algorithms with Less Code
NumPy library is powering almost all the machine learning algorithms to the core in Python programming language, In this article we are going to implement a similar module which has a collection of all the complex code to aid us in building sophisticated models and algorithms of any kind.
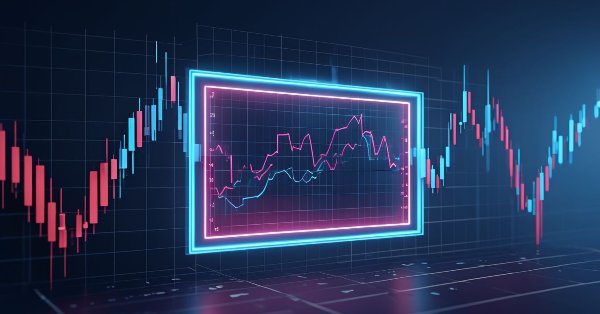
Exploring Advanced Machine Learning Techniques on the Darvas Box Breakout Strategy
The Darvas Box Breakout Strategy, created by Nicolas Darvas, is a technical trading approach that spots potential buy signals when a stock’s price rises above a set "box" range, suggesting strong upward momentum. In this article, we will apply this strategy concept as an example to explore three advanced machine learning techniques. These include using a machine learning model to generate signals rather than to filter trades, employing continuous signals rather than discrete ones, and using models trained on different timeframes to confirm trades.
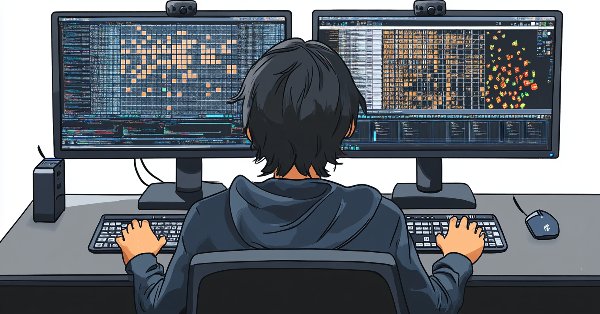
Resampling techniques for prediction and classification assessment in MQL5
In this article, we will explore and implement, methods for assessing model quality that utilize a single dataset as both training and validation sets.
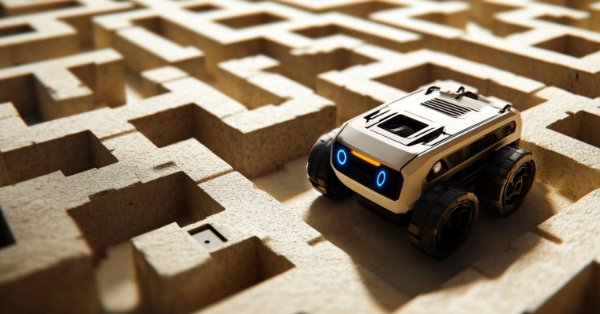
Tabu Search (TS)
The article discusses the Tabu Search algorithm, one of the first and most well-known metaheuristic methods. We will go through the algorithm operation in detail, starting with choosing an initial solution and exploring neighboring options, with an emphasis on using a tabu list. The article covers the key aspects of the algorithm and its features.
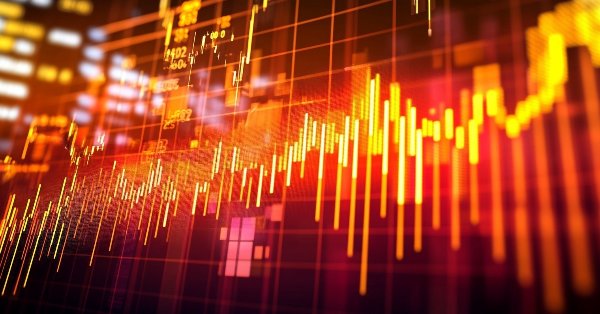
MQL5 Wizard Techniques you should know (Part 57): Supervised Learning with Moving Average and Stochastic Oscillator
Moving Average and Stochastic Oscillator are very common indicators that some traders may not use a lot because of their lagging nature. In a 3-part ‘miniseries' that considers the 3 main forms of machine learning, we look to see if this bias against these indicators is justified, or they might be holding an edge. We do our examination in wizard assembled Expert Advisors.
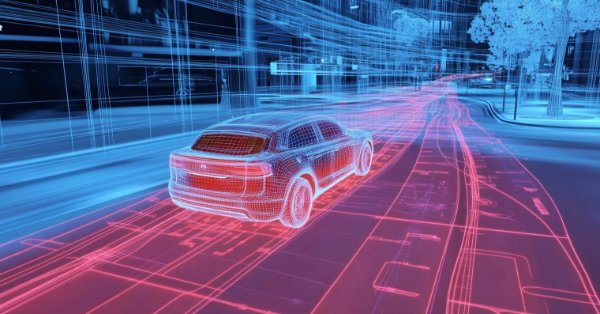
Neural Networks in Trading: A Complex Trajectory Prediction Method (Traj-LLM)
In this article, I would like to introduce you to an interesting trajectory prediction method developed to solve problems in the field of autonomous vehicle movements. The authors of the method combined the best elements of various architectural solutions.
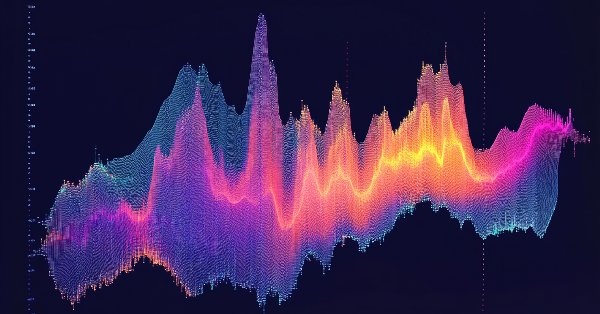
Data Science and ML (Part 34): Time series decomposition, Breaking the stock market down to the core
In a world overflowing with noisy and unpredictable data, identifying meaningful patterns can be challenging. In this article, we'll explore seasonal decomposition, a powerful analytical technique that helps separate data into its key components: trend, seasonal patterns, and noise. By breaking data down this way, we can uncover hidden insights and work with cleaner, more interpretable information.
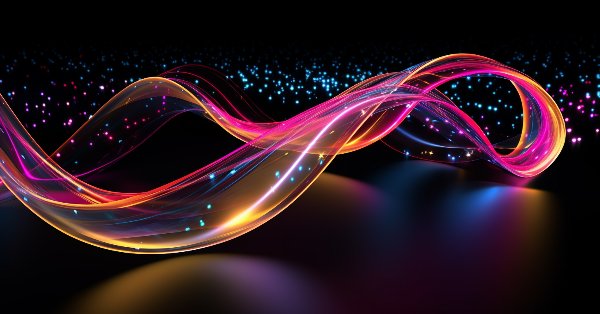
An introduction to Receiver Operating Characteristic curves
ROC curves are graphical representations used to evaluate the performance of classifiers. Despite ROC graphs being relatively straightforward, there exist common misconceptions and pitfalls when using them in practice. This article aims to provide an introduction to ROC graphs as a tool for practitioners seeking to understand classifier performance evaluation.
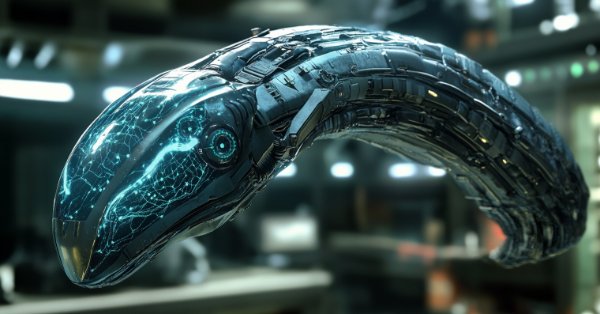
Neural Networks in Trading: State Space Models
A large number of the models we have reviewed so far are based on the Transformer architecture. However, they may be inefficient when dealing with long sequences. And in this article, we will get acquainted with an alternative direction of time series forecasting based on state space models.
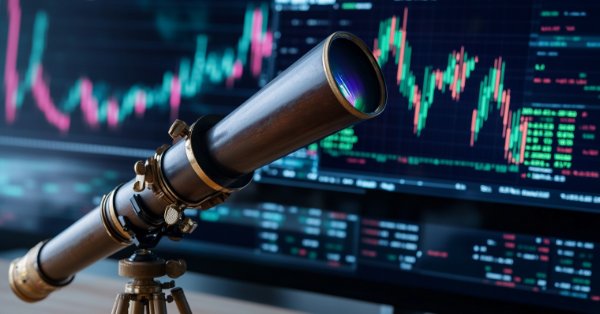
William Gann methods (Part III): Does Astrology Work?
Do the positions of planets and stars affect financial markets? Let's arm ourselves with statistics and big data, and embark on an exciting journey into the world where stars and stock charts intersect.
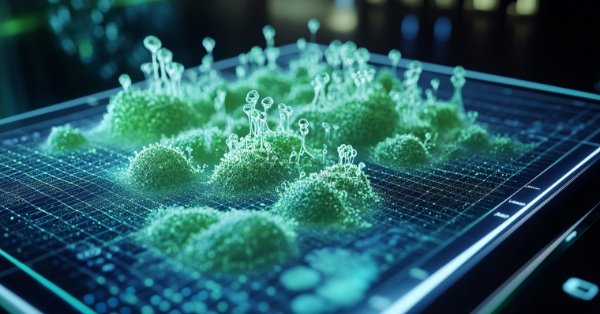
Artificial Algae Algorithm (AAA)
The article considers the Artificial Algae Algorithm (AAA) based on biological processes characteristic of microalgae. The algorithm includes spiral motion, evolutionary process and adaptation, which allows it to solve optimization problems. The article provides an in-depth analysis of the working principles of AAA and its potential in mathematical modeling, highlighting the connection between nature and algorithmic solutions.
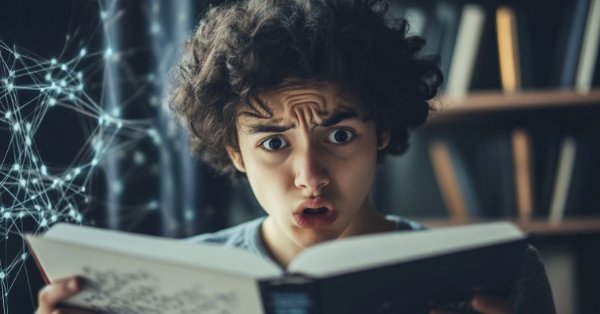
Neural Network in Practice: Sketching a Neuron
In this article we will build a basic neuron. And although it looks simple, and many may consider this code completely trivial and meaningless, I want you to have fun studying this simple sketch of a neuron. Don't be afraid to modify the code, understanding it fully is the goal.
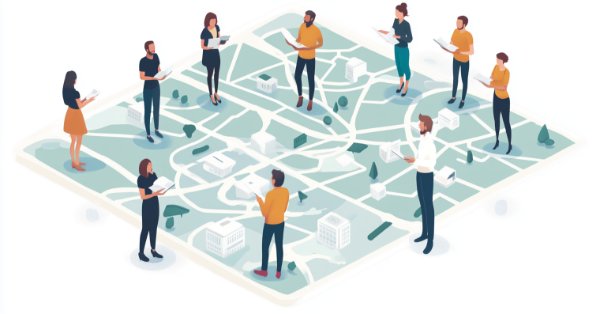
Anarchic Society Optimization (ASO) algorithm
In this article, we will get acquainted with the Anarchic Society Optimization (ASO) algorithm and discuss how an algorithm based on the irrational and adventurous behavior of participants in an anarchic society (an anomalous system of social interaction free from centralized power and various kinds of hierarchies) is able to explore the solution space and avoid the traps of local optimum. The article presents a unified ASO structure applicable to both continuous and discrete problems.
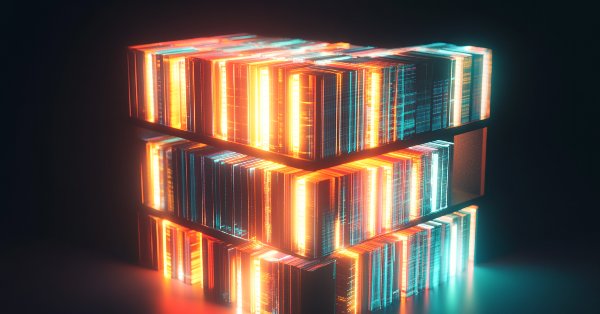
MQL5 Wizard Techniques you should know (Part 55): SAC with Prioritized Experience Replay
Replay buffers in Reinforcement Learning are particularly important with off-policy algorithms like DQN or SAC. This then puts the spotlight on the sampling process of this memory-buffer. While default options with SAC, for instance, use random selection from this buffer, Prioritized Experience Replay buffers fine tune this by sampling from the buffer based on a TD-score. We review the importance of Reinforcement Learning, and, as always, examine just this hypothesis (not the cross-validation) in a wizard assembled Expert Advisor.
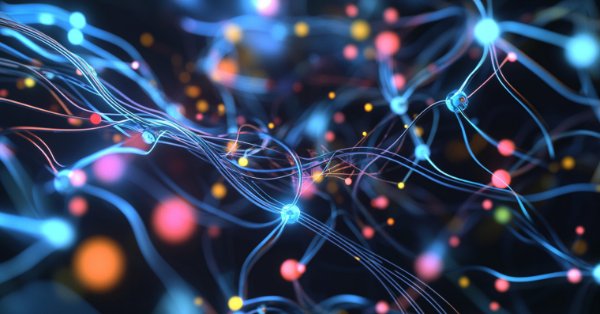
Neural Networks in Trading: Injection of Global Information into Independent Channels (InjectTST)
Most modern multimodal time series forecasting methods use the independent channels approach. This ignores the natural dependence of different channels of the same time series. Smart use of two approaches (independent and mixed channels) is the key to improving the performance of the models.
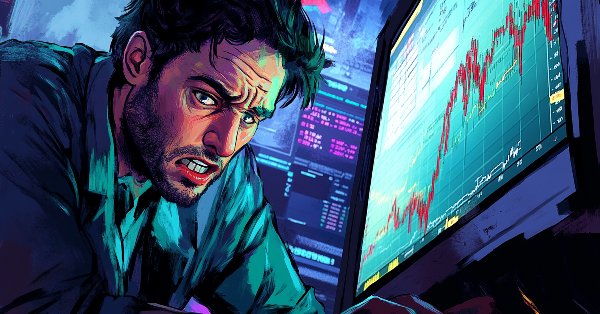
Build Self Optimizing Expert Advisors in MQL5 (Part 6): Stop Out Prevention
Join us in our discussion today as we look for an algorithmic procedure to minimize the total number of times we get stopped out of winning trades. The problem we faced is significantly challenging, and most solutions given in community discussions lack set and fixed rules. Our algorithmic approach to solving the problem increased the profitability of our trades and reduced our average loss per trade. However, there are further advancements to be made to completely filter out all trades that will be stopped out, our solution is a good first step for anyone to try.
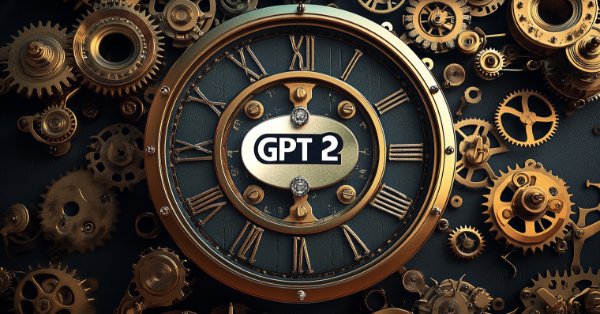
Neural Networks in Trading: Practical Results of the TEMPO Method
We continue our acquaintance with the TEMPO method. In this article we will evaluate the actual effectiveness of the proposed approaches on real historical data.
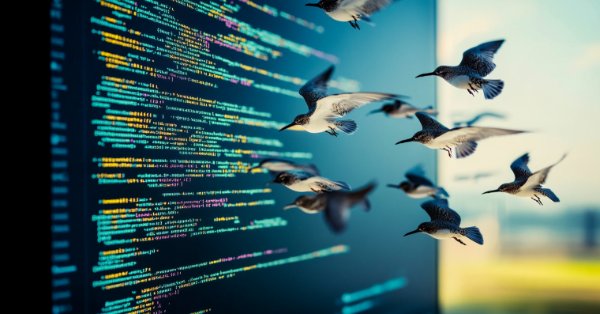
Animal Migration Optimization (AMO) algorithm
The article is devoted to the AMO algorithm, which models the seasonal migration of animals in search of optimal conditions for life and reproduction. The main features of AMO include the use of topological neighborhood and a probabilistic update mechanism, which makes it easy to implement and flexible for various optimization tasks.