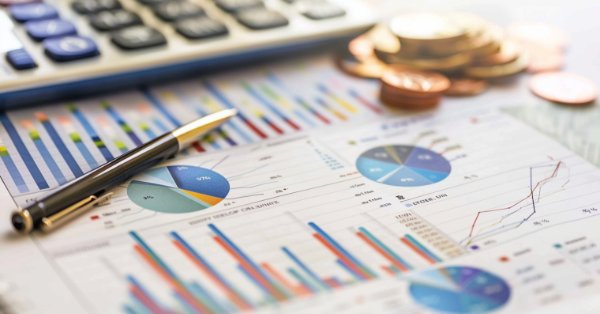
News Trading Made Easy (Part 5): Performing Trades (II)
This article will expand on the trade management class to include buy-stop and sell-stop orders to trade news events and implement an expiration constraint on these orders to prevent any overnight trading. A slippage function will be embedded into the expert to try and prevent or minimize possible slippage that may occur when using stop orders in trading, especially during news events.
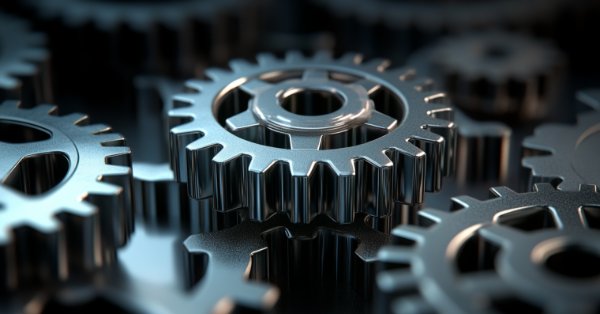
Developing a Replay System (Part 61): Playing the service (II)
In this article, we will look at changes that will allow the replay/simulation system to operate more efficiently and securely. I will also not leave without attention those who want to get the most out of using classes. In addition, we will consider a specific problem in MQL5 that reduces code performance when working with classes, and explain how to solve it.
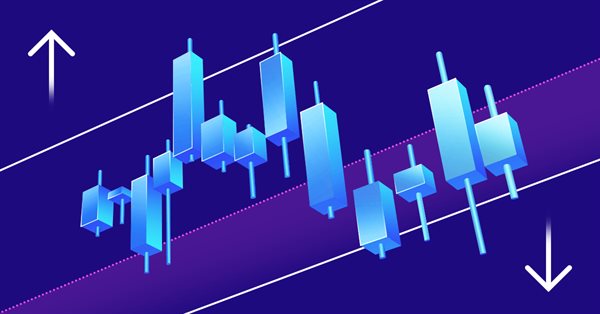
Developing a Replay System — Market simulation (Part 25): Preparing for the next phase
In this article, we complete the first phase of developing our replay and simulation system. Dear reader, with this achievement I confirm that the system has reached an advanced level, paving the way for the introduction of new functionality. The goal is to enrich the system even further, turning it into a powerful tool for research and development of market analysis.
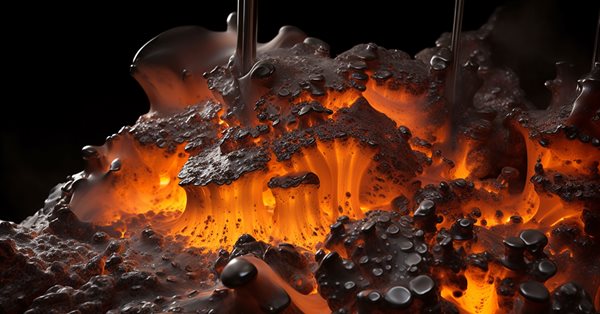
Population optimization algorithms: Simulated Annealing (SA) algorithm. Part I
The Simulated Annealing algorithm is a metaheuristic inspired by the metal annealing process. In the article, we will conduct a thorough analysis of the algorithm and debunk a number of common beliefs and myths surrounding this widely known optimization method. The second part of the article will consider the custom Simulated Isotropic Annealing (SIA) algorithm.
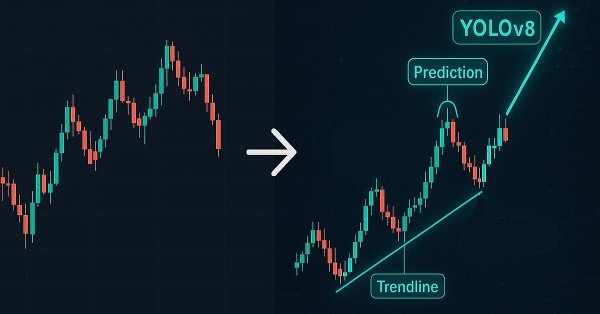
Data Science and ML (Part 41): Forex and Stock Markets Pattern Detection using YOLOv8
Detecting patterns in financial markets is challenging because it involves seeing what's on the chart, something that's difficult to undertake in MQL5 due to image limitations. In this article, we are going to discuss a decent model made in Python that helps us detect patterns present on the chart with minimal effort.
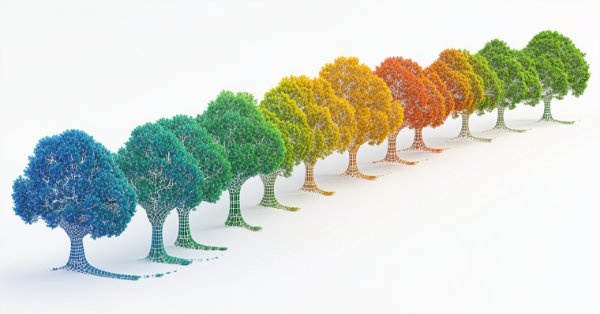
Data Science and ML (Part 31): Using CatBoost AI Models for Trading
CatBoost AI models have gained massive popularity recently among machine learning communities due to their predictive accuracy, efficiency, and robustness to scattered and difficult datasets. In this article, we are going to discuss in detail how to implement these types of models in an attempt to beat the forex market.
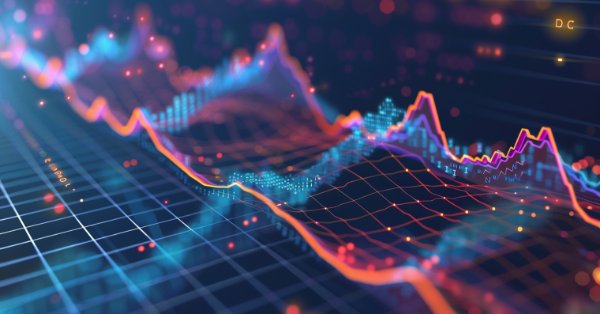
Overcoming ONNX Integration Challenges
ONNX is a great tool for integrating complex AI code between different platforms, it is a great tool that comes with some challenges that one must address to get the most out of it, In this article we discuss the common issues you might face and how to mitigate them.
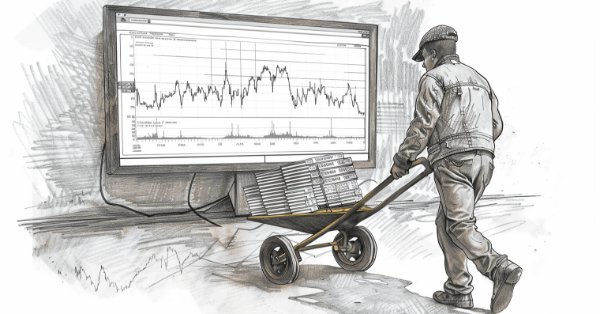
Price Driven CGI Model: Theoretical Foundation
Let's discuss the data manipulation algorithm, as we dive deeper into conceptualizing the idea of using price data to drive CGI objects. Think about transferring the effects of events, human emotions and actions on financial asset prices to a real-life model. This study delves into leveraging price data to influence the scale of a CGI object, controlling growth and emotions. These visible effects can establish a fresh analytical foundation for traders. Further insights are shared in the article.
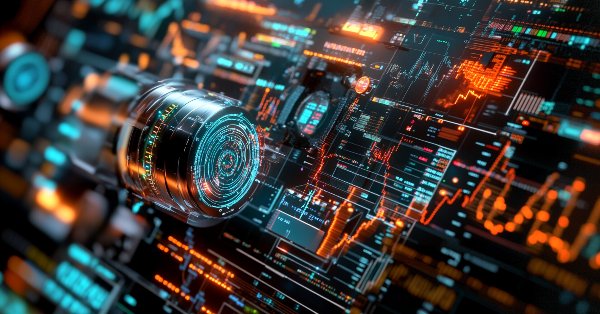
Day Trading Larry Connors RSI2 Mean-Reversion Strategies
Larry Connors is a renowned trader and author, best known for his work in quantitative trading and strategies like the 2-period RSI (RSI2), which helps identify short-term overbought and oversold market conditions. In this article, we’ll first explain the motivation behind our research, then recreate three of Connors’ most famous strategies in MQL5 and apply them to intraday trading of the S&P 500 index CFD.
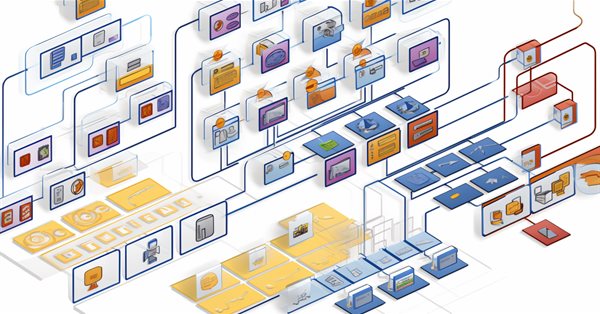
Developing a Replay System (Part 36): Making Adjustments (II)
One of the things that can make our lives as programmers difficult is assumptions. In this article, I will show you how dangerous it is to make assumptions: both in MQL5 programming, where you assume that the type will have a certain value, and in MetaTrader 5, where you assume that different servers work the same.
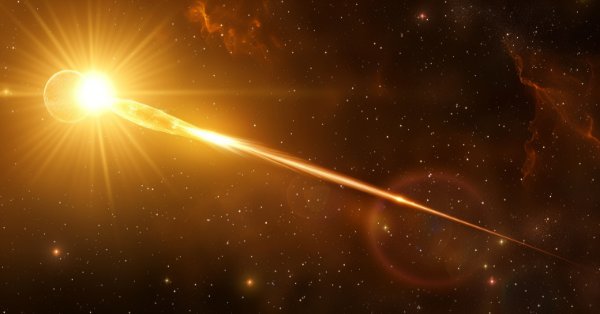
Comet Tail Algorithm (CTA)
In this article, we will look at the Comet Tail Optimization Algorithm (CTA), which draws inspiration from unique space objects - comets and their impressive tails that form when approaching the Sun. The algorithm is based on the concept of the motion of comets and their tails, and is designed to find optimal solutions in optimization problems.
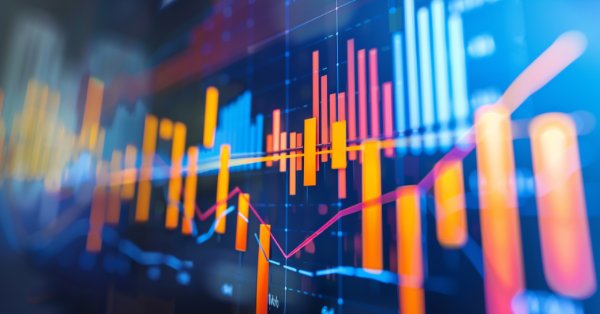
MQL5 Wizard Techniques you should know (Part 26): Moving Averages and the Hurst Exponent
The Hurst Exponent is a measure of how much a time series auto-correlates over the long term. It is understood to be capturing the long-term properties of a time series and therefore carries some weight in time series analysis even outside of economic/ financial time series. We however, focus on its potential benefit to traders by examining how this metric could be paired with moving averages to build a potentially robust signal.
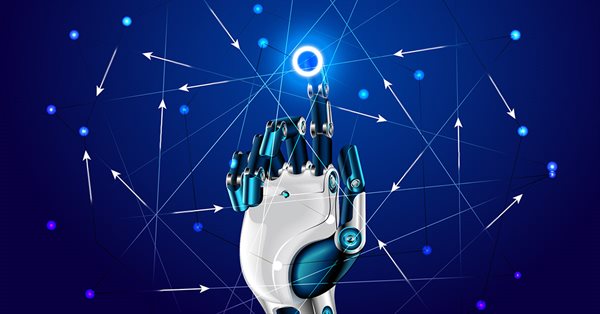
Developing a quality factor for Expert Advisors
In this article, we will see how to develop a quality score that your Expert Advisor can display in the strategy tester. We will look at two well-known calculation methods – Van Tharp and Sunny Harris.
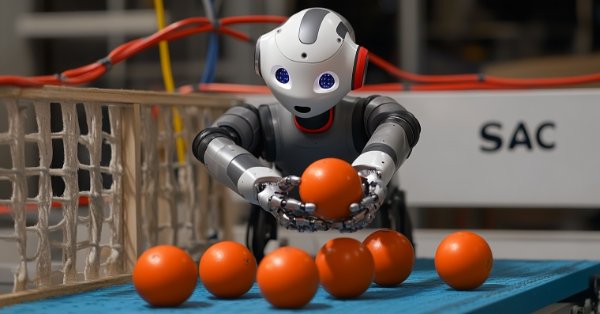
MQL5 Wizard Techniques you should know (Part 51): Reinforcement Learning with SAC
Soft Actor Critic is a Reinforcement Learning algorithm that utilizes 3 neural networks. An actor network and 2 critic networks. These machine learning models are paired in a master slave partnership where the critics are modelled to improve the forecast accuracy of the actor network. While also introducing ONNX in these series, we explore how these ideas could be put to test as a custom signal of a wizard assembled Expert Advisor.
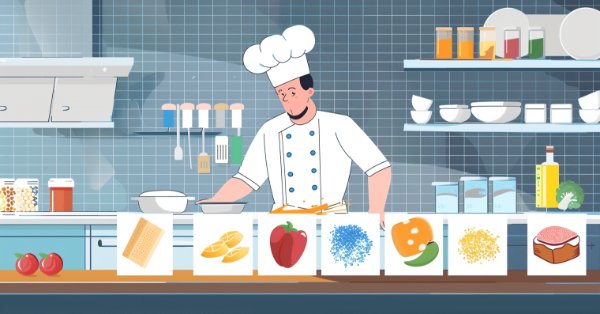
The base class of population algorithms as the backbone of efficient optimization
The article represents a unique research attempt to combine a variety of population algorithms into a single class to simplify the application of optimization methods. This approach not only opens up opportunities for the development of new algorithms, including hybrid variants, but also creates a universal basic test stand. This stand becomes a key tool for choosing the optimal algorithm depending on a specific task.
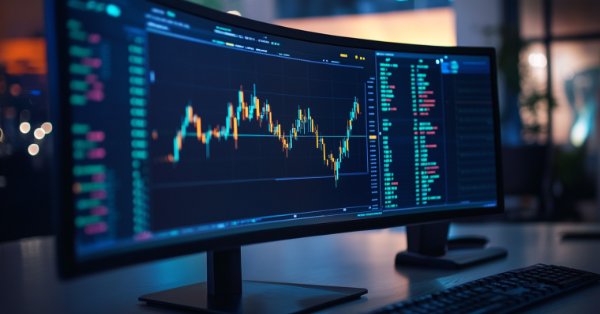
Developing a Replay System (Part 70): Getting the Time Right (III)
In this article, we will look at how to use the CustomBookAdd function correctly and effectively. Despite its apparent simplicity, it has many nuances. For example, it allows you to tell the mouse indicator whether a custom symbol is on auction, being traded, or the market is closed. The content presented here is intended solely for educational purposes. Under no circumstances should the application be viewed for any purpose other than to learn and master the concepts presented.
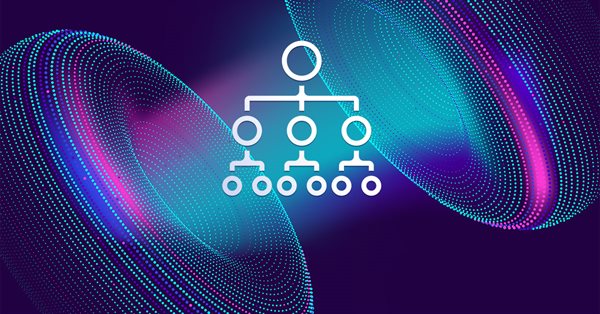
Neural networks made easy (Part 41): Hierarchical models
The article describes hierarchical training models that offer an effective approach to solving complex machine learning problems. Hierarchical models consist of several levels, each of which is responsible for different aspects of the task.
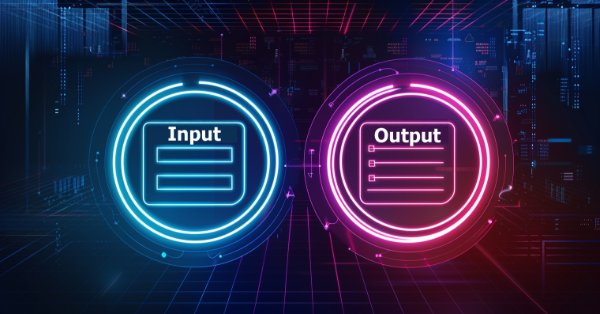
Developing a Replay System (Part 44): Chart Trade Project (III)
In the previous article I explained how you can manipulate template data for use in OBJ_CHART. In that article, I only outlined the topic without going into details, since in that version the work was done in a very simplified way. This was done to make it easier to explain the content, because despite the apparent simplicity of many things, some of them were not so obvious, and without understanding the simplest and most basic part, you would not be able to truly understand the entire picture.
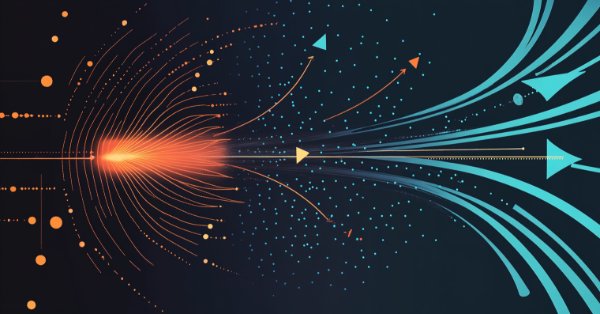
MQL5 Wizard Techniques you should know (Part 31): Selecting the Loss Function
Loss Function is the key metric of machine learning algorithms that provides feedback to the training process by quantifying how well a given set of parameters are performing when compared to their intended target. We explore the various formats of this function in an MQL5 custom wizard class.
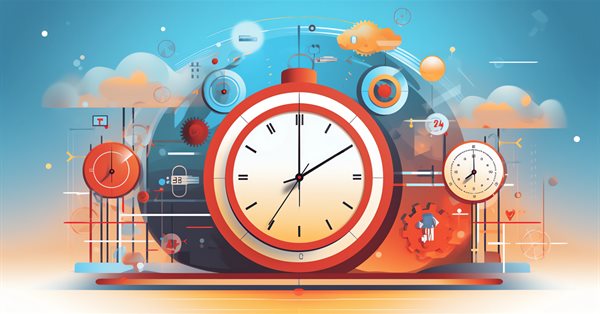
Developing a Replay System (Part 31): Expert Advisor project — C_Mouse class (V)
We need a timer that can show how much time is left till the end of the replay/simulation run. This may seem at first glance to be a simple and quick solution. Many simply try to adapt and use the same system that the trading server uses. But there's one thing that many people don't consider when thinking about this solution: with replay, and even m ore with simulation, the clock works differently. All this complicates the creation of such a system.
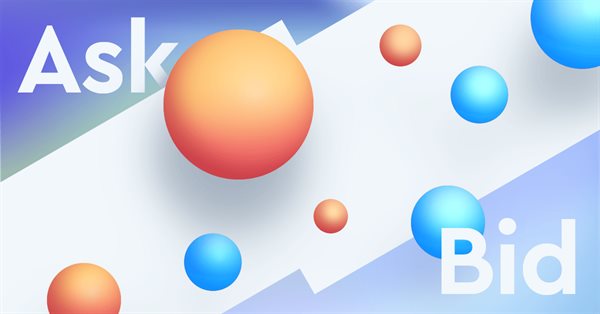
Developing a Replay System — Market simulation (Part 18): Ticks and more ticks (II)
Obviously the current metrics are very far from the ideal time for creating a 1-minute bar. That's the first thing we are going to fix. Fixing the synchronization problem is not difficult. This may seem hard, but it's actually quite simple. We did not make the required correction in the previous article since its purpose was to explain how to transfer the tick data that was used to create the 1-minute bars on the chart into the Market Watch window.
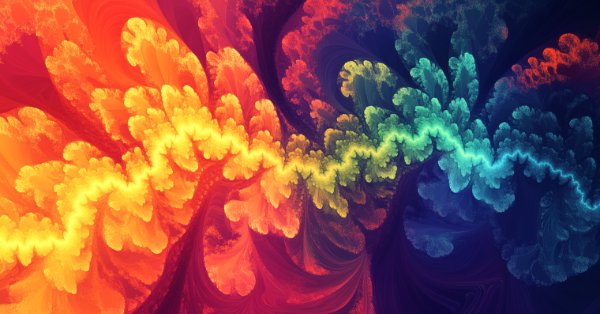
Chaos theory in trading (Part 2): Diving deeper
We continue our dive into chaos theory in financial markets. This time I will consider its applicability to the analysis of currencies and other assets.
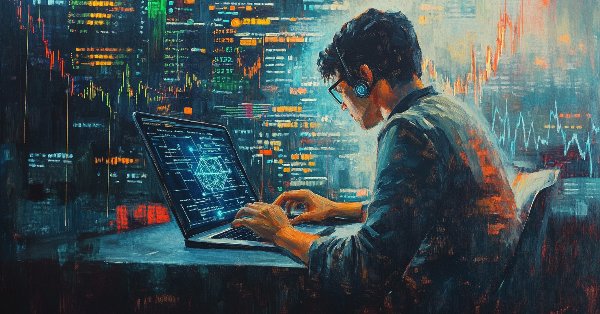
Data Science and ML (Part 38): AI Transfer Learning in Forex Markets
The AI breakthroughs dominating headlines, from ChatGPT to self-driving cars, aren’t built from isolated models but through cumulative knowledge transferred from various models or common fields. Now, this same "learn once, apply everywhere" approach can be applied to help us transform our AI models in algorithmic trading. In this article, we are going to learn how we can leverage the information gained across various instruments to help in improving predictions on others using transfer learning.
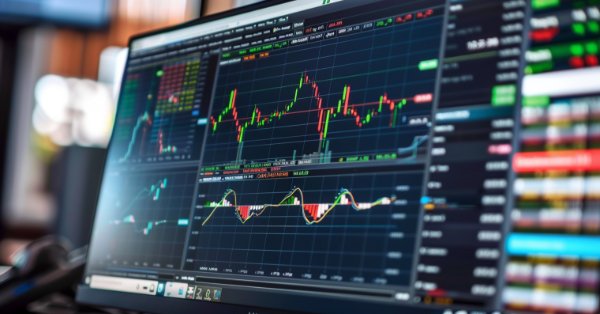
Developing a Replay System (Part 49): Things Get Complicated (I)
In this article, we'll complicate things a little. Using what was shown in the previous articles, we will start to open up the template file so that the user can use their own template. However, I will be making changes gradually, as I will also be refining the indicator to reduce the load on MetaTrader 5.
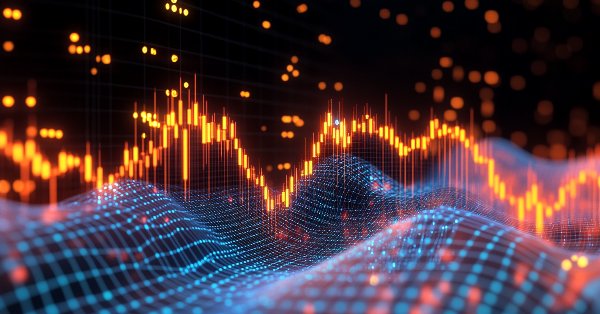
Price Action Analysis Toolkit Development (Part 24): Price Action Quantification Analysis Tool
Candlestick patterns offer valuable insights into potential market moves. Some single candles signal continuation of the current trend, while others foreshadow reversals, depending on their position within the price action. This article introduces an EA that automatically identifies four key candlestick formations. Explore the following sections to learn how this tool can enhance your price-action analysis.
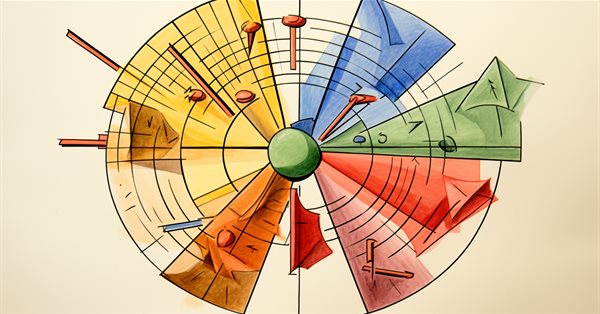
Implementation of the Augmented Dickey Fuller test in MQL5
In this article we demonstrate the implementation of the Augmented Dickey-Fuller test, and apply it to conduct cointegration tests using the Engle-Granger method.
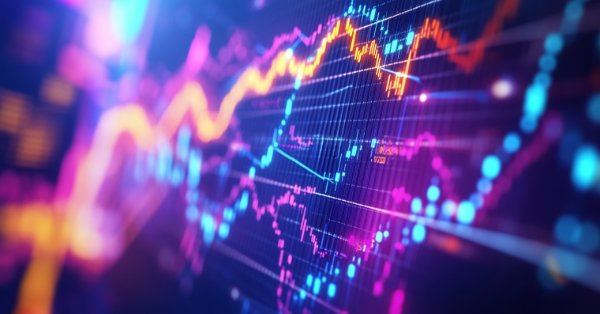
Example of Stochastic Optimization and Optimal Control
This Expert Advisor, named SMOC (likely standing for Stochastic Model Optimal Control), is a simple example of an advanced algorithmic trading system for MetaTrader 5. It uses a combination of technical indicators, model predictive control, and dynamic risk management to make trading decisions. The EA incorporates adaptive parameters, volatility-based position sizing, and trend analysis to optimize its performance across varying market conditions.
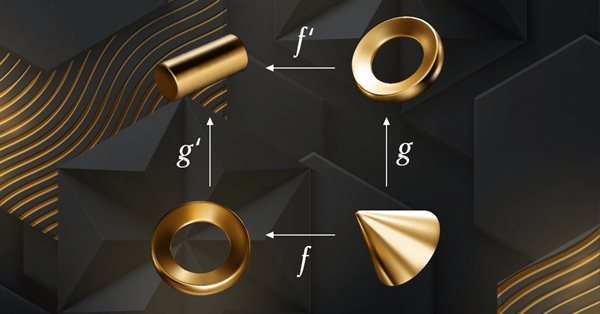
Category Theory in MQL5 (Part 6): Monomorphic Pull-Backs and Epimorphic Push-Outs
Category Theory is a diverse and expanding branch of Mathematics which is only recently getting some coverage in the MQL5 community. These series of articles look to explore and examine some of its concepts & axioms with the overall goal of establishing an open library that provides insight while also hopefully furthering the use of this remarkable field in Traders' strategy development.
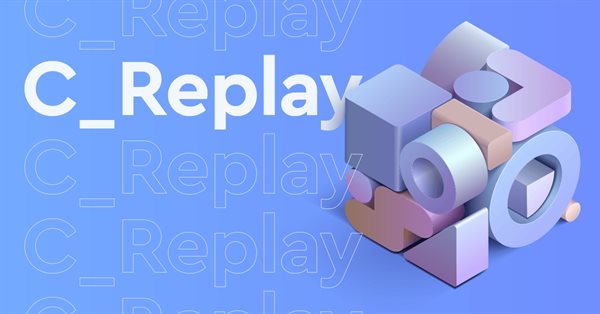
Developing a Replay System — Market simulation (Part 16): New class system
We need to organize our work better. The code is growing, and if this is not done now, then it will become impossible. Let's divide and conquer. MQL5 allows the use of classes which will assist in implementing this task, but for this we need to have some knowledge about classes. Probably the thing that confuses beginners the most is inheritance. In this article, we will look at how to use these mechanisms in a practical and simple way.
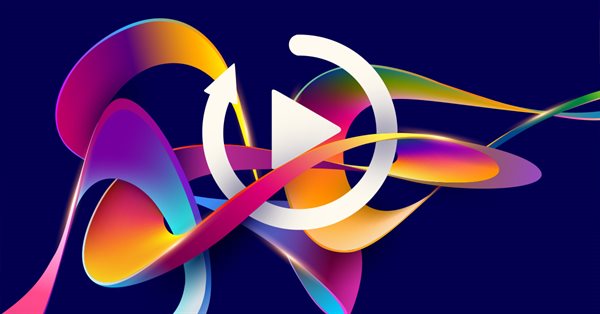
Developing a Replay System — Market simulation (Part 07): First improvements (II)
In the previous article, we made some fixes and added tests to our replication system to ensure the best possible stability. We also started creating and using a configuration file for this system.
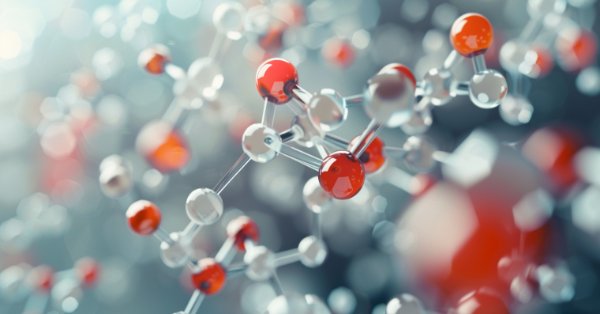
Chemical reaction optimization (CRO) algorithm (Part II): Assembling and results
In the second part, we will collect chemical operators into a single algorithm and present a detailed analysis of its results. Let's find out how the Chemical reaction optimization (CRO) method copes with solving complex problems on test functions.
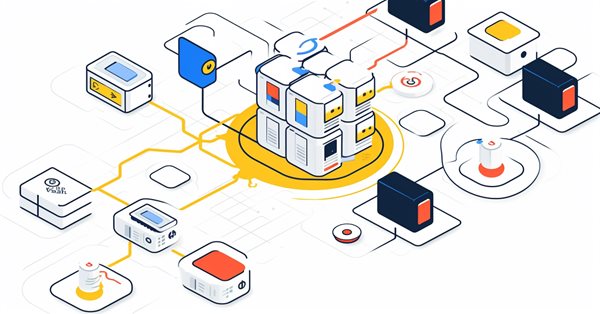
Data label for time series mining (Part 6):Apply and Test in EA Using ONNX
This series of articles introduces several time series labeling methods, which can create data that meets most artificial intelligence models, and targeted data labeling according to needs can make the trained artificial intelligence model more in line with the expected design, improve the accuracy of our model, and even help the model make a qualitative leap!
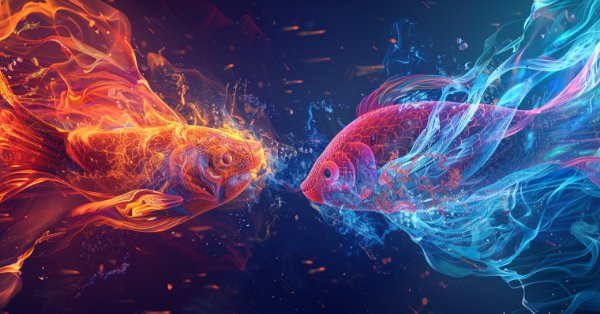
Artificial Cooperative Search (ACS) algorithm
Artificial Cooperative Search (ACS) is an innovative method using a binary matrix and multiple dynamic populations based on mutualistic relationships and cooperation to find optimal solutions quickly and accurately. ACS unique approach to predators and prey enables it to achieve excellent results in numerical optimization problems.
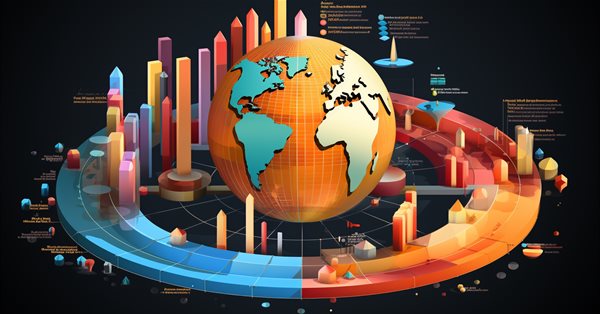
The case for using a Composite Data Set this Q4 in weighing SPDR XLY's next performance
We consider XLY, SPDR’s consumer discretionary spending ETF and see if with tools in MetaTrader’s IDE we can sift through an array of data sets in selecting what could work with a forecasting model with a forward outlook of not more than a year.
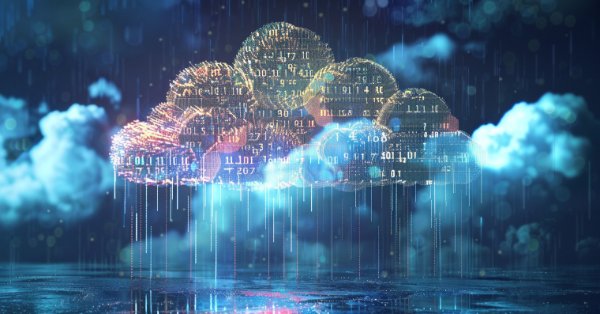
Time series clustering in causal inference
Clustering algorithms in machine learning are important unsupervised learning algorithms that can divide the original data into groups with similar observations. By using these groups, you can analyze the market for a specific cluster, search for the most stable clusters using new data, and make causal inferences. The article proposes an original method for time series clustering in Python.
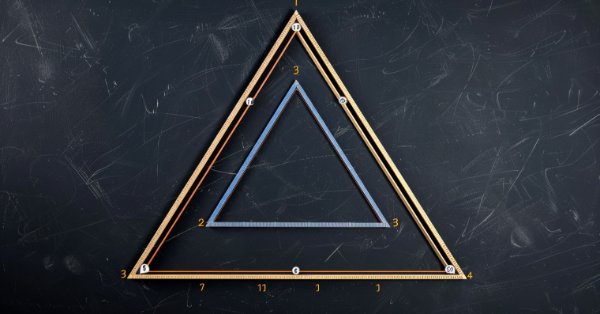
MQL5 Wizard Techniques you should know (Part 27): Moving Averages and the Angle of Attack
The Angle of Attack is an often-quoted metric whose steepness is understood to strongly correlate with the strength of a prevailing trend. We look at how it is commonly used and understood and examine if there are changes that could be introduced in how it's measured for the benefit of a trade system that puts it in use.
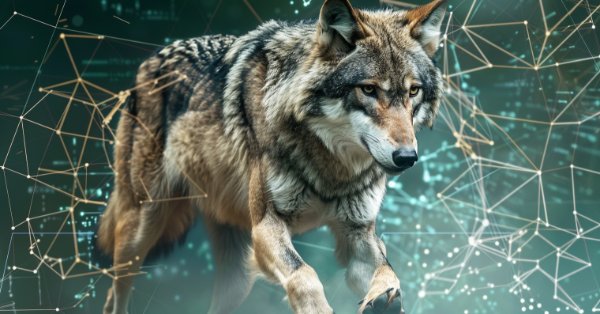
Population optimization algorithms: Resistance to getting stuck in local extrema (Part II)
We continue our experiment that aims to examine the behavior of population optimization algorithms in the context of their ability to efficiently escape local minima when population diversity is low and reach global maxima. Research results are provided.
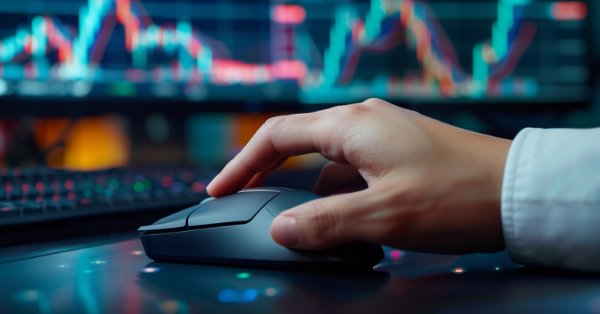
Developing a Replay System (Part 47): Chart Trade Project (VI)
Finally, our Chart Trade indicator starts interacting with the EA, allowing information to be transferred interactively. Therefore, in this article, we will improve the indicator, making it functional enough to be used together with any EA. This will allow us to access the Chart Trade indicator and work with it as if it were actually connected with an EA. But we will do it in a much more interesting way than before.
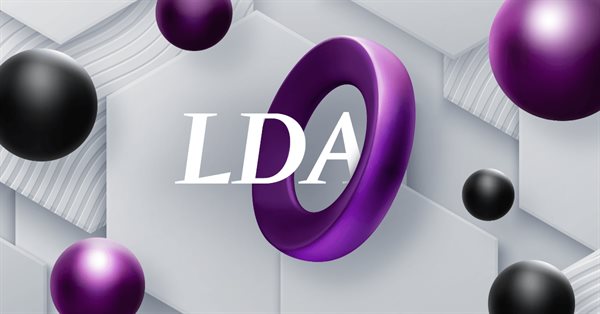
Category Theory in MQL5 (Part 21): Natural Transformations with LDA
This article, the 21st in our series, continues with a look at Natural Transformations and how they can be implemented using linear discriminant analysis. We present applications of this in a signal class format, like in the previous article.
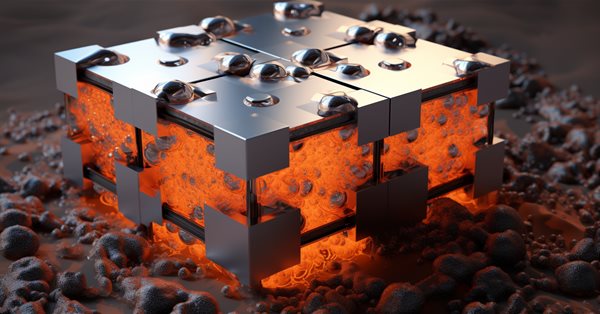
Population optimization algorithms: Simulated Isotropic Annealing (SIA) algorithm. Part II
The first part was devoted to the well-known and popular algorithm - simulated annealing. We have thoroughly considered its pros and cons. The second part of the article is devoted to the radical transformation of the algorithm, which turns it into a new optimization algorithm - Simulated Isotropic Annealing (SIA).