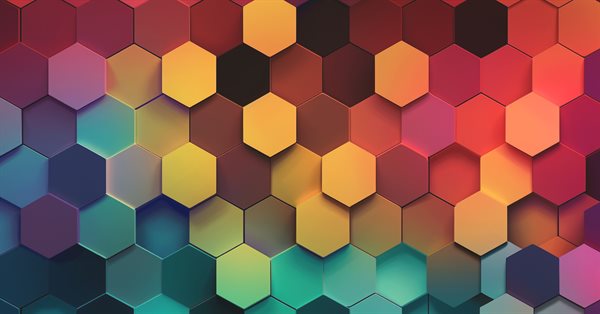
Data Science and Machine Learning(Part 14): Finding Your Way in the Markets with Kohonen Maps
Are you looking for a cutting-edge approach to trading that can help you navigate complex and ever-changing markets? Look no further than Kohonen maps, an innovative form of artificial neural networks that can help you uncover hidden patterns and trends in market data. In this article, we'll explore how Kohonen maps work, and how they can be used to develop smarter, more effective trading strategies. Whether you're a seasoned trader or just starting out, you won't want to miss this exciting new approach to trading.
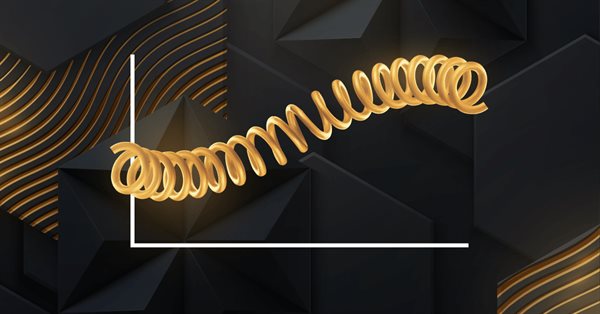
Category Theory in MQL5 (Part 3)
Category Theory is a diverse and expanding branch of Mathematics which as of yet is relatively uncovered in the MQL5 community. These series of articles look to introduce and examine some of its concepts with the overall goal of establishing an open library that provides insight while hopefully furthering the use of this remarkable field in Traders' strategy development.
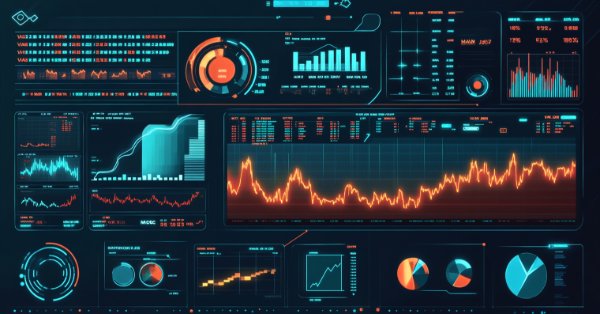
Example of new Indicator and Conditional LSTM
This article explores the development of an Expert Advisor (EA) for automated trading that combines technical analysis with deep learning predictions.
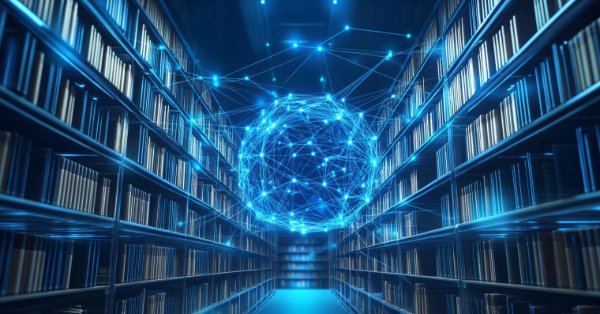
Integrate Your Own LLM into EA (Part 5): Develop and Test Trading Strategy with LLMs (III) – Adapter-Tuning
With the rapid development of artificial intelligence today, language models (LLMs) are an important part of artificial intelligence, so we should think about how to integrate powerful LLMs into our algorithmic trading. For most people, it is difficult to fine-tune these powerful models according to their needs, deploy them locally, and then apply them to algorithmic trading. This series of articles will take a step-by-step approach to achieve this goal.
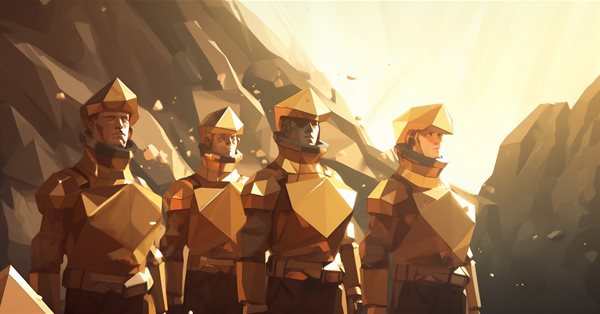
Population optimization algorithms: Stochastic Diffusion Search (SDS)
The article discusses Stochastic Diffusion Search (SDS), which is a very powerful and efficient optimization algorithm based on the principles of random walk. The algorithm allows finding optimal solutions in complex multidimensional spaces, while featuring a high speed of convergence and the ability to avoid local extrema.
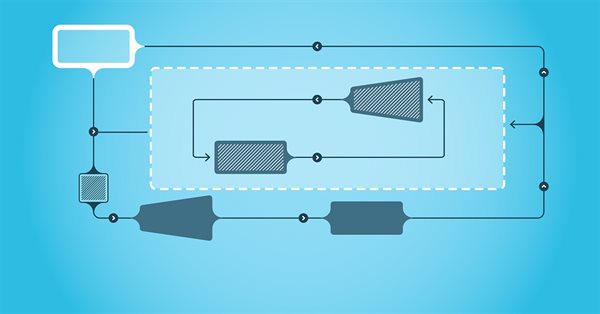
Neural networks made easy (Part 44): Learning skills with dynamics in mind
In the previous article, we introduced the DIAYN method, which offers the algorithm for learning a variety of skills. The acquired skills can be used for various tasks. But such skills can be quite unpredictable, which can make them difficult to use. In this article, we will look at an algorithm for learning predictable skills.
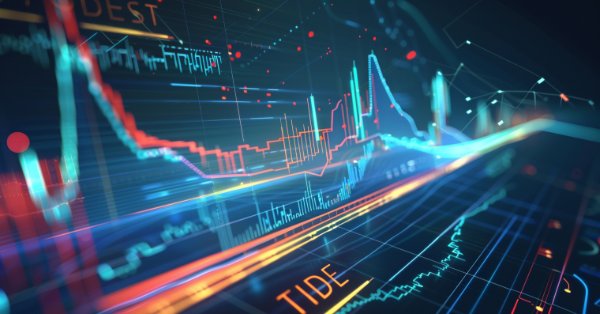
Neural Networks Made Easy (Part 88): Time-Series Dense Encoder (TiDE)
In an attempt to obtain the most accurate forecasts, researchers often complicate forecasting models. Which in turn leads to increased model training and maintenance costs. Is such an increase always justified? This article introduces an algorithm that uses the simplicity and speed of linear models and demonstrates results on par with the best models with a more complex architecture.
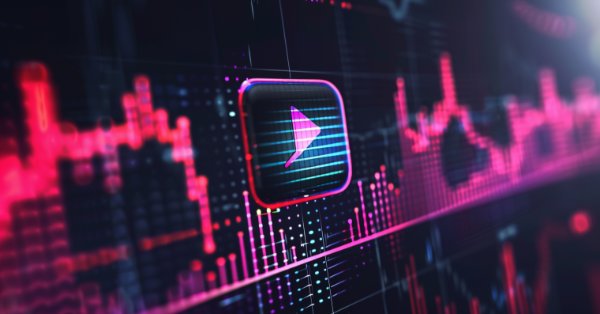
Neural Networks Made Easy (Part 94): Optimizing the Input Sequence
When working with time series, we always use the source data in their historical sequence. But is this the best option? There is an opinion that changing the sequence of the input data will improve the efficiency of the trained models. In this article I invite you to get acquainted with one of the methods for optimizing the input sequence.
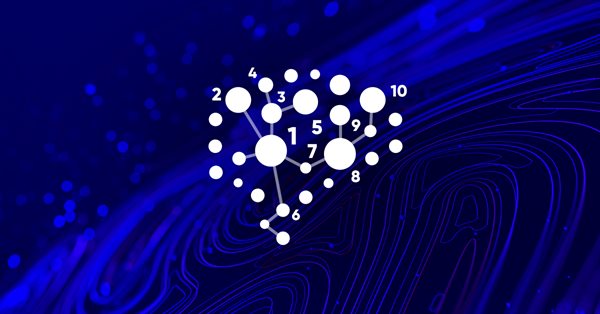
Experiments with neural networks (Part 4): Templates
In this article, I will use experimentation and non-standard approaches to develop a profitable trading system and check whether neural networks can be of any help for traders. MetaTrader 5 as a self-sufficient tool for using neural networks in trading. Simple explanation.
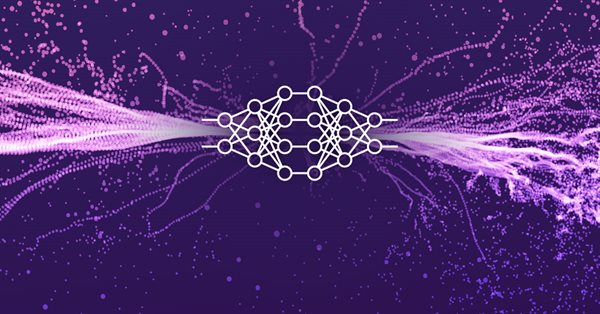
Neural networks made easy (Part 50): Soft Actor-Critic (model optimization)
In the previous article, we implemented the Soft Actor-Critic algorithm, but were unable to train a profitable model. Here we will optimize the previously created model to obtain the desired results.
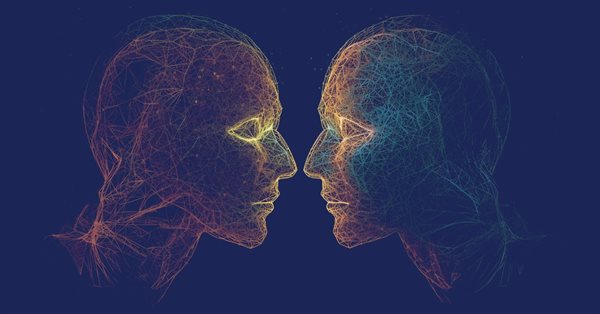
Neural networks made easy (Part 38): Self-Supervised Exploration via Disagreement
One of the key problems within reinforcement learning is environmental exploration. Previously, we have already seen the research method based on Intrinsic Curiosity. Today I propose to look at another algorithm: Exploration via Disagreement.
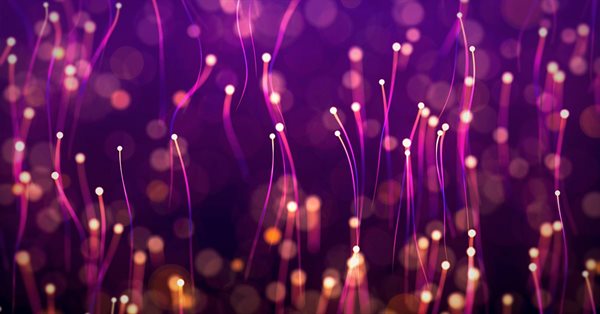
Population optimization algorithms: Firefly Algorithm (FA)
In this article, I will consider the Firefly Algorithm (FA) optimization method. Thanks to the modification, the algorithm has turned from an outsider into a real rating table leader.
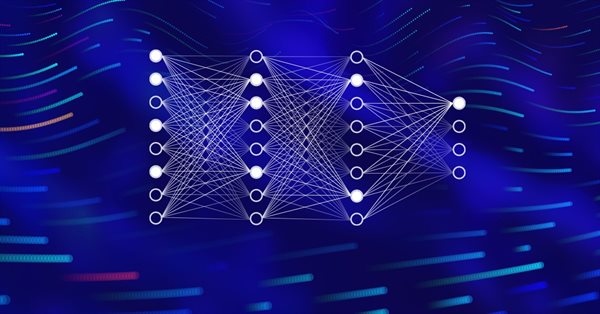
Neural networks made easy (Part 43): Mastering skills without the reward function
The problem of reinforcement learning lies in the need to define a reward function. It can be complex or difficult to formalize. To address this problem, activity-based and environment-based approaches are being explored to learn skills without an explicit reward function.
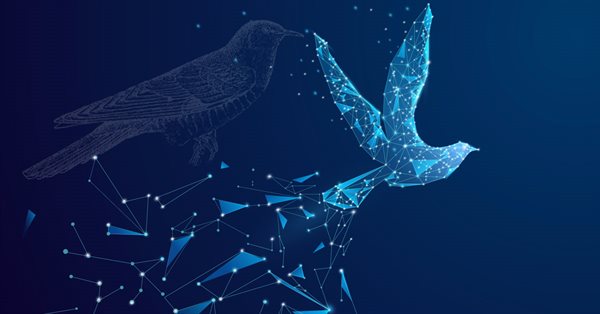
Population optimization algorithms: Cuckoo Optimization Algorithm (COA)
The next algorithm I will consider is cuckoo search optimization using Levy flights. This is one of the latest optimization algorithms and a new leader in the leaderboard.
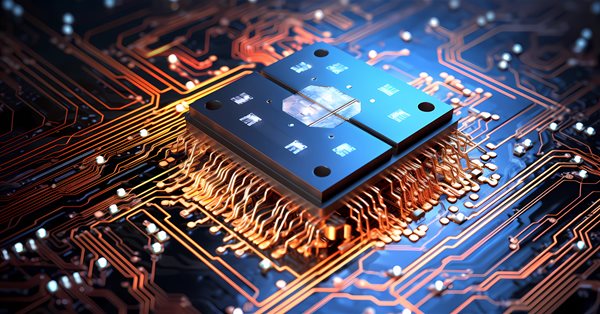
Integrate Your Own LLM into EA (Part 1): Hardware and Environment Deployment
With the rapid development of artificial intelligence today, language models (LLMs) are an important part of artificial intelligence, so we should think about how to integrate powerful LLMs into our algorithmic trading. For most people, it is difficult to fine-tune these powerful models according to their needs, deploy them locally, and then apply them to algorithmic trading. This series of articles will take a step-by-step approach to achieve this goal.
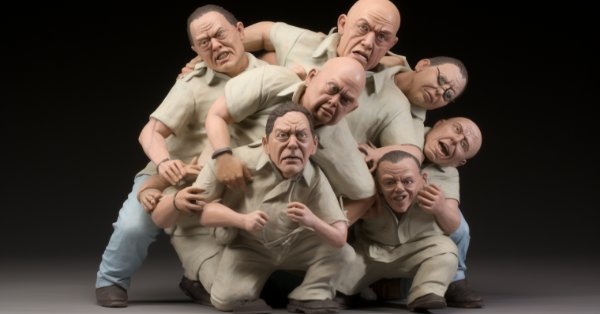
Data Science and Machine Learning (Part 19): Supercharge Your AI models with AdaBoost
AdaBoost, a powerful boosting algorithm designed to elevate the performance of your AI models. AdaBoost, short for Adaptive Boosting, is a sophisticated ensemble learning technique that seamlessly integrates weak learners, enhancing their collective predictive strength.
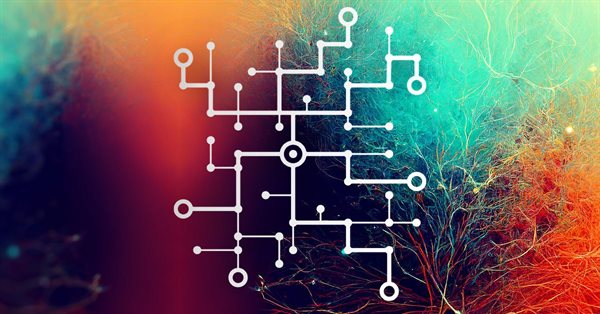
Neural networks made easy (Part 35): Intrinsic Curiosity Module
We continue to study reinforcement learning algorithms. All the algorithms we have considered so far required the creation of a reward policy to enable the agent to evaluate each of its actions at each transition from one system state to another. However, this approach is rather artificial. In practice, there is some time lag between an action and a reward. In this article, we will get acquainted with a model training algorithm which can work with various time delays from the action to the reward.
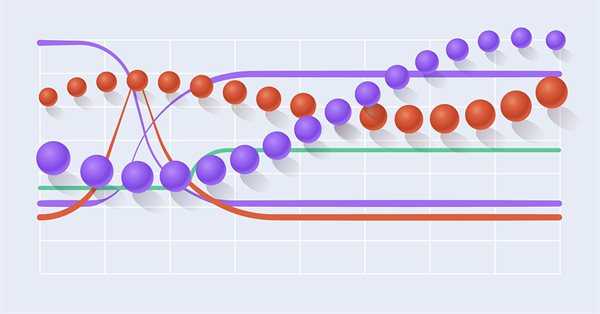
Integrating ML models with the Strategy Tester (Part 3): Managing CSV files (II)
This material provides a complete guide to creating a class in MQL5 for efficient management of CSV files. We will see the implementation of methods for opening, writing, reading, and transforming data. We will also consider how to use them to store and access information. In addition, we will discuss the limitations and the most important aspects of using such a class. This article ca be a valuable resource for those who want to learn how to process CSV files in MQL5.
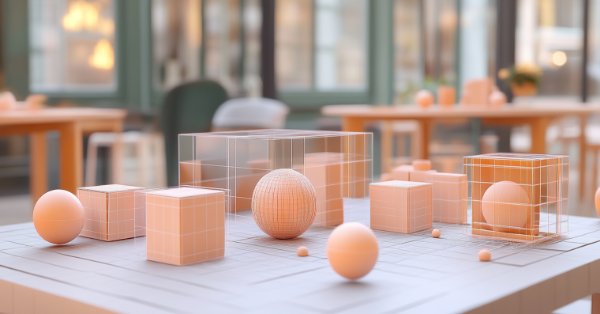
Neural Networks in Trading: Scene-Aware Object Detection (HyperDet3D)
We invite you to get acquainted with a new approach to detecting objects using hypernetworks. A hypernetwork generates weights for the main model, which allows taking into account the specifics of the current market situation. This approach allows us to improve forecasting accuracy by adapting the model to different trading conditions.
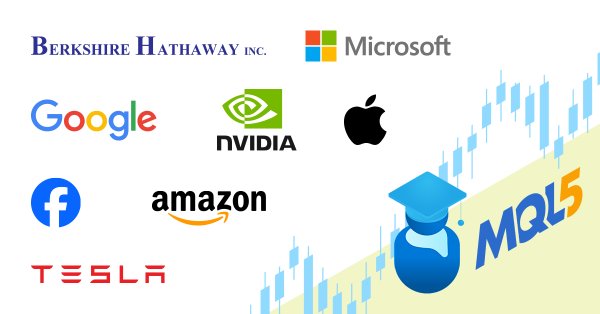
SP500 Trading Strategy in MQL5 For Beginners
Discover how to leverage MQL5 to forecast the S&P 500 with precision, blending in classical technical analysis for added stability and combining algorithms with time-tested principles for robust market insights.
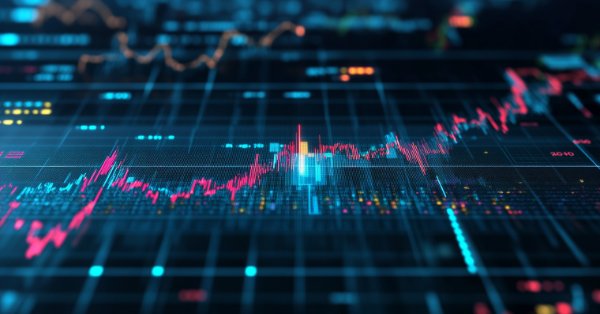
Data Science and ML(Part 30): The Power Couple for Predicting the Stock Market, Convolutional Neural Networks(CNNs) and Recurrent Neural Networks(RNNs)
In this article, We explore the dynamic integration of Convolutional Neural Networks (CNNs) and Recurrent Neural Networks (RNNs) in stock market prediction. By leveraging CNNs' ability to extract patterns and RNNs' proficiency in handling sequential data. Let us see how this powerful combination can enhance the accuracy and efficiency of trading algorithms.
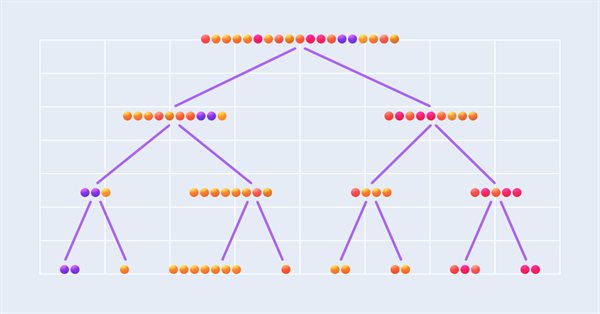
Integrating ML models with the Strategy Tester (Conclusion): Implementing a regression model for price prediction
This article describes the implementation of a regression model based on a decision tree. The model should predict prices of financial assets. We have already prepared the data, trained and evaluated the model, as well as adjusted and optimized it. However, it is important to note that this model is intended for study purposes only and should not be used in real trading.
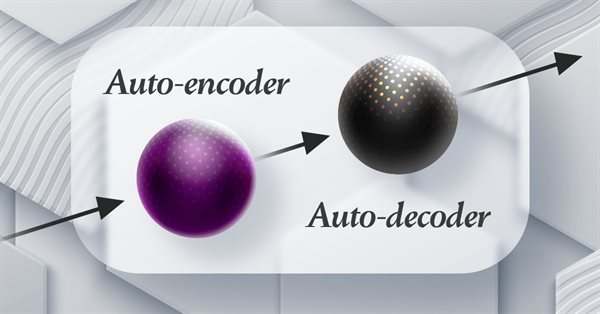
Category Theory in MQL5 (Part 20): A detour to Self-Attention and the Transformer
We digress in our series by pondering at part of the algorithm to chatGPT. Are there any similarities or concepts borrowed from natural transformations? We attempt to answer these and other questions in a fun piece, with our code in a signal class format.
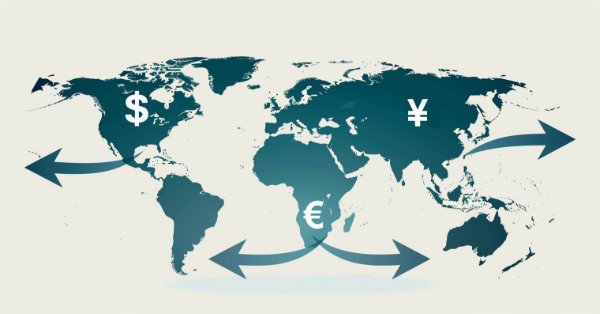
Triangular arbitrage with predictions
This article simplifies triangular arbitrage, showing you how to use predictions and specialized software to trade currencies smarter, even if you're new to the market. Ready to trade with expertise?
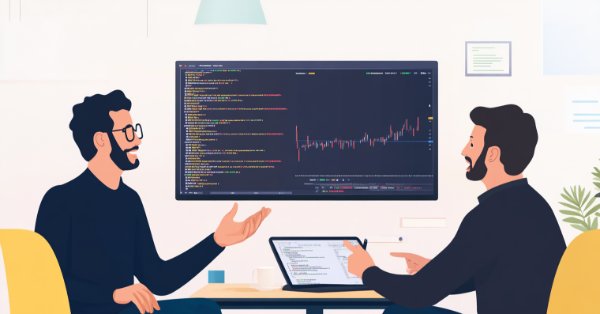
Build Self Optimizing Expert Advisors With MQL5 And Python (Part II): Tuning Deep Neural Networks
Machine learning models come with various adjustable parameters. In this series of articles, we will explore how to customize your AI models to fit your specific market using the SciPy library.
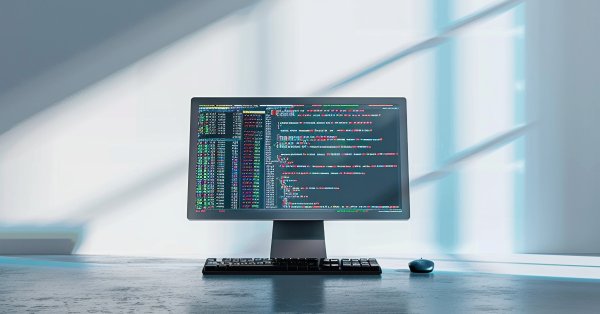
Developing a robot in Python and MQL5 (Part 2): Model selection, creation and training, Python custom tester
We continue the series of articles on developing a trading robot in Python and MQL5. Today we will solve the problem of selecting and training a model, testing it, implementing cross-validation, grid search, as well as the problem of model ensemble.
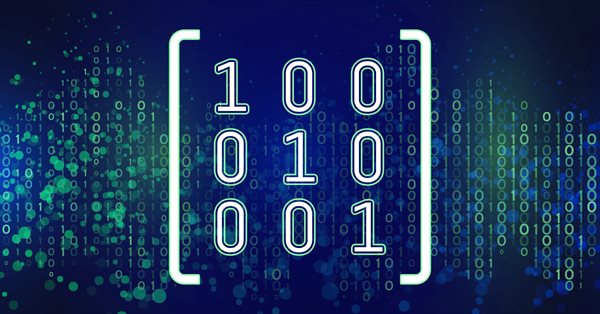
Matrix Utils, Extending the Matrices and Vector Standard Library Functionality
Matrix serves as the foundation of machine learning algorithms and computers in general because of their ability to effectively handle large mathematical operations, The Standard library has everything one needs but let's see how we can extend it by introducing several functions in the utils file, that are not yet available in the library
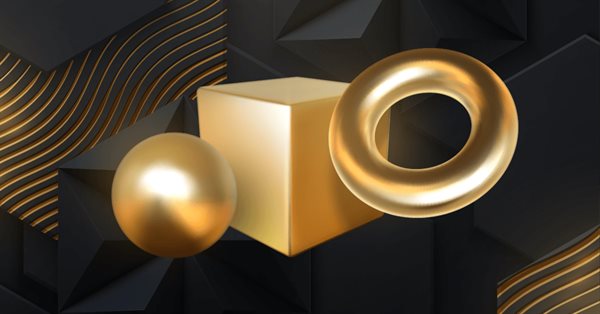
Category Theory in MQL5 (Part 2)
Category Theory is a diverse and expanding branch of Mathematics which as of yet is relatively uncovered in the MQL5 community. These series of articles look to introduce and examine some of its concepts with the overall goal of establishing an open library that attracts comments and discussion while hopefully furthering the use of this remarkable field in Traders' strategy development.
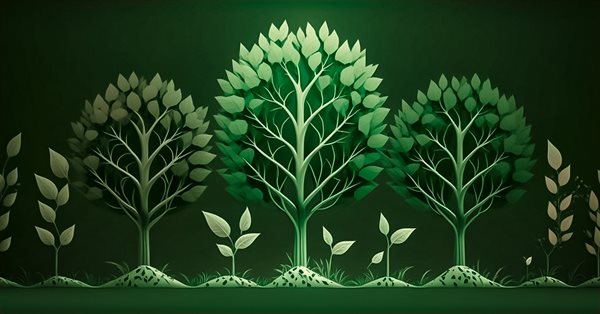
Population optimization algorithms: Saplings Sowing and Growing up (SSG)
Saplings Sowing and Growing up (SSG) algorithm is inspired by one of the most resilient organisms on the planet demonstrating outstanding capability for survival in a wide variety of conditions.
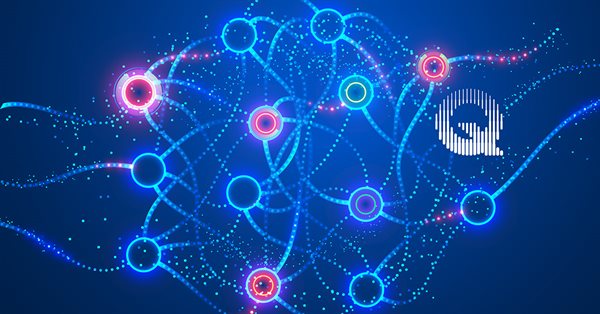
Neural networks made easy (Part 48): Methods for reducing overestimation of Q-function values
In the previous article, we introduced the DDPG method, which allows training models in a continuous action space. However, like other Q-learning methods, DDPG is prone to overestimating Q-function values. This problem often results in training an agent with a suboptimal strategy. In this article, we will look at some approaches to overcome the mentioned issue.
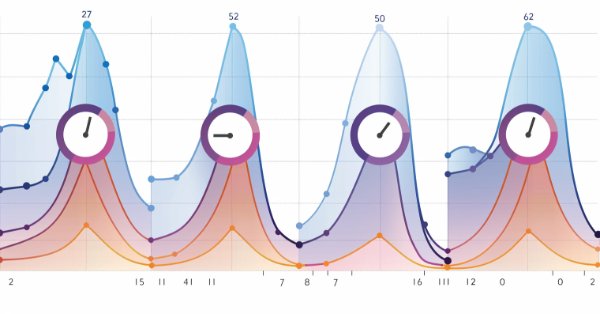
Seasonality Filtering and time period for Deep Learning ONNX models with python for EA
Can we benefit from seasonality when creating models for Deep Learning with Python? Does filtering data for the ONNX models help to get better results? What time period should we use? We will cover all of this over this article.
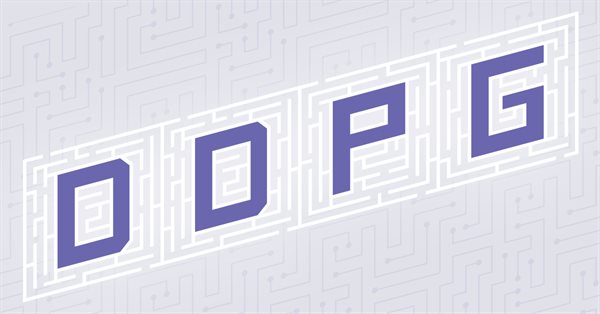
Neural networks made easy (Part 47): Continuous action space
In this article, we expand the range of tasks of our agent. The training process will include some aspects of money and risk management, which are an integral part of any trading strategy.
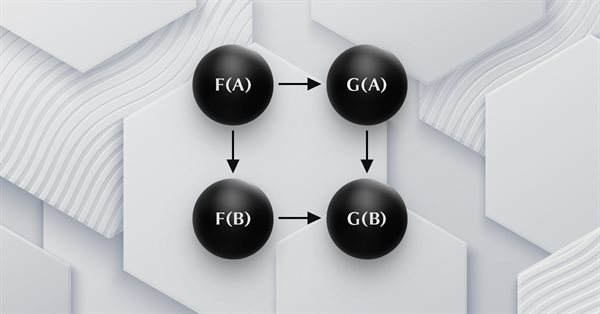
Category Theory in MQL5 (Part 18): Naturality Square
This article continues our series into category theory by introducing natural transformations, a key pillar within the subject. We look at the seemingly complex definition, then delve into examples and applications with this series’ ‘bread and butter’; volatility forecasting.
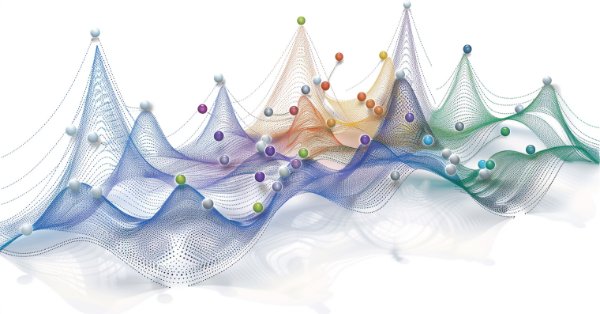
Brain Storm Optimization algorithm (Part II): Multimodality
In the second part of the article, we will move on to the practical implementation of the BSO algorithm, conduct tests on test functions and compare the efficiency of BSO with other optimization methods.
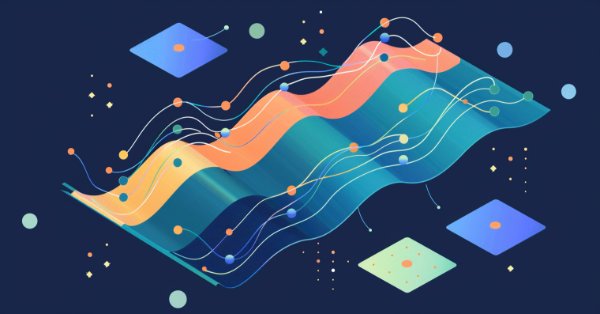
Creating Time Series Predictions using LSTM Neural Networks: Normalizing Price and Tokenizing Time
This article outlines a simple strategy for normalizing the market data using the daily range and training a neural network to enhance market predictions. The developed models may be used in conjunction with an existing technical analysis frameworks or on a standalone basis to assist in predicting the overall market direction. The framework outlined in this article may be further refined by any technical analyst to develop models suitable for both manual and automated trading strategies.
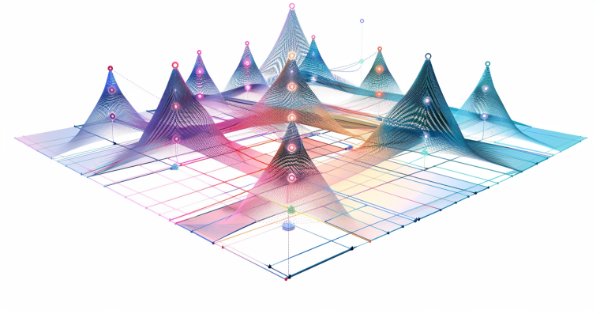
Neural networks made easy (Part 80): Graph Transformer Generative Adversarial Model (GTGAN)
In this article, I will get acquainted with the GTGAN algorithm, which was introduced in January 2024 to solve complex problems of generation architectural layouts with graph constraints.
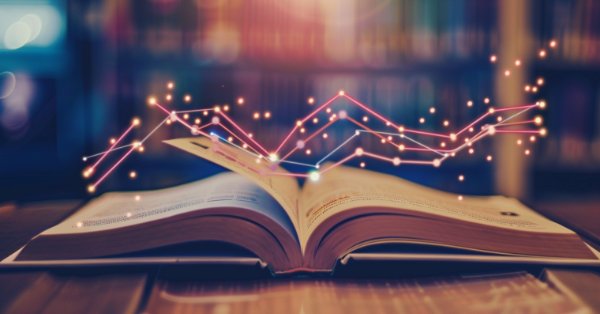
Gain An Edge Over Any Market (Part II): Forecasting Technical Indicators
Did you know that we can gain more accuracy forecasting certain technical indicators than predicting the underlying price of a traded symbol? Join us to explore how to leverage this insight for better trading strategies.
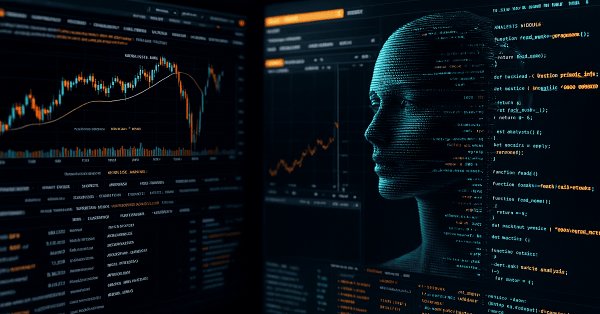
Integrating AI model into already existing MQL5 trading strategy
This topic focuses on incorporating a trained AI model (such as a reinforcement learning model like LSTM or a machine learning-based predictive model) into an existing MQL5 trading strategy.
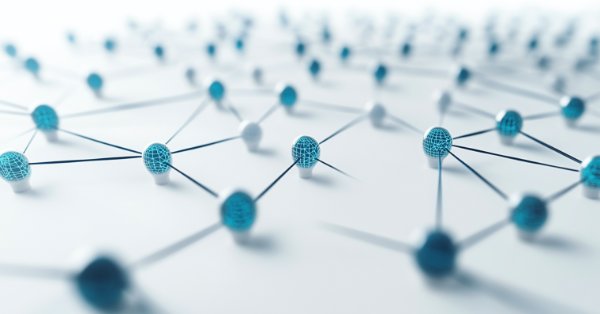
Example of Auto Optimized Take Profits and Indicator Parameters with SMA and EMA
This article presents a sophisticated Expert Advisor for forex trading, combining machine learning with technical analysis. It focuses on trading Apple stock, featuring adaptive optimization, risk management, and multiple strategies. Backtesting shows promising results with high profitability but also significant drawdowns, indicating potential for further refinement.
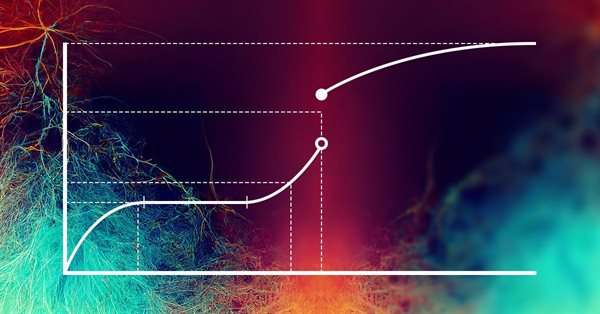
Neural networks made easy (Part 34): Fully Parameterized Quantile Function
We continue studying distributed Q-learning algorithms. In previous articles, we have considered distributed and quantile Q-learning algorithms. In the first algorithm, we trained the probabilities of given ranges of values. In the second algorithm, we trained ranges with a given probability. In both of them, we used a priori knowledge of one distribution and trained another one. In this article, we will consider an algorithm which allows the model to train for both distributions.