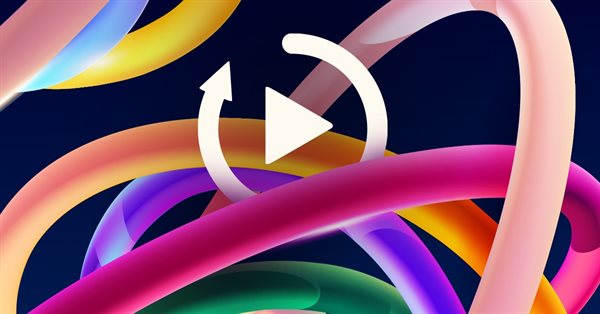
Developing a Replay System — Market simulation (Part 04): adjusting the settings (II)
Let's continue creating the system and controls. Without the ability to control the service, it is difficult to move forward and improve the system.
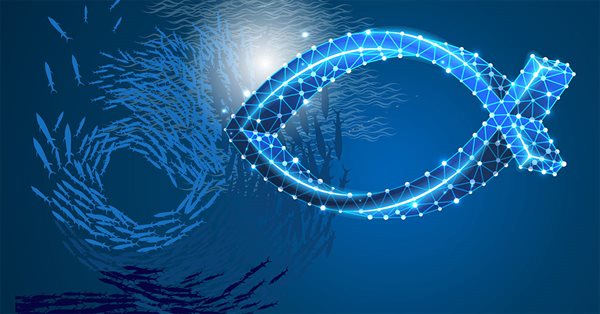
Population optimization algorithms: Fish School Search (FSS)
Fish School Search (FSS) is a new optimization algorithm inspired by the behavior of fish in a school, most of which (up to 80%) swim in an organized community of relatives. It has been proven that fish aggregations play an important role in the efficiency of foraging and protection from predators.
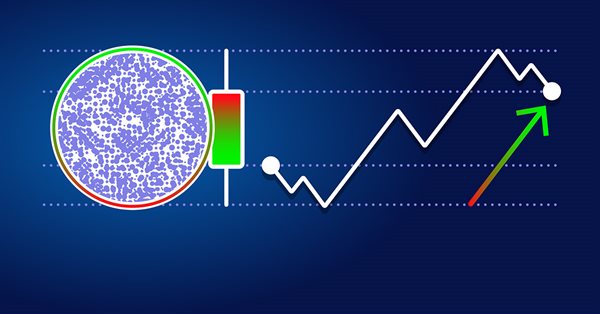
Developing a Replay System — Market simulation (Part 15): Birth of the SIMULATOR (V) - RANDOM WALK
In this article we will complete the development of a simulator for our system. The main goal here will be to configure the algorithm discussed in the previous article. This algorithm aims to create a RANDOM WALK movement. Therefore, to understand today's material, it is necessary to understand the content of previous articles. If you have not followed the development of the simulator, I advise you to read this sequence from the very beginning. Otherwise, you may get confused about what will be explained here.
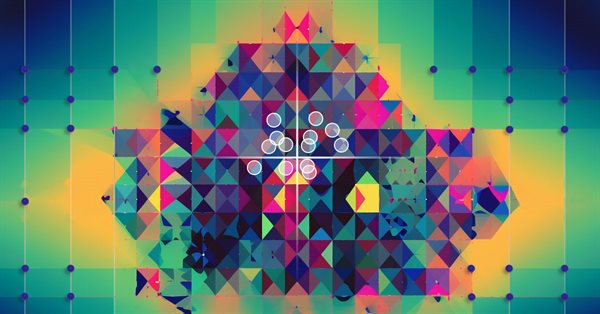
Population optimization algorithms: ElectroMagnetism-like algorithm (ЕМ)
The article describes the principles, methods and possibilities of using the Electromagnetic Algorithm in various optimization problems. The EM algorithm is an efficient optimization tool capable of working with large amounts of data and multidimensional functions.
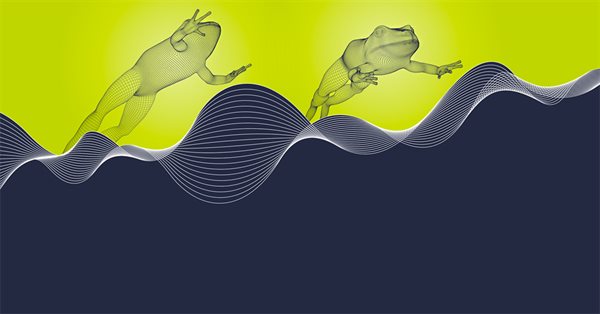
Population optimization algorithms: Shuffled Frog-Leaping algorithm (SFL)
The article presents a detailed description of the shuffled frog-leaping (SFL) algorithm and its capabilities in solving optimization problems. The SFL algorithm is inspired by the behavior of frogs in their natural environment and offers a new approach to function optimization. The SFL algorithm is an efficient and flexible tool capable of processing a variety of data types and achieving optimal solutions.
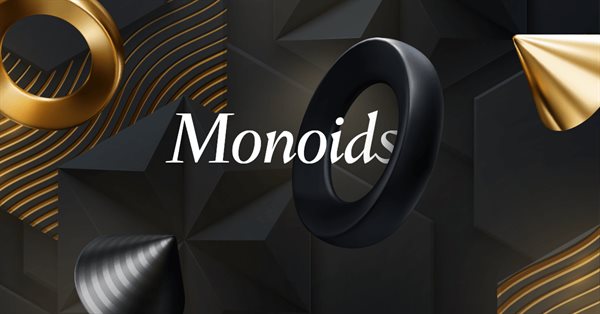
Category Theory in MQL5 (Part 8): Monoids
This article continues the series on category theory implementation in MQL5. Here we introduce monoids as domain (set) that sets category theory apart from other data classification methods by including rules and an identity element.
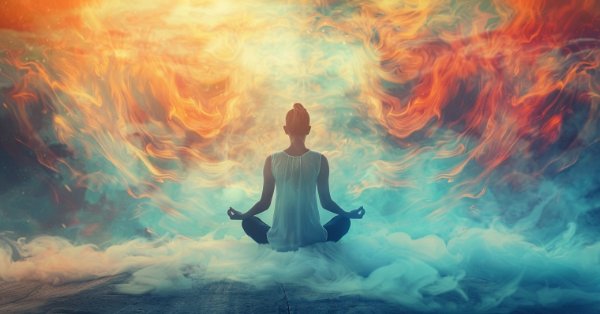
Data Science and Machine Learning(Part 21): Unlocking Neural Networks, Optimization algorithms demystified
Dive into the heart of neural networks as we demystify the optimization algorithms used inside the neural network. In this article, discover the key techniques that unlock the full potential of neural networks, propelling your models to new heights of accuracy and efficiency.
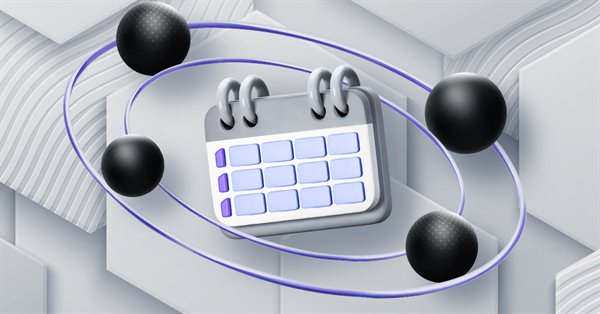
Category Theory in MQL5 (Part 15) : Functors with Graphs
This article on Category Theory implementation in MQL5, continues the series by looking at Functors but this time as a bridge between Graphs and a set. We revisit calendar data, and despite its limitations in Strategy Tester use, make the case using functors in forecasting volatility with the help of correlation.
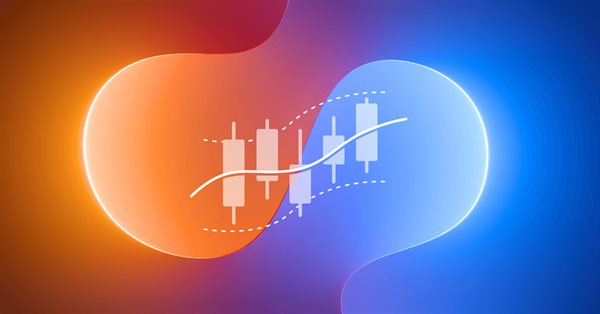
Testing and optimization of binary options strategies in MetaTrader 5
In this article, I will check and optimize binary options strategies in MetaTrader 5.
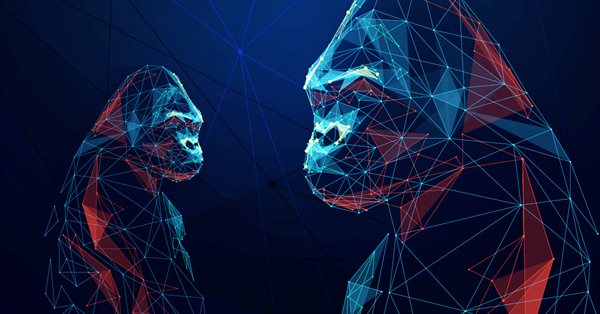
Population optimization algorithms: Monkey algorithm (MA)
In this article, I will consider the Monkey Algorithm (MA) optimization algorithm. The ability of these animals to overcome difficult obstacles and get to the most inaccessible tree tops formed the basis of the idea of the MA algorithm.
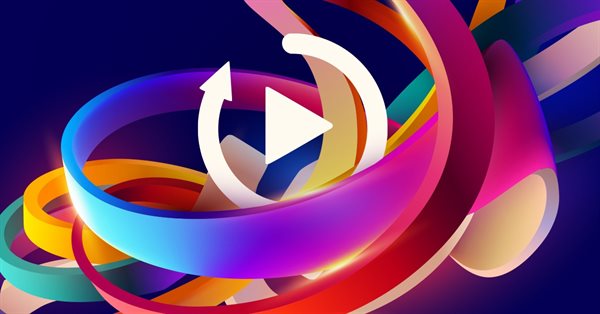
Developing a Replay System — Market simulation (Part 05): Adding Previews
We have managed to develop a way to implement the market replay system in a realistic and accessible way. Now let's continue our project and add data to improve the replay behavior.
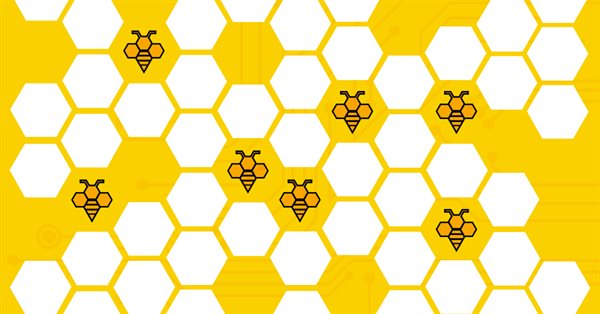
Population optimization algorithms: Artificial Bee Colony (ABC)
In this article, we will study the algorithm of an artificial bee colony and supplement our knowledge with new principles of studying functional spaces. In this article, I will showcase my interpretation of the classic version of the algorithm.
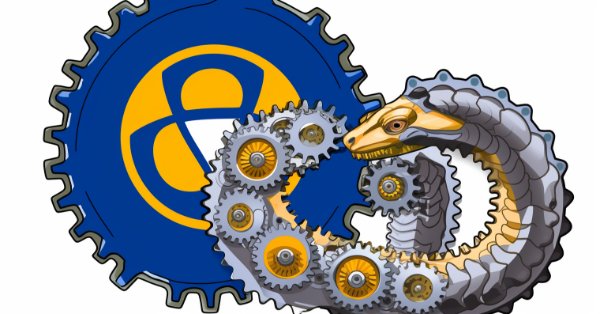
Deep Learning GRU model with Python to ONNX with EA, and GRU vs LSTM models
We will guide you through the entire process of DL with python to make a GRU ONNX model, culminating in the creation of an Expert Advisor (EA) designed for trading, and subsequently comparing GRU model with LSTN model.
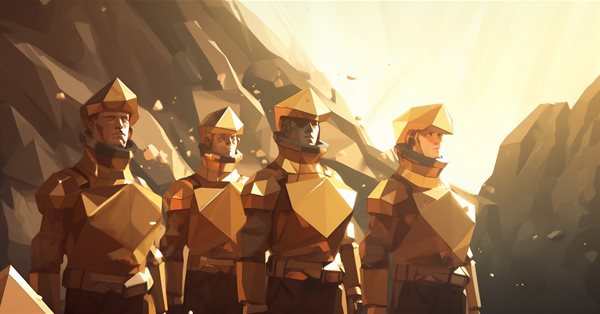
Population optimization algorithms: Stochastic Diffusion Search (SDS)
The article discusses Stochastic Diffusion Search (SDS), which is a very powerful and efficient optimization algorithm based on the principles of random walk. The algorithm allows finding optimal solutions in complex multidimensional spaces, while featuring a high speed of convergence and the ability to avoid local extrema.
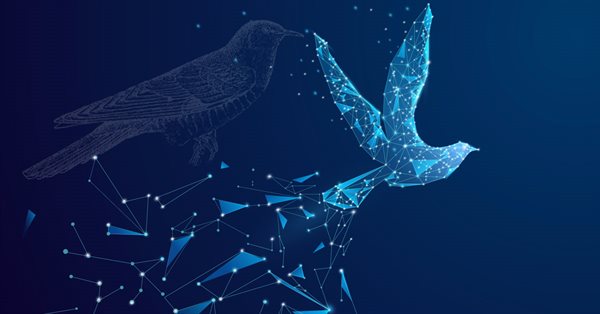
Population optimization algorithms: Cuckoo Optimization Algorithm (COA)
The next algorithm I will consider is cuckoo search optimization using Levy flights. This is one of the latest optimization algorithms and a new leader in the leaderboard.
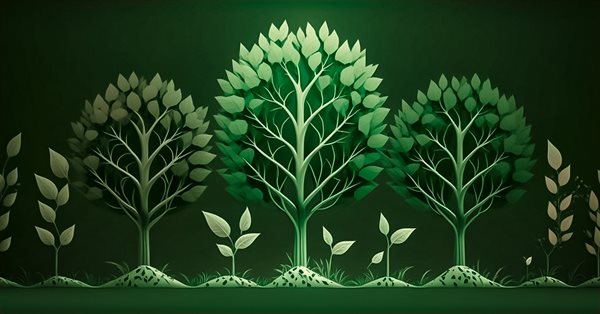
Population optimization algorithms: Saplings Sowing and Growing up (SSG)
Saplings Sowing and Growing up (SSG) algorithm is inspired by one of the most resilient organisms on the planet demonstrating outstanding capability for survival in a wide variety of conditions.
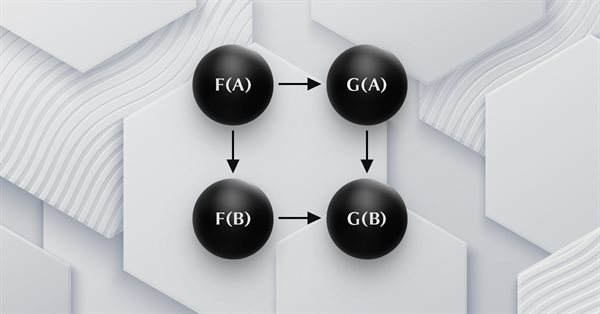
Category Theory in MQL5 (Part 18): Naturality Square
This article continues our series into category theory by introducing natural transformations, a key pillar within the subject. We look at the seemingly complex definition, then delve into examples and applications with this series’ ‘bread and butter’; volatility forecasting.
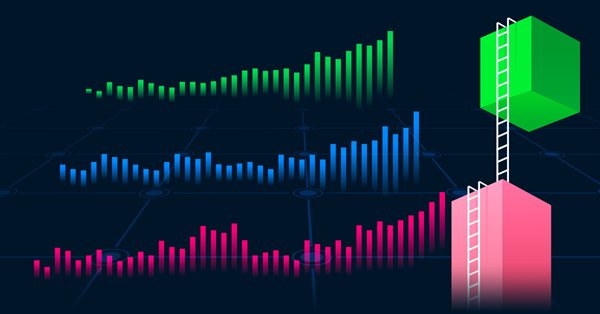
Developing a Replay System (Part 27): Expert Advisor project — C_Mouse class (I)
In this article we will implement the C_Mouse class. It provides the ability to program at the highest level. However, talking about high-level or low-level programming languages is not about including obscene words or jargon in the code. It's the other way around. When we talk about high-level or low-level programming, we mean how easy or difficult the code is for other programmers to understand.
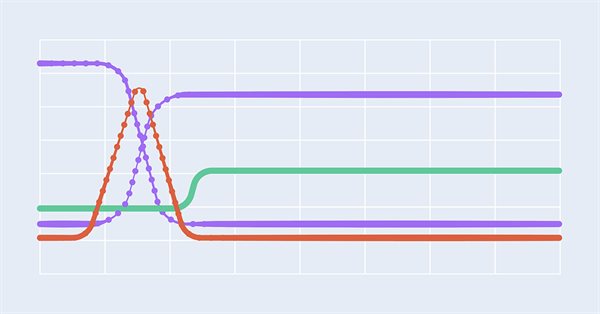
Multilayer perceptron and backpropagation algorithm (Part 3): Integration with the Strategy Tester - Overview (I).
The multilayer perceptron is an evolution of the simple perceptron which can solve non-linear separable problems. Together with the backpropagation algorithm, this neural network can be effectively trained. In Part 3 of the Multilayer Perceptron and Backpropagation series, we'll see how to integrate this technique into the Strategy Tester. This integration will allow the use of complex data analysis aimed at making better decisions to optimize your trading strategies. In this article, we will discuss the advantages and problems of this technique.
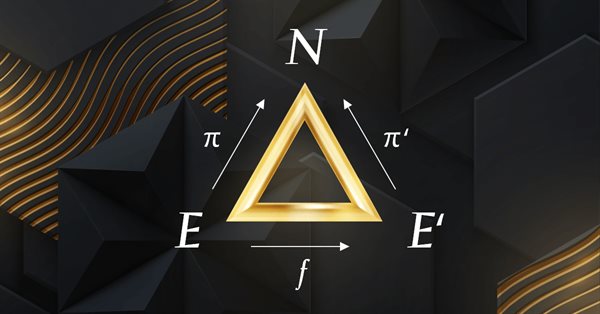
Category Theory in MQL5 (Part 7): Multi, Relative and Indexed Domains
Category Theory is a diverse and expanding branch of Mathematics which is only recently getting some coverage in the MQL5 community. These series of articles look to explore and examine some of its concepts & axioms with the overall goal of establishing an open library that provides insight while also hopefully furthering the use of this remarkable field in Traders' strategy development.
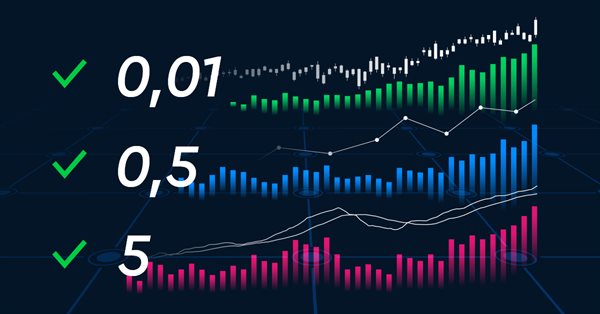
Developing a Replay System (Part 26): Expert Advisor project — C_Terminal class
We can now start creating an Expert Advisor for use in the replay/simulation system. However, we need something improved, not a random solution. Despite this, we should not be intimidated by the initial complexity. It's important to start somewhere, otherwise we end up ruminating about the difficulty of a task without even trying to overcome it. That's what programming is all about: overcoming obstacles through learning, testing, and extensive research.
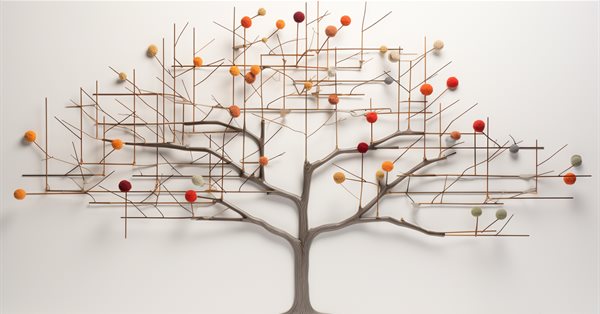
Python, ONNX and MetaTrader 5: Creating a RandomForest model with RobustScaler and PolynomialFeatures data preprocessing
In this article, we will create a random forest model in Python, train the model, and save it as an ONNX pipeline with data preprocessing. After that we will use the model in the MetaTrader 5 terminal.
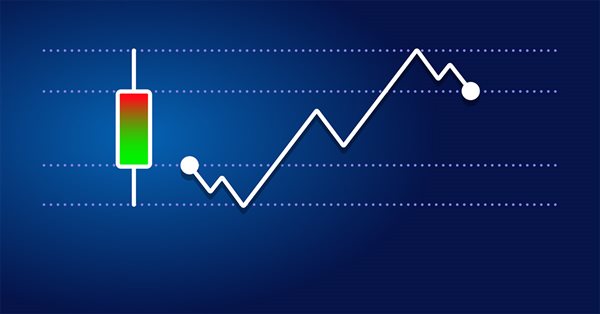
Developing a Replay System — Market simulation (Part 14): Birth of the SIMULATOR (IV)
In this article we will continue the simulator development stage. this time we will see how to effectively create a RANDOM WALK type movement. This type of movement is very intriguing because it forms the basis of everything that happens in the capital market. In addition, we will begin to understand some concepts that are fundamental to those conducting market analysis.
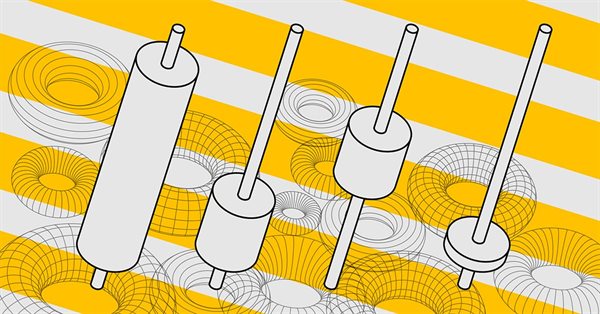
Developing a Replay System — Market simulation (Part 11): Birth of the SIMULATOR (I)
In order to use the data that forms the bars, we must abandon replay and start developing a simulator. We will use 1 minute bars because they offer the least amount of difficulty.
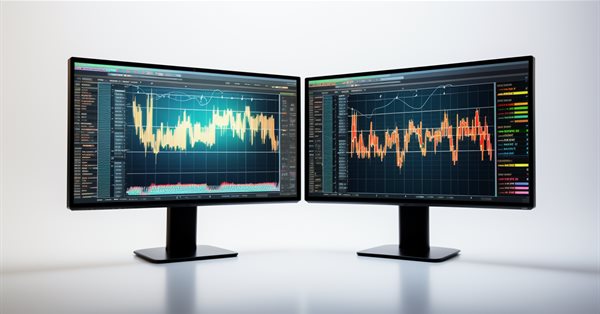
Developing a Replay System (Part 32): Order System (I)
Of all the things that we have developed so far, this system, as you will probably notice and eventually agree, is the most complex. Now we need to do something very simple: make our system simulate the operation of a trading server. This need to accurately implement the way the trading server operates seems like a no-brainer. At least in words. But we need to do this so that the everything is seamless and transparent for the user of the replay/simulation system.
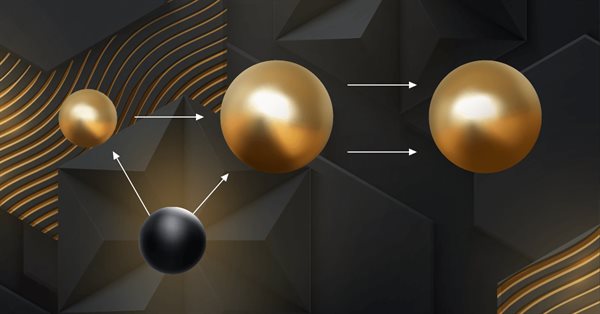
Category Theory in MQL5 (Part 5): Equalizers
Category Theory is a diverse and expanding branch of Mathematics which is only recently getting some coverage in the MQL5 community. These series of articles look to explore and examine some of its concepts & axioms with the overall goal of establishing an open library that provides insight while also hopefully furthering the use of this remarkable field in Traders' strategy development.
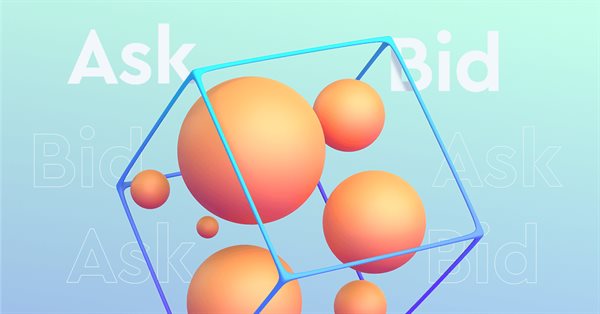
Developing a Replay System — Market simulation (Part 17): Ticks and more ticks (I)
Here we will see how to implement something really interesting, but at the same time very difficult due to certain points that can be very confusing. The worst thing that can happen is that some traders who consider themselves professionals do not know anything about the importance of these concepts in the capital market. Well, although we focus here on programming, understanding some of the issues involved in market trading is paramount to what we are going to implement.
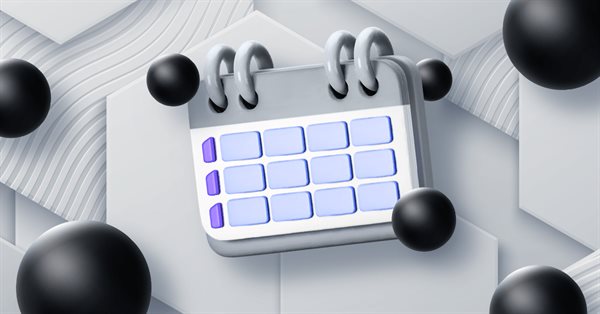
Category Theory in MQL5 (Part 13): Calendar Events with Database Schemas
This article, that follows Category Theory implementation of Orders in MQL5, considers how database schemas can be incorporated for classification in MQL5. We take an introductory look at how database schema concepts could be married with category theory when identifying trade relevant text(string) information. Calendar events are the focus.
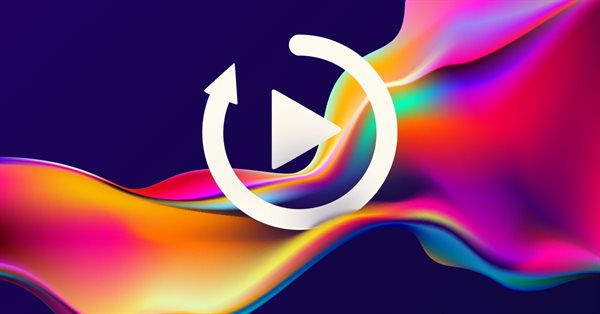
Developing a Replay System — Market simulation (Part 08): Locking the indicator
In this article, we will look at how to lock the indicator while simply using the MQL5 language, and we will do it in a very interesting and amazing way.

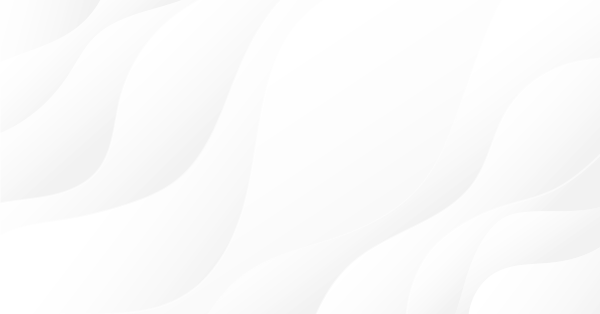
Developing a Replay System — Market simulation (Part 10): Using only real data for Replay
Here we will look at how we can use more reliable data (traded ticks) in the replay system without worrying about whether it is adjusted or not.
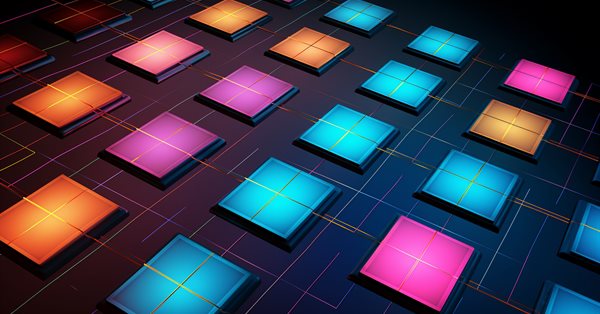
Modified Grid-Hedge EA in MQL5 (Part II): Making a Simple Grid EA
In this article, we explored the classic grid strategy, detailing its automation using an Expert Advisor in MQL5 and analyzing initial backtest results. We highlighted the strategy's need for high holding capacity and outlined plans for optimizing key parameters like distance, takeProfit, and lot sizes in future installments. The series aims to enhance trading strategy efficiency and adaptability to different market conditions.
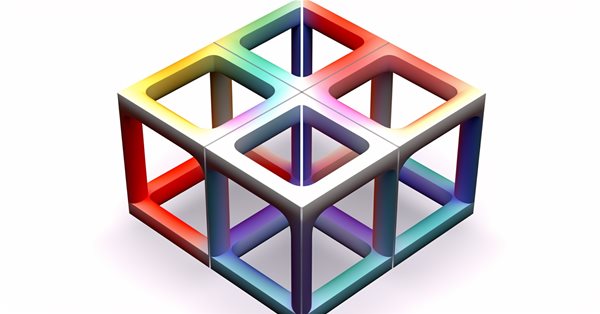
Population optimization algorithms: Nelder–Mead, or simplex search (NM) method
The article presents a complete exploration of the Nelder-Mead method, explaining how the simplex (function parameter space) is modified and rearranged at each iteration to achieve an optimal solution, and describes how the method can be improved.
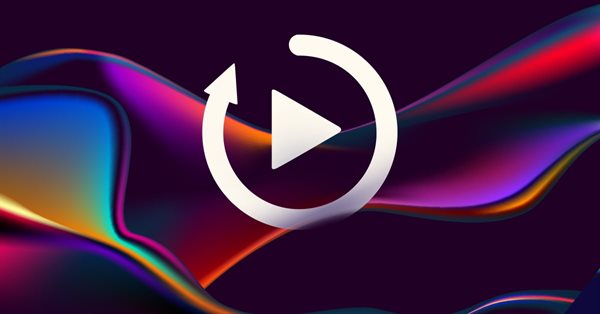
Developing a Replay System — Market simulation (Part 09): Custom events
Here we'll see how custom events are triggered and how the indicator reports the state of the replay/simulation service.
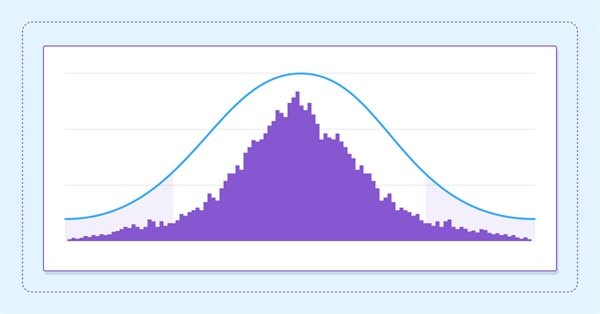
Estimate future performance with confidence intervals
In this article we delve into the application of boostrapping techniques as a means to estimate the future performance of an automated strategy.
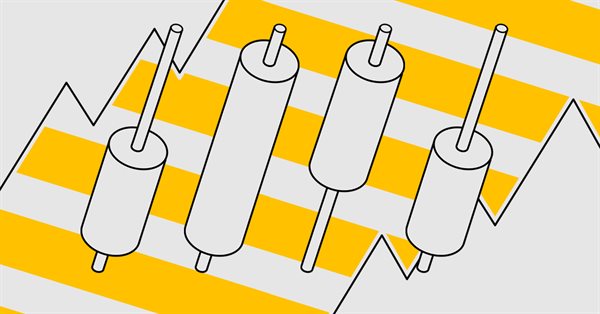
Developing a Replay System — Market simulation (Part 13): Birth of the SIMULATOR (III)
Here we will simplify a few elements related to the work in the next article. I'll also explain how you can visualize what the simulator generates in terms of randomness.
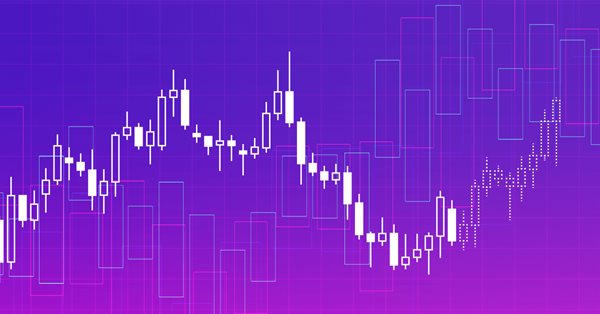
Developing a Replay System — Market simulation (Part 23): FOREX (IV)
Now the creation occurs at the same point where we converted ticks into bars. This way, if something goes wrong during the conversion process, we will immediately notice the error. This is because the same code that places 1-minute bars on the chart during fast forwarding is also used for the positioning system to place bars during normal performance. In other words, the code that is responsible for this task is not duplicated anywhere else. This way we get a much better system for both maintenance and improvement.
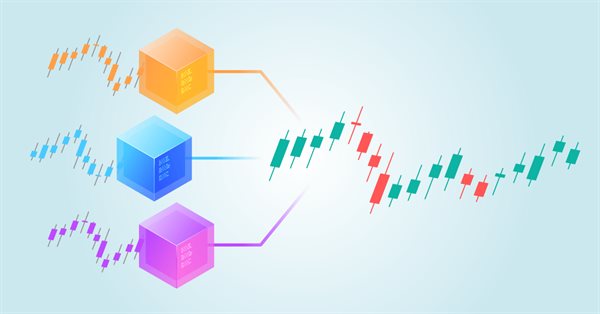
Developing a Replay System — Market simulation (Part 19): Necessary adjustments
Here we will prepare the ground so that if we need to add new functions to the code, this will happen smoothly and easily. The current code cannot yet cover or handle some of the things that will be necessary to make meaningful progress. We need everything to be structured in order to enable the implementation of certain things with the minimal effort. If we do everything correctly, we can get a truly universal system that can very easily adapt to any situation that needs to be handled.
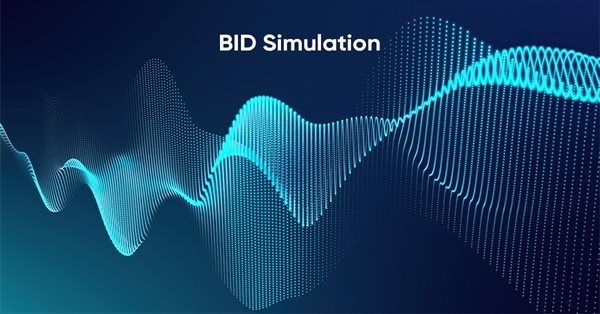
Developing a Replay System — Market simulation (Part 24): FOREX (V)
Today we will remove a limitation that has been preventing simulations based on the Last price and will introduce a new entry point specifically for this type of simulation. The entire operating mechanism will be based on the principles of the forex market. The main difference in this procedure is the separation of Bid and Last simulations. However, it is important to note that the methodology used to randomize the time and adjust it to be compatible with the C_Replay class remains identical in both simulations. This is good because changes in one mode lead to automatic improvements in the other, especially when it comes to handling time between ticks.
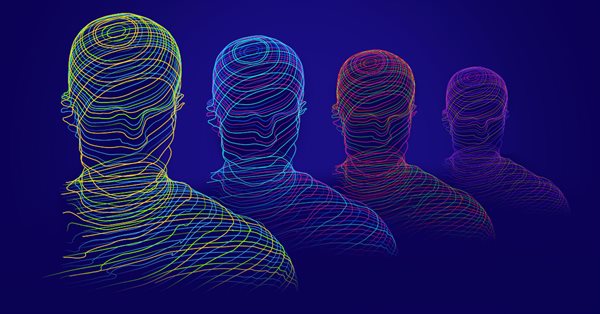
Population optimization algorithms: Mind Evolutionary Computation (MEC) algorithm
The article considers the algorithm of the MEC family called the simple mind evolutionary computation algorithm (Simple MEC, SMEC). The algorithm is distinguished by the beauty of its idea and ease of implementation.
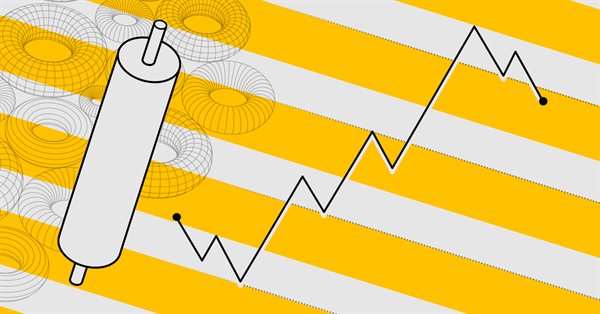
Developing a Replay System — Market simulation (Part 12): Birth of the SIMULATOR (II)
Developing a simulator can be much more interesting than it seems. Today we'll take a few more steps in this direction because things are getting more interesting.