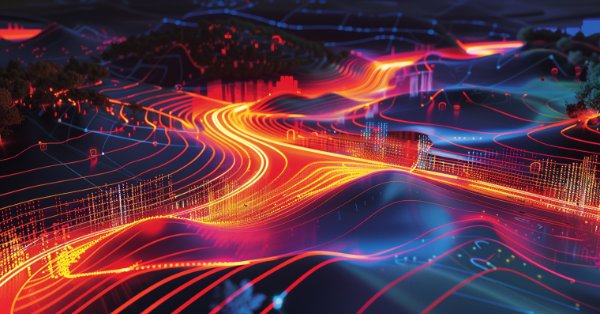
Neural networks made easy (Part 78): Decoder-free Object Detector with Transformer (DFFT)
In this article, I propose to look at the issue of building a trading strategy from a different angle. We will not predict future price movements, but will try to build a trading system based on the analysis of historical data.
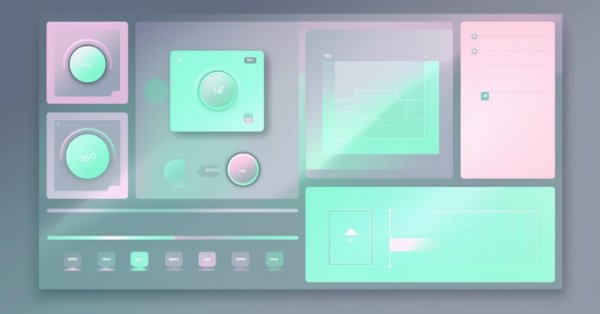
Creating an Interactive Graphical User Interface in MQL5 (Part 1): Making the Panel
This article explores the fundamental steps in crafting and implementing a Graphical User Interface (GUI) panel using MetaQuotes Language 5 (MQL5). Custom utility panels enhance user interaction in trading by simplifying common tasks and visualizing essential trading information. By creating custom panels, traders can streamline their workflow and save time during trading operations.
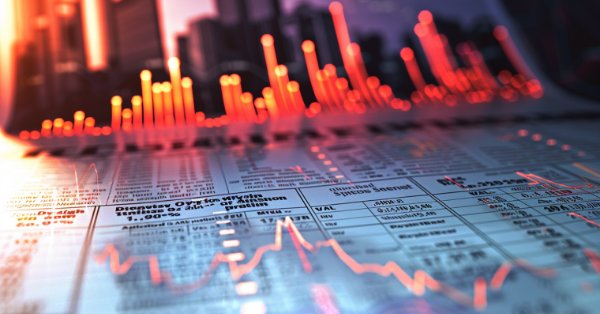
Sentiment Analysis and Deep Learning for Trading with EA and Backtesting with Python
In this article, we will introduce Sentiment Analysis and ONNX Models with Python to be used in an EA. One script runs a trained ONNX model from TensorFlow for deep learning predictions, while another fetches news headlines and quantifies sentiment using AI.
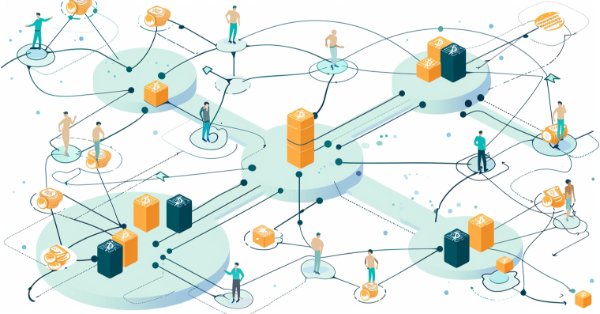
Neural networks made easy (Part 77): Cross-Covariance Transformer (XCiT)
In our models, we often use various attention algorithms. And, probably, most often we use Transformers. Their main disadvantage is the resource requirement. In this article, we will consider a new algorithm that can help reduce computing costs without losing quality.
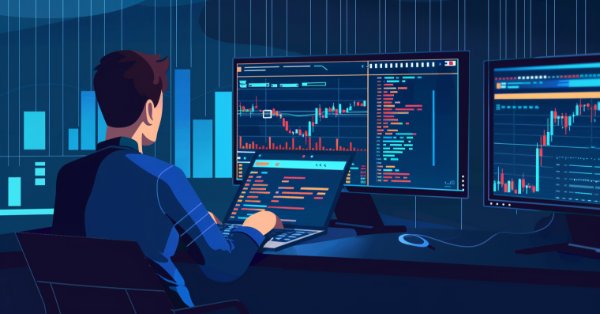
Developing Zone Recovery Martingale strategy in MQL5
The article discusses, in a detailed perspective, the steps that need to be implemented towards the creation of an expert advisor based on the Zone Recovery trading algorithm. This helps aotomate the system saving time for algotraders.
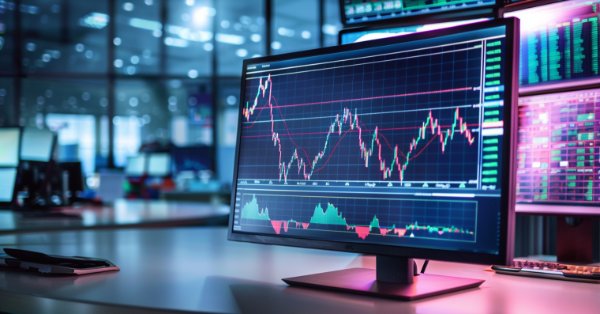
Mastering Market Dynamics: Creating a Support and Resistance Strategy Expert Advisor (EA)
A comprehensive guide to developing an automated trading algorithm based on the Support and Resistance strategy. Detailed information on all aspects of creating an expert advisor in MQL5 and testing it in MetaTrader 5 – from analyzing price range behaviors to risk management.
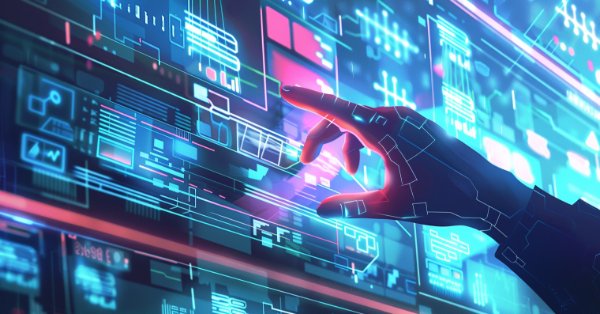
Developing a multi-currency Expert Advisor (Part 4): Pending virtual orders and saving status
Having started developing a multi-currency EA, we have already achieved some results and managed to carry out several code improvement iterations. However, our EA was unable to work with pending orders and resume operation after the terminal restart. Let's add these features.
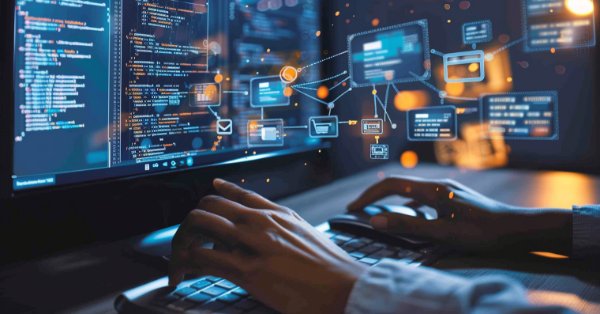
Multibot in MetaTrader (Part II): Improved dynamic template
Developing the theme of the previous article, I decided to create a more flexible and functional template that has greater capabilities and can be effectively used both in freelancing and as a base for developing multi-currency and multi-period EAs with the ability to integrate with external solutions.
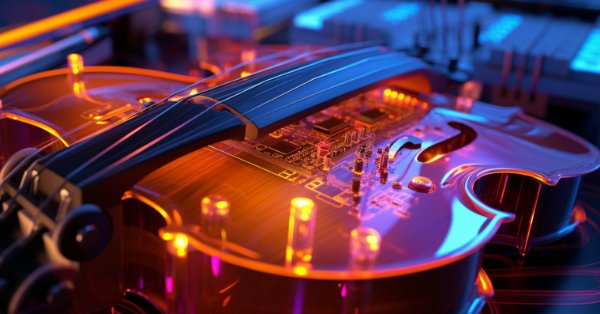
Neural networks made easy (Part 76): Exploring diverse interaction patterns with Multi-future Transformer
This article continues the topic of predicting the upcoming price movement. I invite you to get acquainted with the Multi-future Transformer architecture. Its main idea is to decompose the multimodal distribution of the future into several unimodal distributions, which allows you to effectively simulate various models of interaction between agents on the scene.
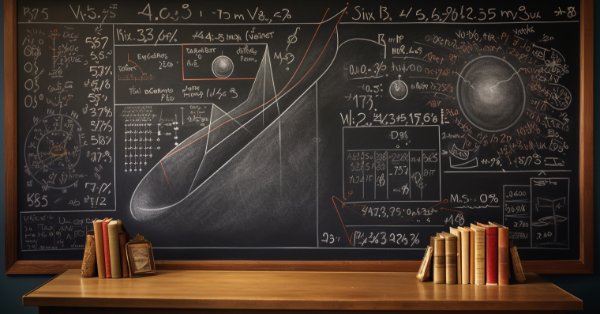
Neural networks made easy (Part 75): Improving the performance of trajectory prediction models
The models we create are becoming larger and more complex. This increases the costs of not only their training as well as operation. However, the time required to make a decision is often critical. In this regard, let us consider methods for optimizing model performance without loss of quality.
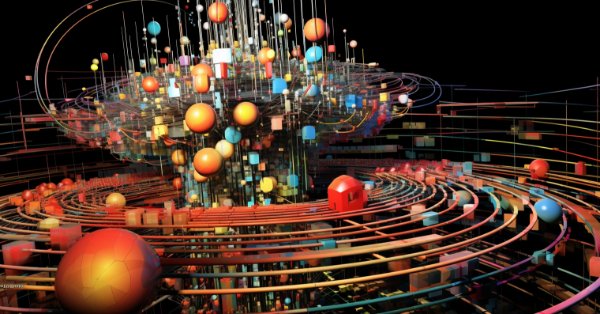
Neural networks made easy (Part 74): Trajectory prediction with adaptation
This article introduces a fairly effective method of multi-agent trajectory forecasting, which is able to adapt to various environmental conditions.
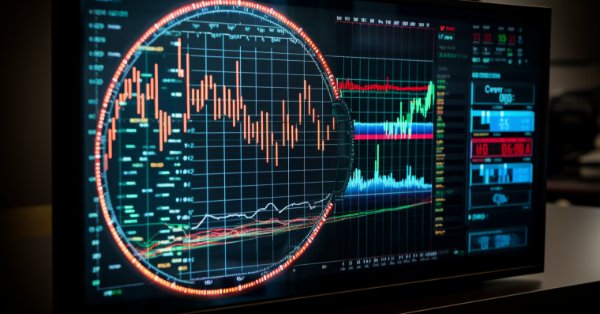
Developing a multi-currency Expert Advisor (Part 3): Architecture revision
We have already made some progress in developing a multi-currency EA with several strategies working in parallel. Considering the accumulated experience, let's review the architecture of our solution and try to improve it before we go too far ahead.
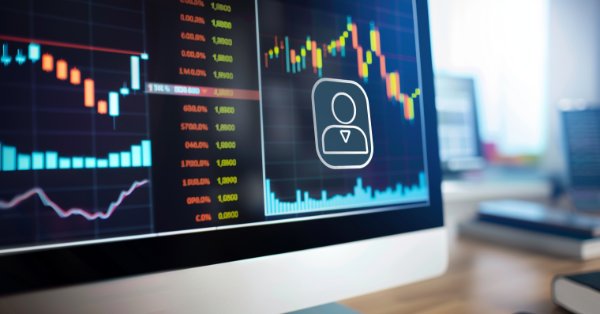
A Step-by-Step Guide on Trading the Break of Structure (BoS) Strategy
A comprehensive guide to developing an automated trading algorithm based on the Break of Structure (BoS) strategy. Detailed information on all aspects of creating an advisor in MQL5 and testing it in MetaTrader 5 — from analyzing price support and resistance to risk management
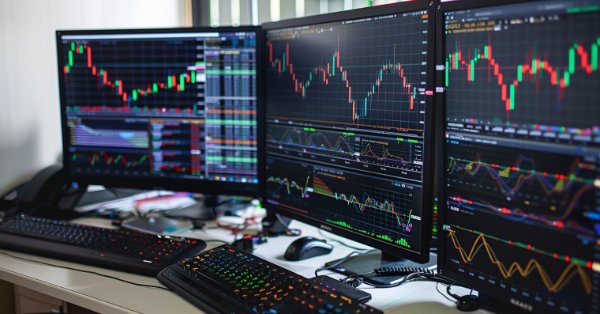
Building A Candlestick Trend Constraint Model (Part 4): Customizing Display Style For Each Trend Wave
In this article, we will explore the capabilities of the powerful MQL5 language in drawing various indicator styles on Meta Trader 5. We will also look at scripts and how they can be used in our model.
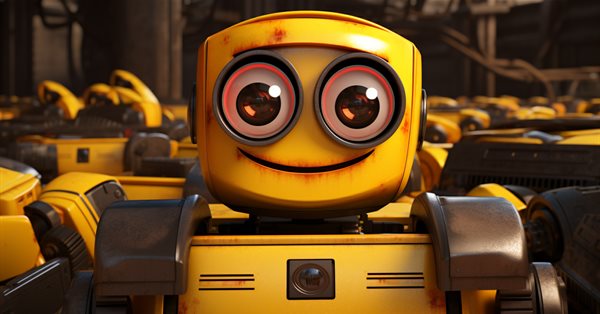
Neural networks made easy (Part 73): AutoBots for predicting price movements
We continue to discuss algorithms for training trajectory prediction models. In this article, we will get acquainted with a method called "AutoBots".
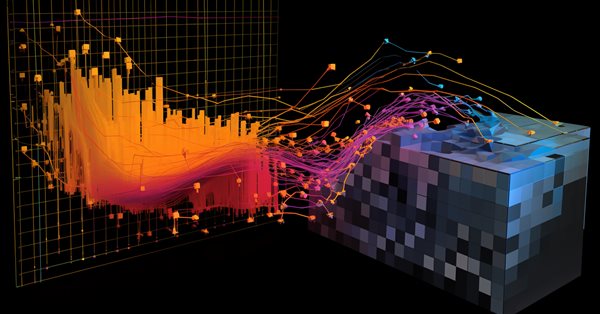
Neural networks made easy (Part 72): Trajectory prediction in noisy environments
The quality of future state predictions plays an important role in the Goal-Conditioned Predictive Coding method, which we discussed in the previous article. In this article I want to introduce you to an algorithm that can significantly improve the prediction quality in stochastic environments, such as financial markets.
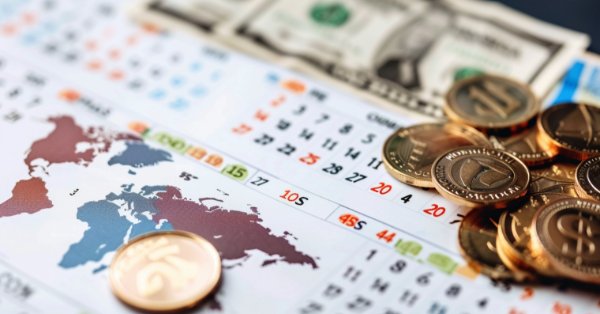
News Trading Made Easy (Part 2): Risk Management
In this article, inheritance will be introduced into our previous and new code. A new database design will be implemented to provide efficiency. Additionally, a risk management class will be created to tackle volume calculations.
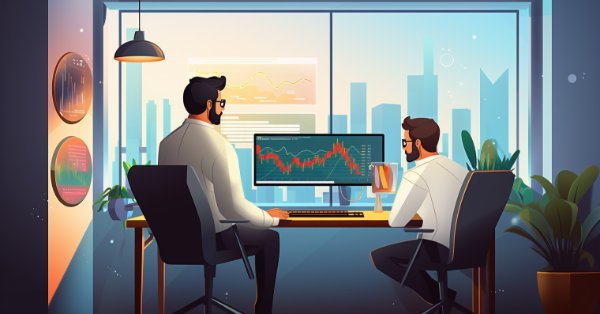
Developing a multi-currency Expert Advisor (Part 2): Transition to virtual positions of trading strategies
Let's continue developing a multi-currency EA with several strategies working in parallel. Let's try to move all the work associated with opening market positions from the strategy level to the level of the EA managing the strategies. The strategies themselves will trade only virtually, without opening market positions.
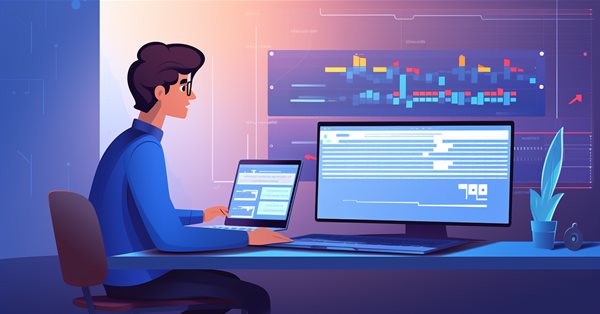
Master MQL5 from beginner to pro (Part II): Basic data types and use of variable
This is a continuation of the series for beginners. In this article, we'll look at how to create constants and variables, write dates, colors, and other useful data. We will learn how to create enumerations like days of the week or line styles (solid, dotted, etc.). Variables and expressions are the basis of programming. They are definitely present in 99% of programs, so understanding them is critical. Therefore, if you are new to programming, this article can be very useful for you. Required programming knowledge level: very basic, within the limits of my previous article (see the link at the beginning).
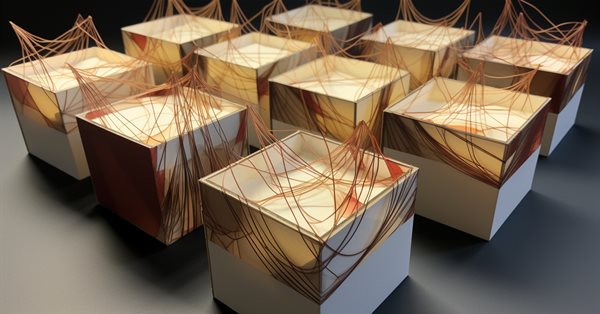
Neural networks made easy (Part 71): Goal-Conditioned Predictive Coding (GCPC)
In previous articles, we discussed the Decision Transformer method and several algorithms derived from it. We experimented with different goal setting methods. During the experiments, we worked with various ways of setting goals. However, the model's study of the earlier passed trajectory always remained outside our attention. In this article. I want to introduce you to a method that fills this gap.
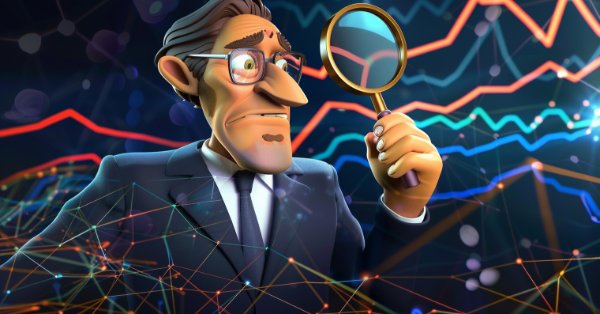
Bill Williams Strategy with and without other indicators and predictions
In this article, we will take a look to one the famous strategies of Bill Williams, and discuss it, and try to improve the strategy with other indicators and with predictions.
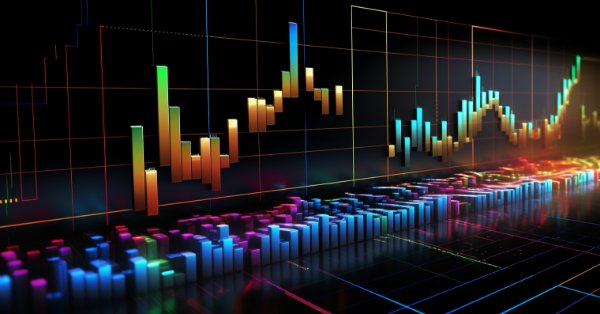
Trailing stop in trading
In this article, we will look at the use of a trailing stop in trading. We will assess how useful and effective it is, and how it can be used. The efficiency of a trailing stop largely depends on price volatility and the selection of the stop loss level. A variety of approaches can be used to set a stop loss.
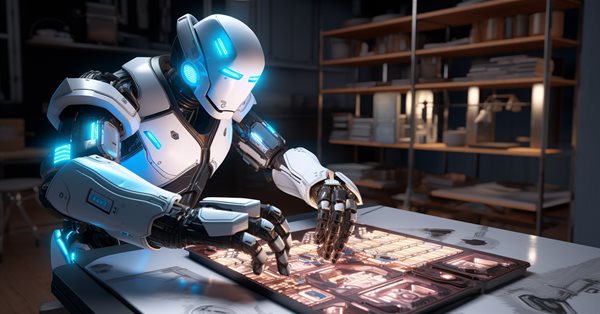
Neural networks made easy (Part 70): Closed-Form Policy Improvement Operators (CFPI)
In this article, we will get acquainted with an algorithm that uses closed-form policy improvement operators to optimize Agent actions in offline mode.
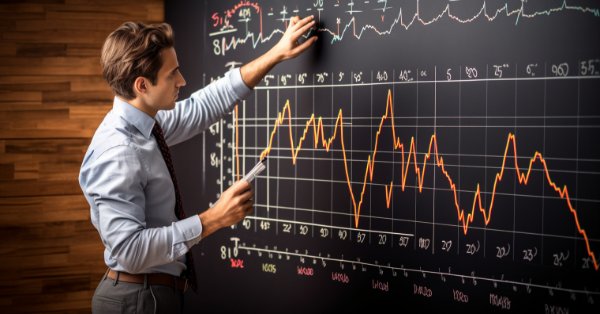
Developing a multi-currency Expert Advisor (Part 1): Collaboration of several trading strategies
There are quite a lot of different trading strategies. So, it might be useful to apply several strategies working in parallel to diversify risks and increase the stability of trading results. But if each strategy is implemented as a separate Expert Advisor (EA), then managing their work on one trading account becomes much more difficult. To solve this problem, it would be reasonable to implement the operation of different trading strategies within a single EA.
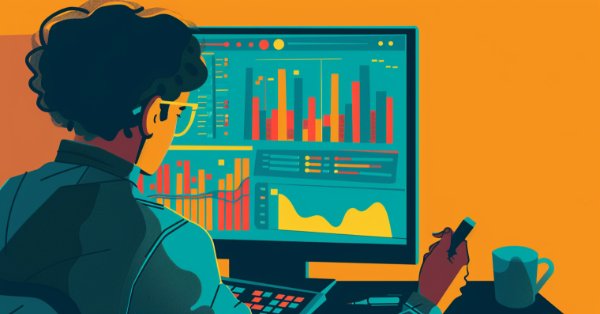
Learn how to trade the Fair Value Gap (FVG)/Imbalances step-by-step: A Smart Money concept approach
A step-by-step guide to creating and implementing an automated trading algorithm in MQL5 based on the Fair Value Gap (FVG) trading strategy. A detailed tutorial on creating an expert advisor that can be useful for both beginners and experienced traders.
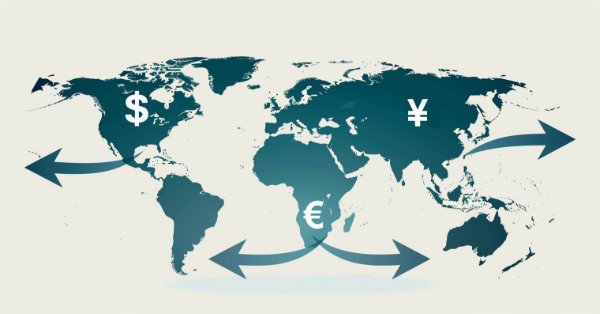
Triangular arbitrage with predictions
This article simplifies triangular arbitrage, showing you how to use predictions and specialized software to trade currencies smarter, even if you're new to the market. Ready to trade with expertise?
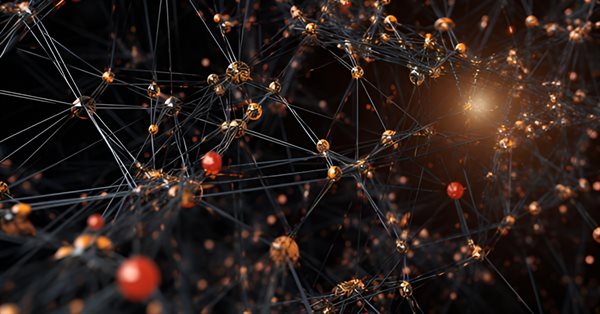
Neural networks made easy (Part 69): Density-based support constraint for the behavioral policy (SPOT)
In offline learning, we use a fixed dataset, which limits the coverage of environmental diversity. During the learning process, our Agent can generate actions beyond this dataset. If there is no feedback from the environment, how can we be sure that the assessments of such actions are correct? Maintaining the Agent's policy within the training dataset becomes an important aspect to ensure the reliability of training. This is what we will talk about in this article.
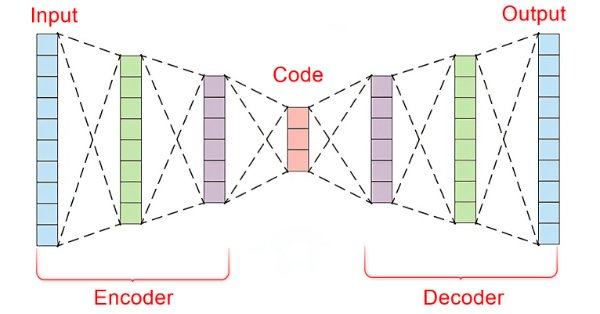
Data Science and Machine Learning (Part 22): Leveraging Autoencoders Neural Networks for Smarter Trades by Moving from Noise to Signal
In the fast-paced world of financial markets, separating meaningful signals from the noise is crucial for successful trading. By employing sophisticated neural network architectures, autoencoders excel at uncovering hidden patterns within market data, transforming noisy input into actionable insights. In this article, we explore how autoencoders are revolutionizing trading practices, offering traders a powerful tool to enhance decision-making and gain a competitive edge in today's dynamic markets.
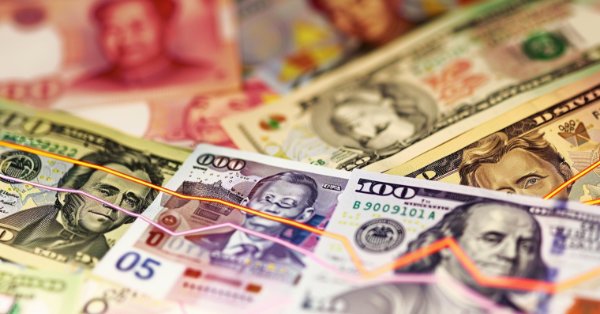
MQL5 Wizard Techniques you should know (Part 17): Multicurrency Trading
Trading across multiple currencies is not available by default when an expert advisor is assembled via the wizard. We examine 2 possible hacks traders can make when looking to test their ideas off more than one symbol at a time.
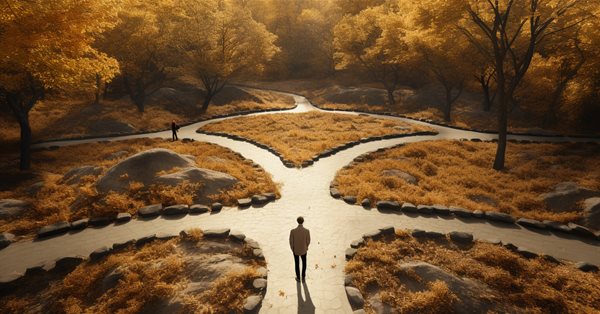
Neural networks made easy (Part 68): Offline Preference-guided Policy Optimization
Since the first articles devoted to reinforcement learning, we have in one way or another touched upon 2 problems: exploring the environment and determining the reward function. Recent articles have been devoted to the problem of exploration in offline learning. In this article, I would like to introduce you to an algorithm whose authors completely eliminated the reward function.
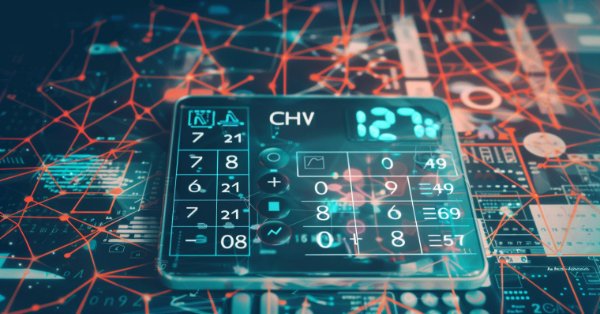
How to build and optimize a volatility-based trading system (Chaikin Volatility - CHV)
In this article, we will provide another volatility-based indicator named Chaikin Volatility. We will understand how to build a custom indicator after identifying how it can be used and constructed. We will share some simple strategies that can be used and then test them to understand which one can be better.
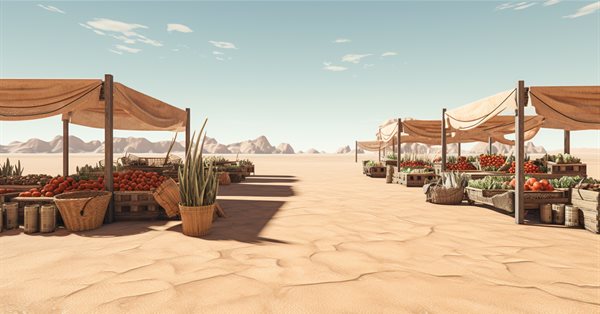
Creating a market making algorithm in MQL5
How do market makers work? Let's consider this issue and create a primitive market-making algorithm.
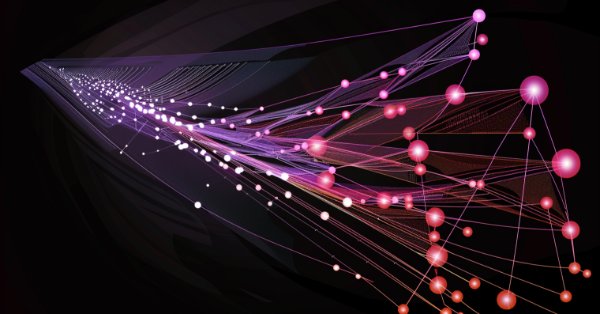
MQL5 Wizard Techniques you should know (Part 16): Principal Component Analysis with Eigen Vectors
Principal Component Analysis, a dimensionality reducing technique in data analysis, is looked at in this article, with how it could be implemented with Eigen values and vectors. As always, we aim to develop a prototype expert-signal-class usable in the MQL5 wizard.
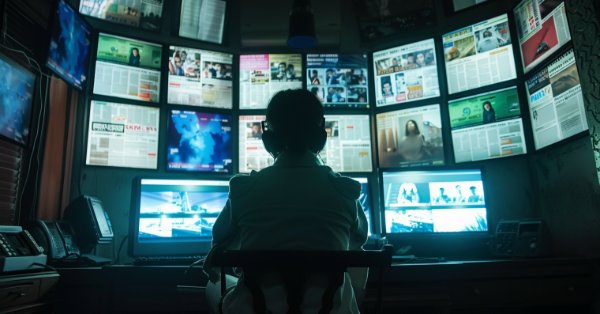
News Trading Made Easy (Part 1): Creating a Database
News trading can be complicated and overwhelming, in this article we will go through steps to obtain news data. Additionally we will learn about the MQL5 Economic Calendar and what it has to offer.
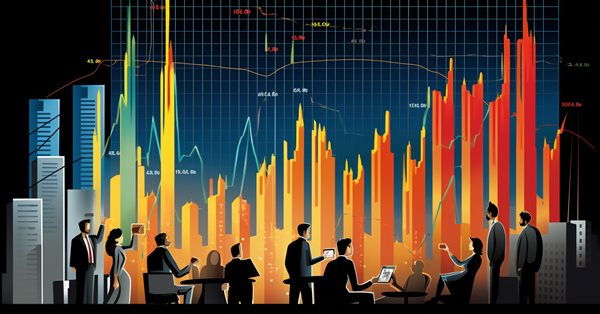
Quantitative analysis in MQL5: Implementing a promising algorithm
We will analyze the question of what quantitative analysis is and how it is used by major players. We will create one of the quantitative analysis algorithms in the MQL5 language.
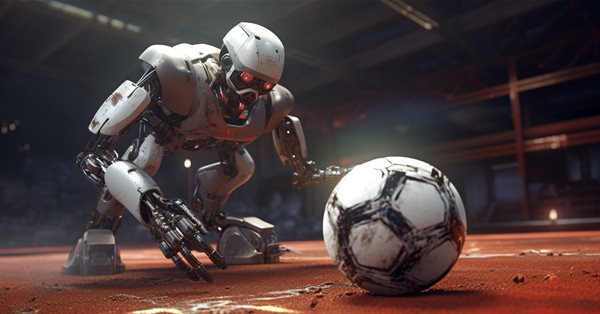
Neural networks made easy (Part 67): Using past experience to solve new tasks
In this article, we continue discussing methods for collecting data into a training set. Obviously, the learning process requires constant interaction with the environment. However, situations can be different.
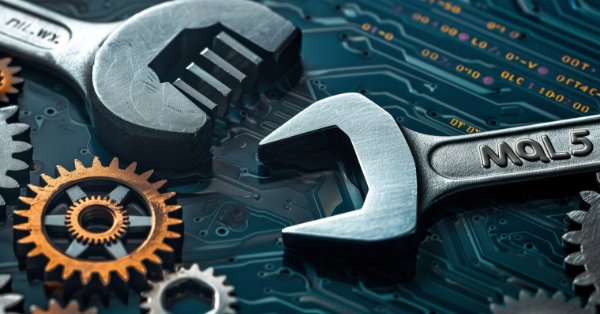
Build Self Optmising Expert Advisors in MQL5
Build expert advisors that look forward and adjust themselves to any market.
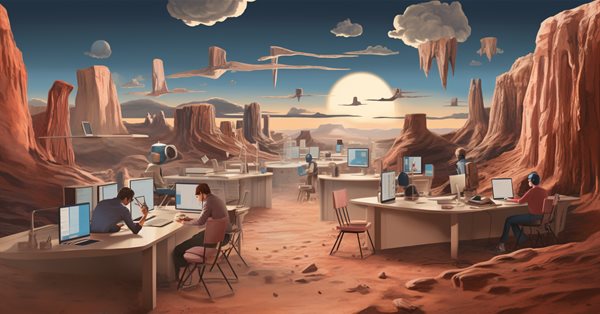
Neural networks made easy (Part 66): Exploration problems in offline learning
Models are trained offline using data from a prepared training dataset. While providing certain advantages, its negative side is that information about the environment is greatly compressed to the size of the training dataset. Which, in turn, limits the possibilities of exploration. In this article, we will consider a method that enables the filling of a training dataset with the most diverse data possible.
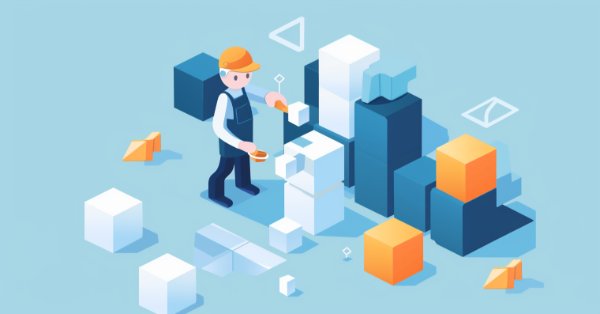
Introduction to MQL5 (Part 6): A Beginner's Guide to Array Functions in MQL5 (II)
Embark on the next phase of our MQL5 journey. In this insightful and beginner-friendly article, we'll look into the remaining array functions, demystifying complex concepts to empower you to craft efficient trading strategies. We’ll be discussing ArrayPrint, ArrayInsert, ArraySize, ArrayRange, ArrarRemove, ArraySwap, ArrayReverse, and ArraySort. Elevate your algorithmic trading expertise with these essential array functions. Join us on the path to MQL5 mastery!
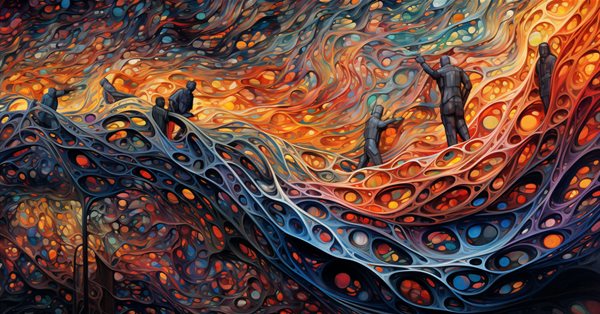
Neural networks made easy (Part 65): Distance Weighted Supervised Learning (DWSL)
In this article, we will get acquainted with an interesting algorithm that is built at the intersection of supervised and reinforcement learning methods.