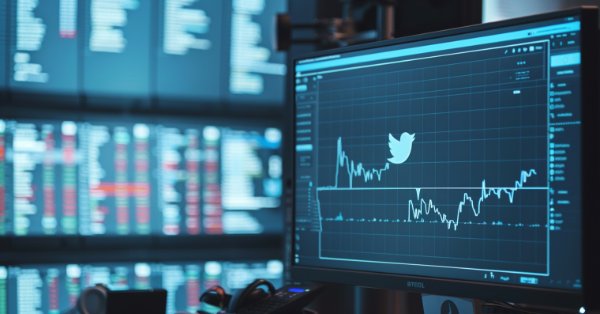
ソケットを使ったツイッターのセンチメント分析
はじめに
本稿では、ソーシャルメディアプラットフォームからのリアルタイムセンチメント分析を活用して売買の意思決定をおこなう高度な取引ボットを紹介します。このボットは、MetaTrader 5 (MT5)とPythonベースのセンチメント分析エンジンを統合しており、クオンツファイナンスと自然言語処理の最先端の融合を象徴しています。
ボットのアーキテクチャは、MT5の取引機能とPythonのデータ処理能力のギャップを埋めるために、ソケット通信を利用したクライアントサーバーモデルに基づいて構築されています。中心的な機能は、特定の金融商品に関連するツイッターのセンチメントを分析し、ソーシャルメディアの話題を実用的な売買シグナルに変換することです。
この革新的なアプローチは、金融における学際的技術の可能性を示すだけでなく、現代の取引戦略における代替データソースの重要性の高まりを浮き彫りにしています。ボットの機能とコード構造を深く掘り下げ、ソーシャルメディアデータの処理、ネットワーク通信の管理、センチメントスコアに基づく取引の実行方法を探求します。
以下の分析では、MQL5で書かれたMetaTrader 5エキスパートアドバイザー(EA)とPythonサーバーの両方について説明しながら、ボットのコンポーネントに関する洞察を提供します。 その複雑な相互作用、センチメント分析手法、実装された取引ロジックを検証し、この探求はソーシャルメディア分析とアルゴリズム取引の交差点に関心を持つトレーダー、開発者、研究者に貴重な視点を提供します。
詳細
MetaTrader EAとPythonサーバーの両方のコードを分解し、それらの主要コンポーネントと機能を説明します。
MetaTrader 5 EA (MQL5)
1. 初期化と入力
EAはまず、取引銘柄、ツイッターAPI認証情報、ストップロスや利益確定レベルなどの取引パラメータの入力パラメータを定義します。また、取引執行とポジション管理に必要なライブラリも含まれています。
//+------------------------------------------------------------------+ //| Twitter_Sentiment_with_soquets.mq5 | //| Copyright 2024, Javier Santiago Gaston de Iriarte Cabrera. | //| https://www.mql5.com/ja/users/jsgaston/news | //+------------------------------------------------------------------+ #property copyright "Copyright 2024, Javier Santiago Gaston de Iriarte Cabrera." #property link "https://www.mql5.com/ja/users/jsgaston/news" #property version "1.001" #property description "This EA sends data to a Python server and receives sentiment analysis" input group "---- Symbol to work with ----" input string symbol1 = "BTCUSD"; // Symbol input group "---- Passwords ----" input string twitter_api_key = "TwitterApiKey"; // Twitter API key input string twitter_api_secret = "TwitterApiSecret"; // Twitter API secret input string twitter_access_token = "TwitterAccessToken"; // Twitter Access Token input string twitter_access_token_secret = "TwitterAccessTokenSecret"; // Twitter Access Token Secret input string twitter_bearer_token = "TwitterBearerToken"; // Twitter Bearer Token input string client_id = "TwitterClientID"; // Twitter Client ID input string client_secret = "TwitterClientSecret"; // Twitter Client Secret input group "---- Stops ----" input bool InpUseStops = false; // Use stops in trading input int InpTakeProfit = 1000; // TakeProfit level input int InpStopLoss = 500; // StopLoss level input double InpLot = 0.1; // Lot size #include <Trade\Trade.mqh> // Instatiate Trades Execution Library #include <Trade\OrderInfo.mqh> // Instatiate Library for Orders Information #include <Trade\PositionInfo.mqh> // Library for all position features and information // Create a trade object CTrade trade; // Last request time datetime lastRequestTime = 0; int requestInterval = 30 * 60; // 30 minutes in seconds
2. OnInit()関数
- プッシュ通知が有効かどうかをチェックします。
- 初期化中にPythonサーバーとの通信を開始します。
//+------------------------------------------------------------------+ //| Expert initialization function | //+------------------------------------------------------------------+ int OnInit() { if(!TerminalInfoInteger(TERMINAL_NOTIFICATIONS_ENABLED)) { Print("Push notifications are not enabled. Please enable them in terminal settings."); return INIT_FAILED; } // Call the function to communicate with the Python server during initialization string data = StringFormat("%s,%s,%s,%s,%s,%s,%s,%s", symbol1, twitter_api_key, twitter_api_secret, twitter_access_token, twitter_access_token_secret, twitter_bearer_token, client_id, client_secret); string result = CommunicateWithPython(data); Print("Result received from Python server during initialization: ", result); return(INIT_SUCCEEDED); }
3. OnTick()関数
- Pythonサーバーへのリクエスト間隔を30分にします。
- CommunicateWithPython()関数を使用して、データを形式し、Pythonサーバーに送信します。
- 受信したセンチメントデータを処理し、センチメントスコアに基づいて取引を実行します。
//+------------------------------------------------------------------+ //| Expert tick function | //+------------------------------------------------------------------+ void OnTick() { // Check if 30 minutes have passed since the last request if(TimeCurrent() - lastRequestTime < requestInterval) { return; // Exit if the interval has not passed } // Update the last request time lastRequestTime = TimeCurrent(); // Call the function to communicate with the Python server string data = StringFormat("%s,%s,%s,%s,%s,%s,%s,%s", symbol1, twitter_api_key, twitter_api_secret, twitter_access_token, twitter_access_token_secret, twitter_bearer_token, client_id, client_secret); string result = CommunicateWithPython(data); if(result == "") { Print("No data received from Python"); return; } // Process the sentiment value Print("Raw result: ", result); // Debug line string sentiment_values[]; int num_elements = StringSplit(result, ',', sentiment_values); Print("Number of elements: ", num_elements); // Debug line if(num_elements > 0) { double tweet_sentiment = StringToDouble(sentiment_values[0]); Print("Twitter sentiment: ", tweet_sentiment); // Debug line double price = SymbolInfoDouble(symbol1, SYMBOL_BID); double take_profit = InpTakeProfit * _Point; double stop_loss = InpStopLoss * _Point; if(tweet_sentiment > 0) { // Buy if sentiment is positive if(PositionSelect(symbol1)) { Print("Position already open. Skipping buy."); } else { if(trade.Buy(InpLot, symbol1, price, price - stop_loss, price + take_profit)) Print("Buying ", InpLot, " lots of ", symbol1); else Print("Failed to place buy order. Error: ", GetLastError()); } } else if(tweet_sentiment < 0) { // Sell if sentiment is negative if(PositionSelect(symbol1)) { Print("Position already open. Skipping sell."); } else { if(trade.Sell(InpLot, symbol1, price, price + stop_loss, price - take_profit)) Print("Selling ", InpLot, " lots of ", symbol1); else Print("Failed to place sell order. Error: ", GetLastError()); } } } else if(StringFind(result, "ERROR,") == 0) { Print("Error received from Python server: ", result); } else { Print("Unexpected response format: ", result); } }
4. ソケット通信
- InitSocket()関数はソケットを作成し、Pythonサーバーに接続します。
- CommunicateWithPython()関数は、Pythonサーバーへのデータ送信とPythonサーバーからのレスポンス受信を処理します。
//+------------------------------------------------------------------+ //| Initialize socket | //+------------------------------------------------------------------+ int InitSocket() { int socket_handle = SocketCreate(); if(socket_handle < 0) { Print("Error creating socket"); return -1; } Print("Socket created successfully."); // Connect to Python server bool isConnected = SocketConnect(socket_handle, "127.0.0.1", 65432, 5000); if(!isConnected) { int error = GetLastError(); Print("Error connecting to Python server. Error code: ", error); SocketClose(socket_handle); return -1; } Print("Connection to Python server established."); return socket_handle; } //+------------------------------------------------------------------+ //| Function to send and receive data | //+------------------------------------------------------------------+ string CommunicateWithPython(string data) { int socket_handle = InitSocket(); if(socket_handle < 0) return ""; // Ensure data is encoded in UTF-8 uchar send_buffer[]; StringToCharArray(data, send_buffer); int bytesSent = SocketSend(socket_handle, send_buffer, ArraySize(send_buffer)); if(bytesSent < 0) { Print("Error sending data!"); SocketClose(socket_handle); return ""; } Print("Data sent: ", bytesSent); uint timeout = 5000; // 5 seconds timeout uchar rsp[]; string result; uint timeout_check = GetTickCount() + timeout; do { uint len = SocketIsReadable(socket_handle); if(len) { int rsp_len; rsp_len = SocketRead(socket_handle, rsp, len, timeout); if(rsp_len > 0) { result += CharArrayToString(rsp, 0, rsp_len); } } } while(GetTickCount() < timeout_check && !IsStopped()); SocketClose(socket_handle); if(result == "") { Print("No data received from Python"); return ""; } Print("Data received from Python: ", result); return result; } //+------------------------------------------------------------------+ //| Helper function to convert uchar array to string | //+------------------------------------------------------------------+ string CharArrayToString(const uchar &arr[], int start, int length) { string result; char temp[]; ArrayResize(temp, length); ArrayCopy(temp, arr, 0, start, length); result = CharArrayToString(temp); return result; } //+------------------------------------------------------------------+
5. 取引ロジック
- センチメントがプラス(> 0)の場合、買いポジションを建てようとします。
- センチメントがマイナス(< 0)の場合、売りポジションを建てようとします。
- 取引執行にCTradeクラスを使用します。
Pythonサーバー
1. サーバーのセットアップ
- start_server()関数は、着信コネクションをリッスンするソケットサーバーを初期化します。
def start_server(): """Starts the server that waits for incoming connections.""" server_socket = socket.socket(socket.AF_INET, socket.SOCK_STREAM) server_socket.bind(('127.0.0.1', 65432)) server_socket.listen(1) print("Python server started and waiting for connections...") while True: client_socket, addr = server_socket.accept() print(f"Connection from {addr}") try: data = client_socket.recv(1024) data = data.decode('utf-8', errors='ignore') print(f"Received data: {data}") inputs = data.split(',') if len(inputs) != 8: raise ValueError("Eight inputs were expected") symbol, twitter_api_key, twitter_api_secret, twitter_access_token, twitter_access_token_secret, twitter_bearer_token, client_id, client_secret = inputs result = process_data(symbol, twitter_bearer_token) result_string = f"{result['tweet_sentiment']}" client_socket.sendall(result_string.encode('utf-8')) print(f"Response sent to client: {result_string}") except Exception as e: print(f"Communication error: {e}") error_message = f"ERROR,{str(e)}" client_socket.sendall(error_message.encode('utf-8')) finally: client_socket.shutdown(socket.SHUT_RDWR) client_socket.close() print("Connection closed")
2. データ処理
- コネクションを受信すると、データをデコードし、別々の入力に分割します。
- 銘柄とツイッターベアラートークンを指定してprocess_data()関数を呼び出します。
def process_data(symbol, bearer_token): """Processes the data obtained from news and tweets.""" result = { "tweet_sentiment": 0} try: result["tweet_sentiment"] = analyze_tweets(bearer_token, symbol) except Exception as e: raise Exception(f"Error processing data: {e}") print(f"Data processed. Result: {result}") return result
3. ツイート分析
- analyze_tweets()関数は、ツイッター APIを使用して、指定した銘柄に関する最近のツイートを取得します。
- TextBlobを使って各ツイートのセンチメント分析をおこないます。
- 検索されたすべてのツイートの平均センチメントスコアを計算します。
def analyze_tweets(bearer_token, symbol): """Analyzes recent tweets related to the given symbol.""" try: headers = { 'Authorization': f'Bearer {bearer_token}', } query = f"{symbol} lang:en -is:retweet" # Get the current time and subtract an hour end_time = datetime.now(timezone.utc) - timedelta(seconds=10) # Subtract 10 seconds from the current time start_time = end_time - timedelta(hours=4) # Convert to RFC 3339 (ISO 8601) format with second precision and 'Z' at the end start_time_str = start_time.strftime('%Y-%m-%dT%H:%M:%SZ') end_time_str = end_time.strftime('%Y-%m-%dT%H:%M:%SZ') search_url = f"https://api.twitter.com/2/tweets/search/recent?query={query}&max_results=100&start_time={start_time_str}&end_time={end_time_str}&sort_order=relevancy" print(f"Performing tweet search with query: {query}") print(f"Search URL: {search_url}") response = requests.get(search_url, headers=headers) print(f"Response status code: {response.status_code}") print(f"Response text: {response.text}") if response.status_code != 200: raise Exception(f"Error searching tweets: {response.status_code} - {response.text}") tweets = response.json().get('data', []) if not tweets: print("No tweets found") return 0 sentiments = [TextBlob(tweet['text']).sentiment.polarity for tweet in tweets] if not sentiments: print("No sentiments found") return 0 average_sentiment = sum(sentiments) / len(sentiments) return average_sentiment except Exception as e: print(f"Error: {e}") raise Exception(f"Error analyzing tweets: {e}")
4. エラー処理
- try-exceptブロックを実装し、データ処理やAPIリクエスト中の潜在的なエラーをキャッチして処理します。
5. 応答:
- 算出したセンチメントスコアをMT5クライアントに返送します。
重要なポイント
1. リアルタイム統合:このシステムは、30分ごとに最近のツイートを取得して分析することで、ほぼリアルタイムのセンチメント分析を提供します。
2. センチメントに基づく取引:EAはセンチメントスコアを取引判断に使用し、センチメントがプラスの場合は買いポジションを、マイナスの場合は売りポジションを建てます。
3. エラー処理:EAとPythonサーバーの両方が、APIリクエスト、データ処理、または通信に関する潜在的な問題を管理するためのエラー処理を実装しています。
4. スケーラビリティ:ソケットベースの通信により、MT5 EAを大幅に変更することなく、Pythonサーバーにデータソースを追加したり、より複雑な分析を追加したりといった拡張の可能性があります。
5. セキュリティへの配慮:システムは各リクエストでAPIクレデンシャルを渡します。本番環境では、これをよりセキュアにする必要があります。
6. 制約:現在の実装では、新しいポジションを開設するだけで、センチメントの変化に基づいて既存のポジションを管理することはありません。
このボットは、外部データ分析をMT5取引に統合する興味深いアプローチを示しています。しかし、本番の取引環境で使用するには、徹底的なテストと、場合によっては取引ロジックの改良が必要でしょう。
進め方
手順1:URLを挿入する(ツール→EA内)
手順2:Pythonサーバーの起動
Python server started and waiting for connections...
手順3:EAとの接続を開始し、データの受信を待つ
2024.07.24 23:29:45.087 Twitter_Sentiment_with_soquets_v4 (EURUSD,H1) Socket created successfully. 2024.07.24 23:29:45.090 Twitter_Sentiment_with_soquets_v4 (EURUSD,H1) Connection to Python server established. 2024.07.24 23:29:45.090 Twitter_Sentiment_with_soquets_v4 (EURUSD,H1) Data sent: 380
Data processed. Result: {'tweet_sentiment': 0.20970252525252508} Response sent to client: 0.20970252525252508 Connection closed
2024.07.24 23:29:50.082 Twitter_Sentiment_with_soquets_v4 (EURUSD,H1) Data received from Python: 0.20970252525252508 2024.07.24 23:29:50.082 Twitter_Sentiment_with_soquets_v4 (EURUSD,H1) Result received from Python server during initialization: 0.20970252525252508 2024.07.24 23:29:50.082 Twitter_Sentiment_with_soquets_v4 (EURUSD,H1) Socket created successfully. 2024.07.24 23:29:50.084 Twitter_Sentiment_with_soquets_v4 (EURUSD,H1) Connection to Python server established. 2024.07.24 23:29:50.084 Twitter_Sentiment_with_soquets_v4 (EURUSD,H1) Data sent: 380 2024.07.24 23:29:55.083 Twitter_Sentiment_with_soquets_v4 (EURUSD,H1) Data received from Python: 0.20970252525252508 2024.07.24 23:29:55.083 Twitter_Sentiment_with_soquets_v4 (EURUSD,H1) Raw result: 0.20970252525252508 2024.07.24 23:29:55.083 Twitter_Sentiment_with_soquets_v4 (EURUSD,H1) Number of elements: 1 2024.07.24 23:29:55.084 Twitter_Sentiment_with_soquets_v4 (EURUSD,H1) Twitter sentiment: 0.20970252525252508 2024.07.24 23:29:55.201 Twitter_Sentiment_with_soquets_v4 (EURUSD,H1) Buying 0.1 lots of EURUSD
良い結果を得るには?
より良い結果を得るために戦略とセンチメント分析を改善するために、以下の修正を検討します。
1. センチメント分析を洗練させる
- より高度なNLPテクニックを導入します。
* BERTやGPTのような事前に訓練された言語モデルを使用して、より微妙なセンチメント分析をおこないます。
* 資産の特定の属性に焦点を当てるために、アスペクトベースのセンチメント分析を組み込む。
- データソースを拡大します。
* 金融ニュース記事、SEC提出書類、その他の関連テキストソースを含みます。
* ツイッターだけでなく、複数のソーシャルメディアプラットフォームからデータを分析します。
- センチメント傾向分析を実施します。
* 絶対値だけでなく、時間の経過によるセンチメントの変化を追跡します。
* 短期的な変動を平準化するために、センチメントスコアの移動平均を使用します。
2. 取引ロジックを強化する
- センチメントの閾値を導入します。
* ロングポジションやショートポジションを建てるための特定のセンチメントレベルを定義します。
* エントリ時とエグジット時で異なる閾値を使用します。
- センチメントとテクニカル分析を組み合わせます
* テクニカル指標の確認ツールとしてセンチメントを利用します。
* 例えば、センチメントがポジティブで、価格が移動平均線より上にある場合のみ買います。
- 出来高分析を取り入れます
* ソーシャルメディアの投稿量とセンチメントを考慮します。
* 出来高が多く、センチメントが強ければ、より信頼性の高いシグナルとなります。
3. ポジションサイジングとリスク管理
- ダイナミックポジションサイジングを実施します
* センチメントシグナルの強さに基づいてポジションサイズを調整します。
* ポジションのサイズを決める際には、口座残高と市場全体のボラティリティを考慮します。
- 損切りと利食いのレベルにはセンチメントを利用します。
* センチメントのボラティリティに基づいて損切り水準を調整します。
* 潜在的なセンチメントの変化を考慮した利益目標を設定します。
4. 時間枠の考慮
- 複数の時間枠でセンチメントを分析します。
* 短期的なセンチメントによるエントリ/エグジットのタイミング
* 市場全体の方向性に関する長期的なセンチメントトレンド
- 時間ベースのフィルタを実装します。
* センチメントを分析し、取引をおこなう際には、曜日や時間帯を考慮します。
* 資産によっては、特定の市場時間帯により信頼性の高いセンチメントシグナルが出る場合があります。
5. 資産別チューニング
- 異なる資産クラス用に戦略をカスタマイズします。
* 暗号通貨は伝統的な株式とは異なるセンチメントに反応するかもしれません。
* 資産に特化したセンチメント辞書やモデルを開発します。
6. 機械学習の統合
- 値動きを予測する機械学習モデルを開発します。
* 従来の市場データと並んで、センチメントスコアを特徴として使用します。
* 継続的な戦略改善のために強化学習を導入します。
7. バックテストと最適化
- 広範なバックテストを実施します。
* 異なる市況と期間にわたって戦略をテストします。
* 過剰学習を避けるために、ウォークフォワード最適化を使用します。
- パラメータの最適化を実施します。
* 遺伝的アルゴリズムやその他の最適化技術を使用して、戦略パラメータを微調整します。
8. センチメントの検証
- センチメント分析の精度を検証するシステムを導入します。
* 分析したテキストのサンプルを定期的に手動でチェックします。
* センチメントスコアとその後の値動きとの相関を追跡します。
9. 適応戦略:
- 変化する市況に適応できるシステムを開発します。
* 市場全体のボラティリティに基づき、センチメントの閾値を調整します。
* 異なるサブストラテジーを切り替えるためのレジーム検出を実装します。
10. センチメント崩壊
- センチメントに時間減衰係数を導入します。
* 最近のセンチメントデータを重視します。
* 古いセンチメントスコアの影響を徐々に減らしていきます。
11. 逆張りアプローチ
- 特定の条件下では、逆張り戦略の実施を検討します。
* 一方向への極端なセンチメントは、反転の可能性を示すかもしれません。
このような修正は戦略を向上させる可能性がある一方で、複雑さを増すことも忘れてはなりません。それぞれの変更を徹底的にテストし、システム全体への影響を理解することが極めて重要です。簡単な修正から始め、各ステップを検証しながら徐々に複雑にしていきます。さらに、特に金融市場のダイナミックな世界では、過去の実績が将来の結果を保証するものではないことを常に意識してください。
また、ツィッターのツイートを取得するために、デベロッパーのアカウントにXを使っています。
結論
本稿で紹介した取引ボットは、ソーシャルメディアのセンチメント分析とアルゴリズム取引の統合における重要な前進を意味します。MetaTrader 5とPythonの力を活用することで、このシステムは金融市場におけるリアルタイムデータ駆動型意思決定の可能性を示しています。
ソケットベースのコミュニケーション、ツイッターデータのセンチメント分析、自動売買を組み合わせたこのボットの革新的なアプローチは、学際的な技術統合から生まれる可能性を示しています。現代の取引戦略における代替データソースの重要性の高まりや、金融の意思決定に貴重な洞察を提供する自然言語処理の可能性を強調しています。
しかし、どの取引システムにも改善と改良の余地があることは言うまでもありません。より洗練されたセンチメント分析手法の導入や機械学習モデルの適用など、戦略を強化するための提案は、将来の発展のためのロードマップを提供します。これらの改善により、より強固で信頼性の高い売買シグナル、優れたリスク管理、そして全体的なパフォーマンスの向上が期待できます。
このボットはセンチメントに基づく取引へのエキサイティングなアプローチを提示していますが、実取引環境に導入する前には徹底的なテストと検証が不可欠です。金融市場の複雑さ、急激なセンチメントの変化の可能性、アルゴリズム取引に内在するリスクを考慮すると、導入と最適化には慎重かつ理路整然としたアプローチが求められます。
自然言語処理、機械学習、アルゴリズム取引の分野が進化し続ける中で、このボットのようなシステムは金融業界においてますます重要な役割を果たすでしょう。トレーダーにとって新しいツールであるだけでなく、金融市場をどのように理解し、対話するかという新しいパラダイムを象徴しています。
この最初の実装から、完全に最適化され市場に投入できるシステムへの道のりは、継続的な学習、テスト、改良の連続です。限界を常に押し広げ、新たな洞察を求めつつ、変化し続ける市場環境の中でより多くの情報に基づいた意思決定を行うために努力していきます。
MetaQuotes Ltdにより英語から翻訳されました。
元の記事: https://www.mql5.com/en/articles/15407





- 無料取引アプリ
- 8千を超えるシグナルをコピー
- 金融ニュースで金融マーケットを探索