Lorentzos Roussos / Profil
- Information
11+ Jahre
Erfahrung
|
11
Produkte
|
289
Demoversionen
|
218
Jobs
|
0
Signale
|
0
Abonnenten
|
💎 The simplest Neural Network Coding Guide : https://www.mql5.com/en/blogs/post/752324
💎 The simplest DLL for mql5 Coding Guide : https://www.mql5.com/en/blogs/post/753426
💎 Harmonic patterns scanner for MT5 : https://www.mql5.com/en/market/product/51212
💎 Similar price action candlestick patterns indicator for MT5 : https://www.mql5.com/en/market/product/133318
💎 Fibonacci Sonar indicator : https://www.mql5.com/en/market/product/133627
💎 Chart projection indicator : https://www.mql5.com/en/market/product/5569
💎 Youtube : https://www.youtube.com/@lorentzor1938
Qualität der Leistungsbeschreibung | 5.0 | |
Ergebnis der Qualitätsprüfung | 5.0 | |
Erreichbarkeit und Kommunikation | 5.0 |

How many click download thinking its for their mobile mt5?


☕️



Like forex factory it appears to be hostile toward sellers .




Der Flower-Indikator ist ein technisches Analysetool, das hauptsächlich zur Trenderkennung und zur Unterstützung von Scalping- oder Intraday-Handelsstrategien eingesetzt wird. Der Flower-Indikator ist ein Signaltrendindikator, der auf technischen Analysen und Preisdynamikanalysen basiert. Er visualisiert Trends mithilfe farbiger Blumen – blaue Blumen signalisieren einen Aufwärtstrend, rote Blumen einen Abwärtstrend. Jedes Level kann als Unterstützung und Widerstand fungieren (achten Sie auf +/-

Der Flower-Indikator ist ein technisches Analysetool, das hauptsächlich zur Trenderkennung und zur Unterstützung von Scalping- oder Intraday-Handelsstrategien eingesetzt wird. Der Flower-Indikator ist ein Signaltrendindikator, der auf technischen Analysen und Preisdynamikanalysen basiert. Er visualisiert Trends mithilfe farbiger Blumen – blaue Blumen signalisieren einen Aufwärtstrend, rote Blumen einen Abwärtstrend. Jedes Level kann als Unterstützung und Widerstand fungieren (achten Sie auf +/-

✔️ Expert Advisor
✔️ MT4
✔️ MT5
✔️ MultiSymbol
✔️ MultiTimeframe
✔️ AutoTrades
✔️ Control From One Chart
✔️ Never Repaints
Instant demo downloads ,wont need to register anything ,the demos have a nag screen on start for about 30seconds ,then it disappears :
◼️ Download Free Demo For MT4 : https://c.mql5.com/6/908/Voenix_Demo_MT4.ex4
◼️ Download Free Demo For MT5 : https://c.mql5.com/6/908/Voenix_Demo_MT5.ex5
💎 More Info on this short video (dont need to have sound on its quitte visual) : https://www.youtube.com/watch?v=jJ8kzL5Wusk
Harmonische muster EA Wenn Sie dies lieber in einem Live-Chart sehen möchten, anstatt die Beschreibung zu lesen, können Sie hier sofort eine kostenlose Demo herunterladen. Enthaltene Muster: ABCD-Muster Gartley-Muster Fledermausmuster Cypher-Muster 3Drives-Muster Schwarzer Schwan-Muster Weißes Schwanenmuster Quasimodo-Muster oder Über-Unter-Muster Alternatives Bat-Muster Schmetterlingsmuster Tiefes Krabbenmuster Krabbenmuster Hai-Muster FiveO-Muster Kopf- und Schultermuster Aufsteigendes
Qualität der Leistungsbeschreibung | 5.0 | |
Ergebnis der Qualitätsprüfung | 5.0 | |
Erreichbarkeit und Kommunikation | 5.0 |

⏰ If you dont wan't to read all that (video you can watch on mute) : https://www.youtube.com/watch?v=Oaly8VQP7to
Includes : ✔️ TPOS (minus the characters display mode)
✔️ Vwap (volume weighted average price) calculation.
✔️ POC Point Of Control
✔️ Value Area
✔️ Developing POC
✔️ Developing VWAP
✔️ Bar Dynamics
✔️ Crosshair
✔️ Hover Fx to assist focus on data points
The indicator is not periodic or overall (on the chart) rather the user can define the start and end of the calculation range on the chart manually.
Many Instances of the indicator can coexist on a chart
You can download an instant 100% free demo ,with a 30 second nag screen on start, at the following link
◼️ Instant Free Demo : https://c.mql5.com/6/888/ForexMarketProfile_Demo.ex4
📖 Manual : https://www.mql5.com/en/blogs/post/744395
📢 Subscribe to Telegram Channel for more : https://t.me/lorentzor
Forex Market Profile (kurz FMP) Was das nicht ist: FMP ist nicht die klassische buchstabencodierte TPO-Anzeige , zeigt nicht die Berechnung des gesamten Diagrammdatenprofils an und segmentiert das Diagramm nicht in Perioden und berechnet diese nicht. Was es macht : Am wichtigsten ist, dass der FMP-Indikator Daten verarbeitet, die sich zwischen dem linken Rand des benutzerdefinierten Spektrums und dem rechten Rand des benutzerdefinierten Spektrums befinden. Der Benutzer kann das Spektrum



◼️ Short Video Presentation with no sound (if you dont want to read all that) :
https://www.youtube.com/watch?v=jJ8kzL5Wusk
Instant demo downloads ,wont need to register anything ,the demos have a nag screen on start for about 30seconds ,then it disappears :
◼️ Download Free Demo For MT4 : https://c.mql5.com/6/908/Voenix_Demo_MT4.ex4
◼️ Download Free Demo For MT5 : https://c.mql5.com/6/908/Voenix_Demo_MT5.ex5
📢 Subscribe to Telegram Channel for more : https://t.me/lorentzor
The scanner contains the following patterns :
✔️ ABCD pattern
✔️ Gartley pattern
✔️ Bat pattern
✔️ Cypher pattern
✔️ 3Drives pattern
✔️ Black Swan pattern
✔️ White Swan pattern
✔️ Quasimodo pattern or Over Under pattern
✔️ Alt Bat pattern
✔️ Butterfly pattern
✔️ Deep Crab pattern
✔️ Crab pattern
✔️ Shark pattern
✔️ FiveO pattern
✔️ Head And Shoulders pattern
✔️ Ascending Triangle pattern
✔️ One Two Three pattern
and 8 custom patterns .
✔️ Expert Advisor
✔️ MT4
✔️ MT5
✔️ MultiSymbol
✔️ MultiTimeframe
✔️ AutoTrades
✔️ Control From One Chart
✔️ Never Repaints
Harmonische Muster , scanner und Trader Enthaltene Muster: ABCD-Muster Gartley-Muster Fledermausmuster Cypher-Muster 3Drives-Muster Schwarzer Schwan-Muster Weißes Schwanenmuster Quasimodo-Muster oder Über-Unter-Muster Alternatives Bat-Muster Schmetterlingsmuster Tiefes Krabbenmuster Krabbenmuster Hai-Muster FiveO-Muster Kopf- und Schultermuster Aufsteigendes Dreiecksmuster Eins-zwei-drei-Muster Und 8 benutzerdefinierte Muster Voenix ist ein Scanner für harmonische Muster mit mehreren Zeitrahmen




https://c.mql5.com/6/966/Similar_Price_Action_MQL4_Demo__1.ex4
💎 Similar price action MT5 free download for EURUSD :
https://c.mql5.com/6/966/Similar_Price_Action_MQL_Demo__1.ex5
💎 Similar price action basic showcase :
https://www.youtube.com/watch?v=Shzdwm_UUTo
☕️
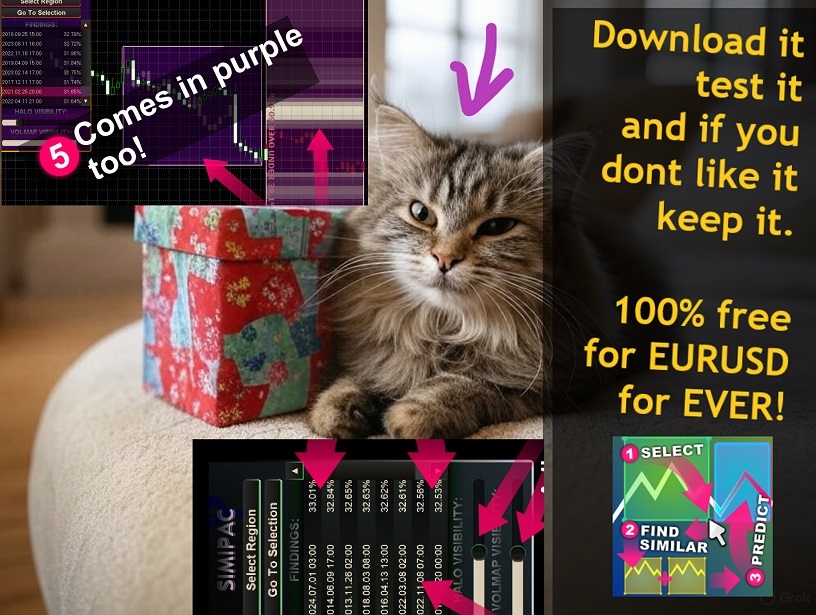

Fibonacci-Sonar Das Fibonacci-Sonar verfügt über eine interne Bibliothek mit Preisaktionsmustern, die aus 28 Symbolen und 7 Zeitrahmen gesammelt wurden. Jedes Muster ist in zwei Seiten unterteilt: die Vorher- und die Nachherseite. Das Fibonacci-Sonar verwendet die Vorherseite, um ähnliche Muster zu erkennen. Die jüngste Preisaktion (zu jedem Zeitpunkt) bis zum Eröffnungspreis des neuesten Balkens wird als Vorherseite zum Durchsuchen der Bibliothek verwendet. Die Nachherseite enthält eine

💎 Similar price action candlestick patterns indicator for MT4 : https://www.mql5.com/en/market/product/133347
https://www.youtube.com/watch?v=Shzdwm_UUTo
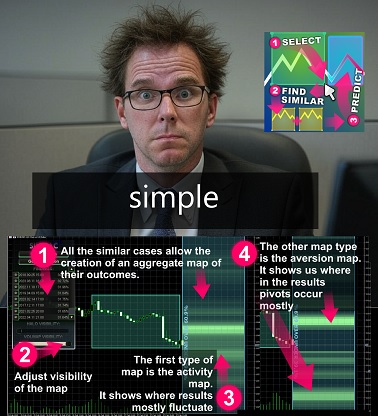



Indikator für ähnliche Preisaktionen „Ähnliche Preisaktionen“ findet vergangene Sequenzen von Kerzenmustern, die einem von Ihnen ausgewählten Bereich des Charts so ähnlich wie möglich sind. Der Indikator führt eine Suche durch, die direkt vor der ersten Kerze des von Ihnen ausgewählten Preisaktionsbereichs beginnt und bis zum allerersten ältesten Balken des Charts reicht. Es gibt mehrere Kriterien zur Bewertung der Ähnlichkeit zwischen zwei Sequenzen: Sequenzen müssen identisch lang sein. Wenn


Der Flower-Indikator ist ein technisches Analysetool, das hauptsächlich zur Trenderkennung und zur Unterstützung von Scalping- oder Intraday-Handelsstrategien eingesetzt wird. Der Flower-Indikator ist ein Signaltrendindikator, der auf technischen Analysen und Preisdynamikanalysen basiert. Er visualisiert Trends mithilfe farbiger Blumen – blaue Blumen signalisieren einen Aufwärtstrend, rote Blumen einen Abwärtstrend. Jedes Level kann als Unterstützung und Widerstand fungieren (achten Sie auf +/-
Der Flower-Indikator ist ein technisches Analysetool, das hauptsächlich zur Trenderkennung und zur Unterstützung von Scalping- oder Intraday-Handelsstrategien eingesetzt wird. Der Flower-Indikator ist ein Signaltrendindikator, der auf technischen Analysen und Preisdynamikanalysen basiert. Er visualisiert Trends mithilfe farbiger Blumen – blaue Blumen signalisieren einen Aufwärtstrend, rote Blumen einen Abwärtstrend. Jedes Level kann als Unterstützung und Widerstand fungieren (achten Sie auf +/-