'NeuroNet.cl' as 'const string cl_program' NeuroNet.cl 1 1
Does not compile.
Another question. Can I try running it without using old data? And in what order to run it?
Where to find the file #include "legendre.mqh"?
star-ik #:
Where to find the #include "legendre.mqh" file?
Where to find the #include "legendre.mqh" file?
The specified library was used in FEDformer. For the purposes of this article, the line can be simply deleted.
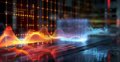
Нейросети — это просто (Часть 89): Трансформер частотного разложения сигнала (FEDformer)
- www.mql5.com
Все рассмотренные нами ранее модели анализируют состояние окружающей среды в виде временной последовательности. Однако, тот же временной ряд можно представить и в виде частотных характеристик. В данной статье я предлагаю вам познакомиться с алгоритмом, который использует частотные характеристики временной последовательности для прогнозирования будущих состояний.

You are missing trading opportunities:
- Free trading apps
- Over 8,000 signals for copying
- Economic news for exploring financial markets
Registration
Log in
You agree to website policy and terms of use
If you do not have an account, please register
Check out the new article: Neural Networks Made Easy (Part 94): Optimizing the Input Sequence.
When working with time series, we always use the source data in their historical sequence. But is this the best option? There is an opinion that changing the sequence of the input data will improve the efficiency of the trained models. In this article I invite you to get acquainted with one of the methods for optimizing the input sequence.
When used in Transformer architecture models, the effectiveness of long-term dependency detection is highly dependent on many factors. These include sequence length, various positional encoding strategies, and data tokenization.
Such thoughts led the authors of the paper "Segment, Shuffle, and Stitch: A Simple Mechanism for Improving Time-Series Representations" to the idea of finding the optimal use of historical sequence. Could there be a better organization of time series that would allow for more efficient representation learning given the task at hand?
In the article, the authors present a simple and ready-to-use mechanism called Segment, Shuffle, Stitch (S3), designed to learn how to optimize the representation of time series. As the name suggests, S3 works by segmenting a time series into multiple non-overlapping segments, shuffling these segments into the most optimal order, and then combining the shuffled segments into a new sequence. It should be noted here that the order of segment shuffling is learned for each specific task.
Author: Dmitriy Gizlyk