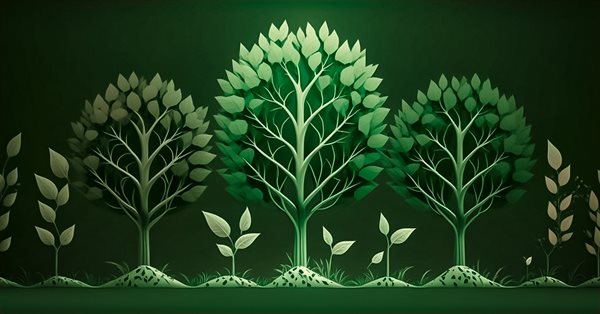
Population optimization algorithms: Saplings Sowing and Growing up (SSG)
Saplings Sowing and Growing up (SSG) algorithm is inspired by one of the most resilient organisms on the planet demonstrating outstanding capability for survival in a wide variety of conditions.
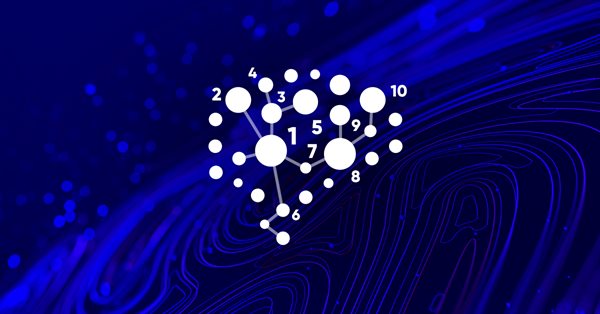
Experiments with neural networks (Part 4): Templates
In this article, I will use experimentation and non-standard approaches to develop a profitable trading system and check whether neural networks can be of any help for traders. MetaTrader 5 as a self-sufficient tool for using neural networks in trading. Simple explanation.
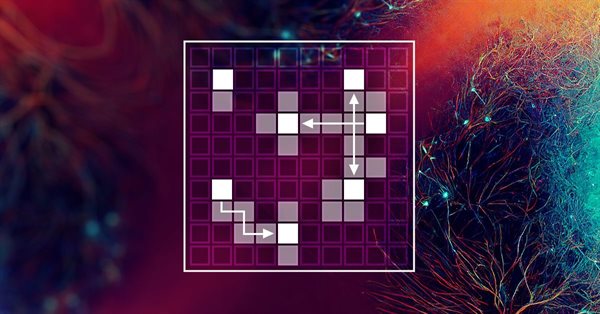
Neural networks made easy (Part 36): Relational Reinforcement Learning
In the reinforcement learning models we discussed in previous article, we used various variants of convolutional networks that are able to identify various objects in the original data. The main advantage of convolutional networks is the ability to identify objects regardless of their location. At the same time, convolutional networks do not always perform well when there are various deformations of objects and noise. These are the issues which the relational model can solve.
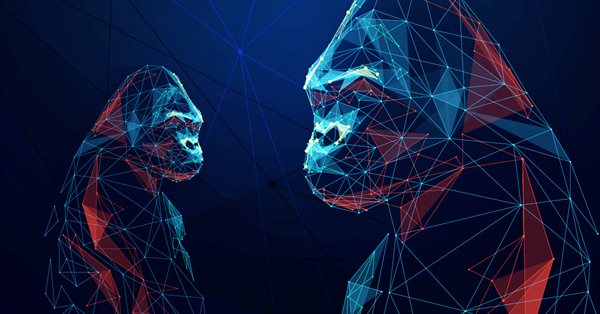
Population optimization algorithms: Monkey algorithm (MA)
In this article, I will consider the Monkey Algorithm (MA) optimization algorithm. The ability of these animals to overcome difficult obstacles and get to the most inaccessible tree tops formed the basis of the idea of the MA algorithm.
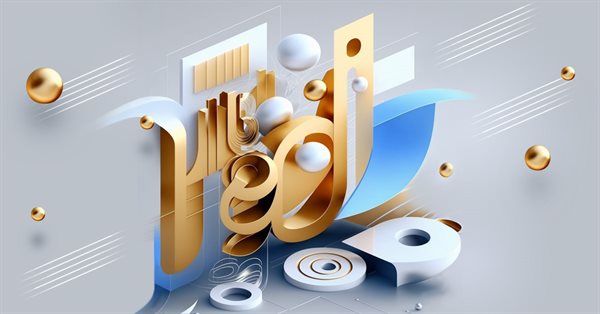
Population optimization algorithms: Harmony Search (HS)
In the current article, I will study and test the most powerful optimization algorithm - harmonic search (HS) inspired by the process of finding the perfect sound harmony. So what algorithm is now the leader in our rating?
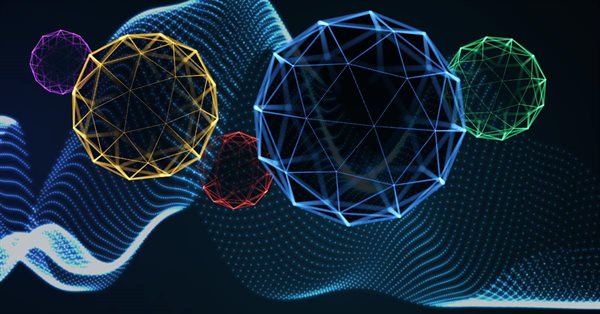
An example of how to ensemble ONNX models in MQL5
ONNX (Open Neural Network eXchange) is an open format built to represent neural networks. In this article, we will show how to use two ONNX models in one Expert Advisor simultaneously.
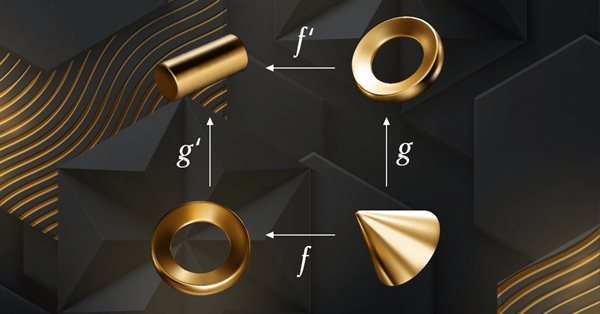
Category Theory in MQL5 (Part 6): Monomorphic Pull-Backs and Epimorphic Push-Outs
Category Theory is a diverse and expanding branch of Mathematics which is only recently getting some coverage in the MQL5 community. These series of articles look to explore and examine some of its concepts & axioms with the overall goal of establishing an open library that provides insight while also hopefully furthering the use of this remarkable field in Traders' strategy development.
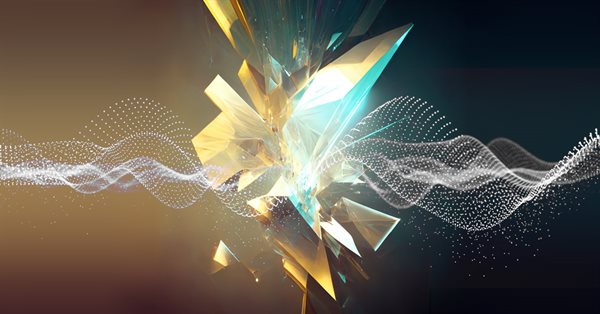
Backpropagation Neural Networks using MQL5 Matrices
The article describes the theory and practice of applying the backpropagation algorithm in MQL5 using matrices. It provides ready-made classes along with script, indicator and Expert Advisor examples.
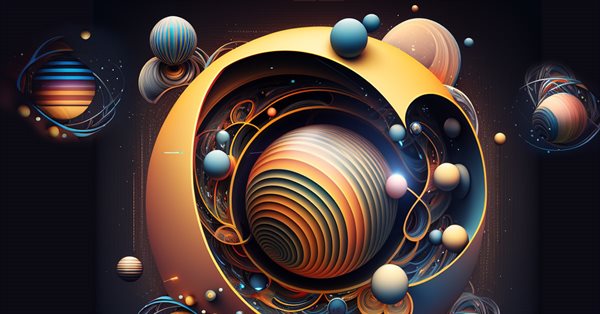
Population optimization algorithms: Gravitational Search Algorithm (GSA)
GSA is a population optimization algorithm inspired by inanimate nature. Thanks to Newton's law of gravity implemented in the algorithm, the high reliability of modeling the interaction of physical bodies allows us to observe the enchanting dance of planetary systems and galactic clusters. In this article, I will consider one of the most interesting and original optimization algorithms. The simulator of the space objects movement is provided as well.
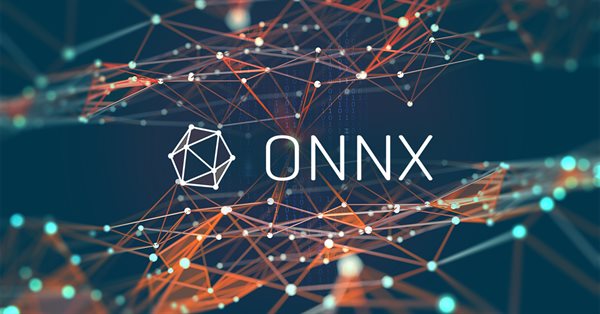
How to use ONNX models in MQL5
ONNX (Open Neural Network Exchange) is an open format built to represent machine learning models. In this article, we will consider how to create a CNN-LSTM model to forecast financial timeseries. We will also show how to use the created ONNX model in an MQL5 Expert Advisor.
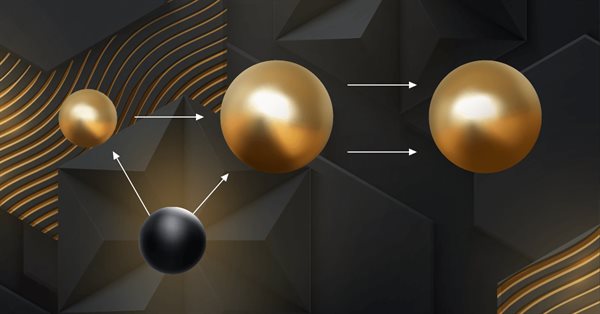
Category Theory in MQL5 (Part 5): Equalizers
Category Theory is a diverse and expanding branch of Mathematics which is only recently getting some coverage in the MQL5 community. These series of articles look to explore and examine some of its concepts & axioms with the overall goal of establishing an open library that provides insight while also hopefully furthering the use of this remarkable field in Traders' strategy development.
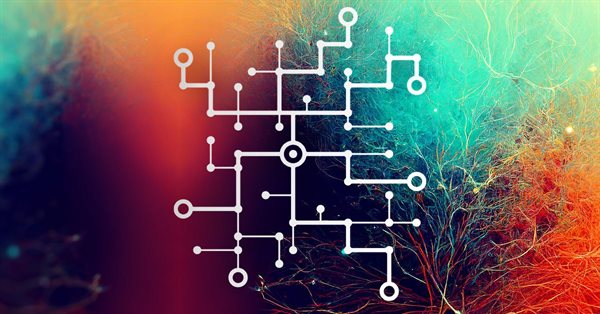
Neural networks made easy (Part 35): Intrinsic Curiosity Module
We continue to study reinforcement learning algorithms. All the algorithms we have considered so far required the creation of a reward policy to enable the agent to evaluate each of its actions at each transition from one system state to another. However, this approach is rather artificial. In practice, there is some time lag between an action and a reward. In this article, we will get acquainted with a model training algorithm which can work with various time delays from the action to the reward.
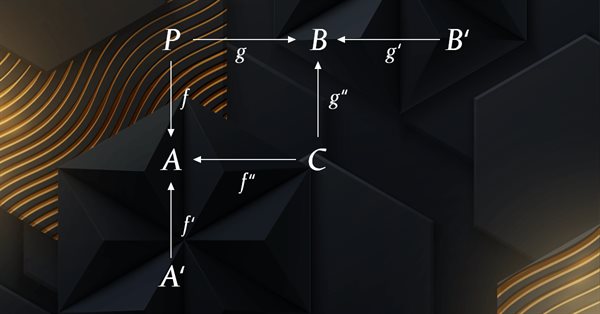
Category Theory in MQL5 (Part 4): Spans, Experiments, and Compositions
Category Theory is a diverse and expanding branch of Mathematics which as of yet is relatively uncovered in the MQL5 community. These series of articles look to introduce and examine some of its concepts with the overall goal of establishing an open library that provides insight while hopefully furthering the use of this remarkable field in Traders' strategy development.
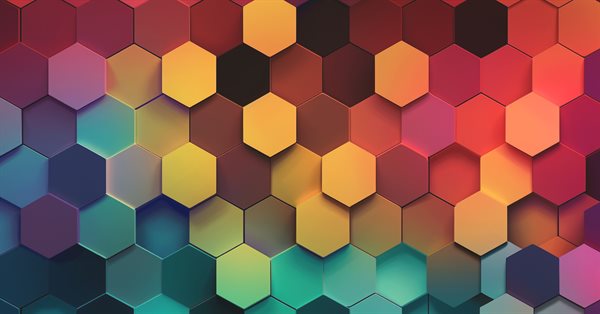
Data Science and Machine Learning(Part 14): Finding Your Way in the Markets with Kohonen Maps
Are you looking for a cutting-edge approach to trading that can help you navigate complex and ever-changing markets? Look no further than Kohonen maps, an innovative form of artificial neural networks that can help you uncover hidden patterns and trends in market data. In this article, we'll explore how Kohonen maps work, and how they can be used to develop smarter, more effective trading strategies. Whether you're a seasoned trader or just starting out, you won't want to miss this exciting new approach to trading.
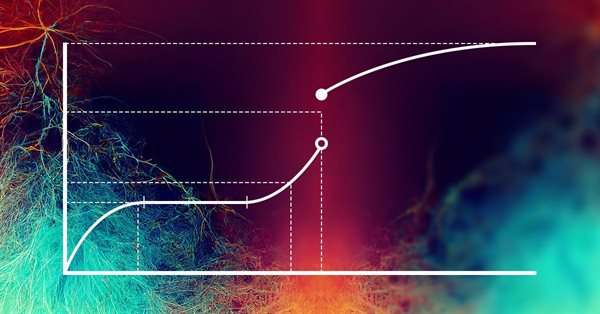
Neural networks made easy (Part 34): Fully Parameterized Quantile Function
We continue studying distributed Q-learning algorithms. In previous articles, we have considered distributed and quantile Q-learning algorithms. In the first algorithm, we trained the probabilities of given ranges of values. In the second algorithm, we trained ranges with a given probability. In both of them, we used a priori knowledge of one distribution and trained another one. In this article, we will consider an algorithm which allows the model to train for both distributions.
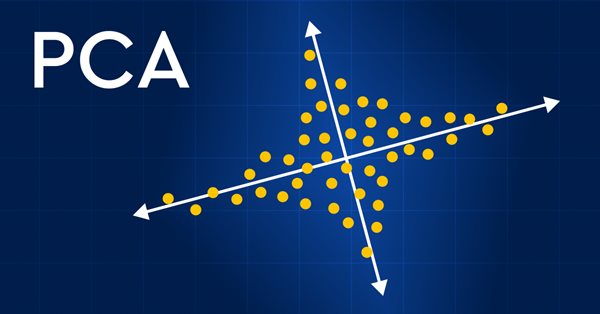
Data Science and Machine Learning (Part 13): Improve your financial market analysis with Principal Component Analysis (PCA)
Revolutionize your financial market analysis with Principal Component Analysis (PCA)! Discover how this powerful technique can unlock hidden patterns in your data, uncover latent market trends, and optimize your investment strategies. In this article, we explore how PCA can provide a new lens for analyzing complex financial data, revealing insights that would be missed by traditional approaches. Find out how applying PCA to financial market data can give you a competitive edge and help you stay ahead of the curve
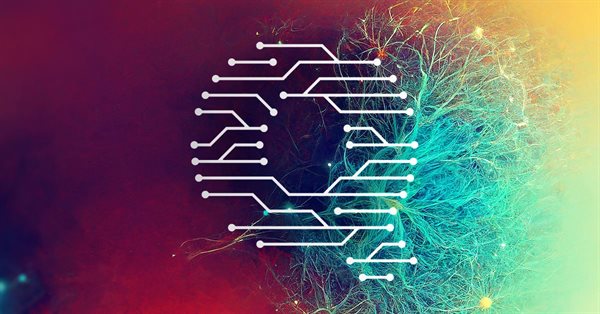
Neural networks made easy (Part 33): Quantile regression in distributed Q-learning
We continue studying distributed Q-learning. Today we will look at this approach from the other side. We will consider the possibility of using quantile regression to solve price prediction tasks.
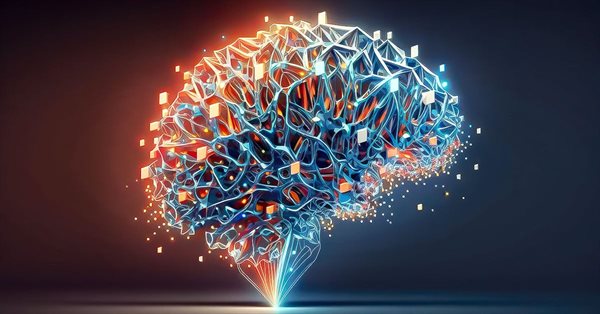
Data Science and Machine Learning (Part 12): Can Self-Training Neural Networks Help You Outsmart the Stock Market?
Are you tired of constantly trying to predict the stock market? Do you wish you had a crystal ball to help you make more informed investment decisions? Self-trained neural networks might be the solution you've been looking for. In this article, we explore whether these powerful algorithms can help you "ride the wave" and outsmart the stock market. By analyzing vast amounts of data and identifying patterns, self-trained neural networks can make predictions that are often more accurate than human traders. Discover how you can use this cutting-edge technology to maximize your profits and make smarter investment decisions.
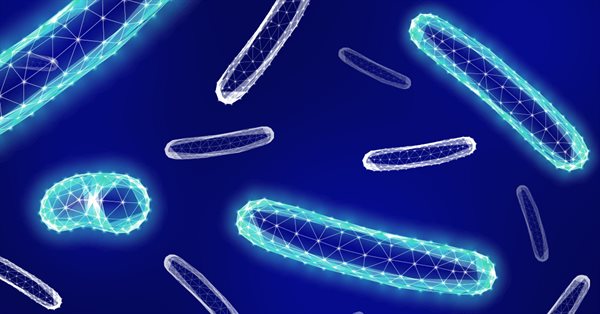
Population optimization algorithms: Bacterial Foraging Optimization (BFO)
E. coli bacterium foraging strategy inspired scientists to create the BFO optimization algorithm. The algorithm contains original ideas and promising approaches to optimization and is worthy of further study.
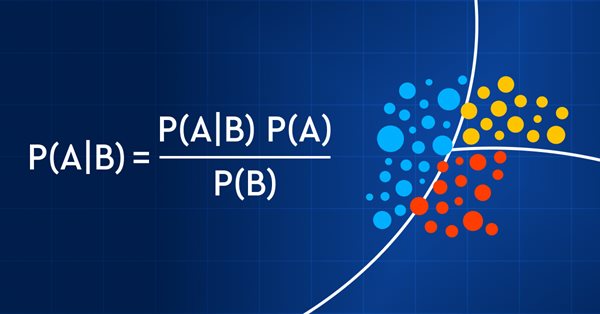
Data Science and Machine Learning (Part 11): Naïve Bayes, Probability theory in Trading
Trading with probability is like walking on a tightrope - it requires precision, balance, and a keen understanding of risk. In the world of trading, the probability is everything. It's the difference between success and failure, profit and loss. By leveraging the power of probability, traders can make informed decisions, manage risk effectively, and achieve their financial goals. So, whether you're a seasoned investor or a novice trader, understanding probability is the key to unlocking your trading potential. In this article, we'll explore the exciting world of trading with probability and show you how to take your trading game to the next level.
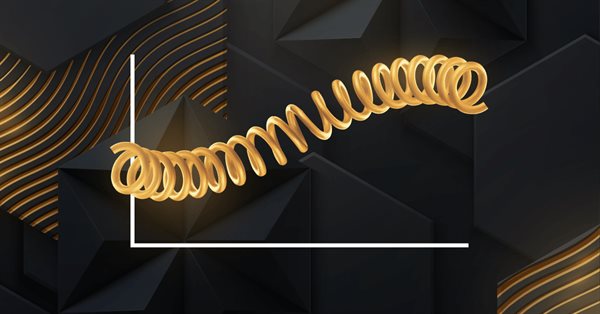
Category Theory in MQL5 (Part 3)
Category Theory is a diverse and expanding branch of Mathematics which as of yet is relatively uncovered in the MQL5 community. These series of articles look to introduce and examine some of its concepts with the overall goal of establishing an open library that provides insight while hopefully furthering the use of this remarkable field in Traders' strategy development.
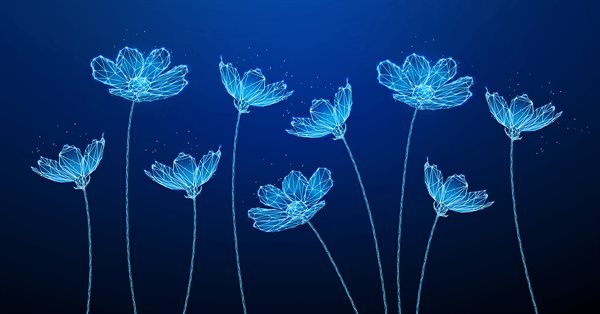
Population optimization algorithms: Invasive Weed Optimization (IWO)
The amazing ability of weeds to survive in a wide variety of conditions has become the idea for a powerful optimization algorithm. IWO is one of the best algorithms among the previously reviewed ones.
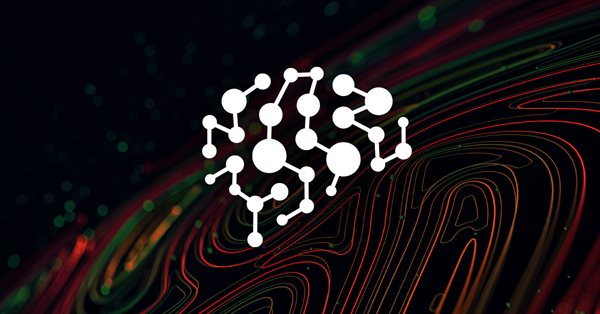
Experiments with neural networks (Part 3): Practical application
In this article series, I use experimentation and non-standard approaches to develop a profitable trading system and check whether neural networks can be of any help for traders. MetaTrader 5 is approached as a self-sufficient tool for using neural networks in trading.
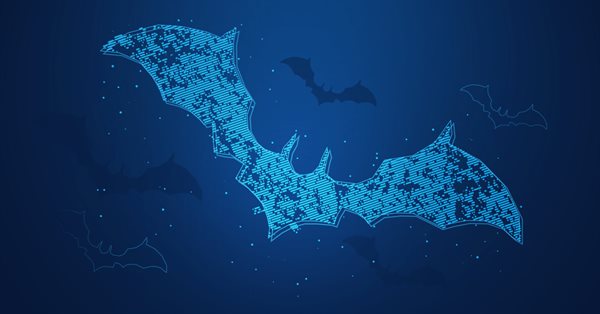
Population optimization algorithms: Bat algorithm (BA)
In this article, I will consider the Bat Algorithm (BA), which shows good convergence on smooth functions.
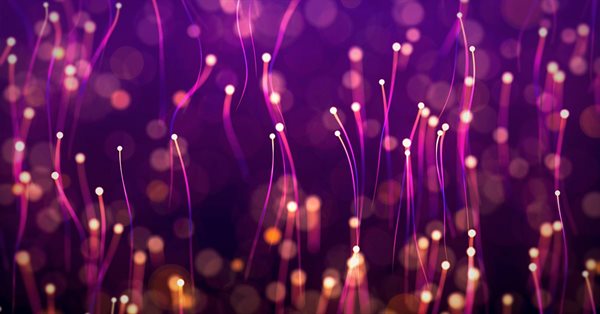
Population optimization algorithms: Firefly Algorithm (FA)
In this article, I will consider the Firefly Algorithm (FA) optimization method. Thanks to the modification, the algorithm has turned from an outsider into a real rating table leader.
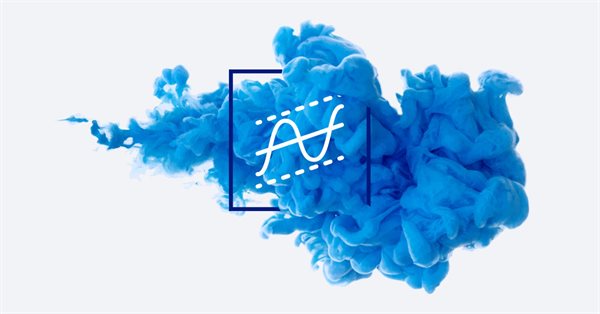
Measuring Indicator Information
Machine learning has become a popular method for strategy development. Whilst there has been more emphasis on maximizing profitability and prediction accuracy , the importance of processing the data used to build predictive models has not received a lot of attention. In this article we consider using the concept of entropy to evaluate the appropriateness of indicators to be used in predictive model building as documented in the book Testing and Tuning Market Trading Systems by Timothy Masters.
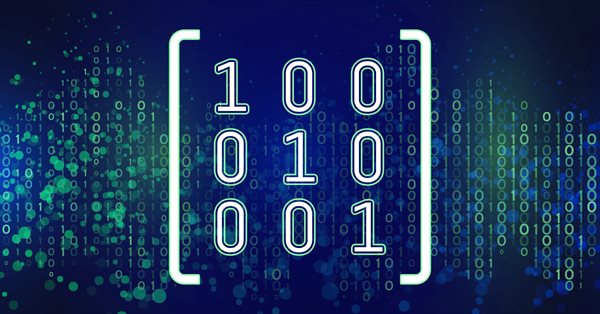
Matrix Utils, Extending the Matrices and Vector Standard Library Functionality
Matrix serves as the foundation of machine learning algorithms and computers in general because of their ability to effectively handle large mathematical operations, The Standard library has everything one needs but let's see how we can extend it by introducing several functions in the utils file, that are not yet available in the library
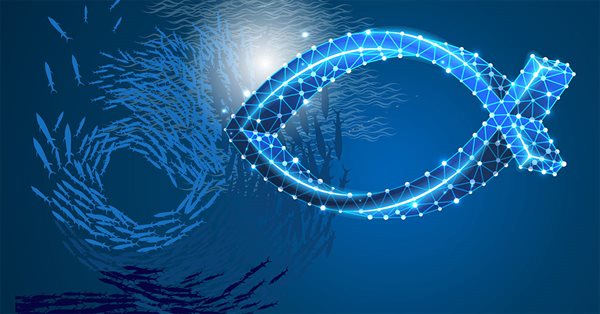
Population optimization algorithms: Fish School Search (FSS)
Fish School Search (FSS) is a new optimization algorithm inspired by the behavior of fish in a school, most of which (up to 80%) swim in an organized community of relatives. It has been proven that fish aggregations play an important role in the efficiency of foraging and protection from predators.
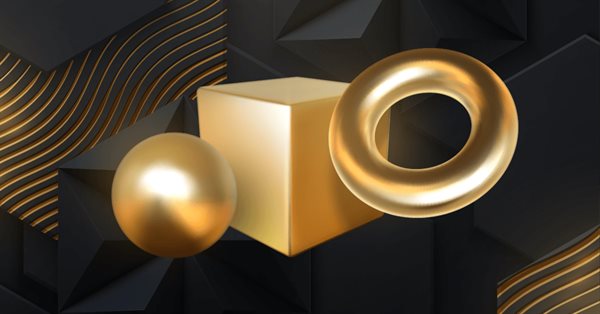
Category Theory in MQL5 (Part 2)
Category Theory is a diverse and expanding branch of Mathematics which as of yet is relatively uncovered in the MQL5 community. These series of articles look to introduce and examine some of its concepts with the overall goal of establishing an open library that attracts comments and discussion while hopefully furthering the use of this remarkable field in Traders' strategy development.
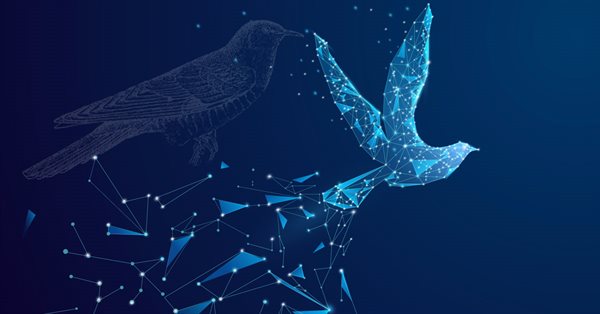
Population optimization algorithms: Cuckoo Optimization Algorithm (COA)
The next algorithm I will consider is cuckoo search optimization using Levy flights. This is one of the latest optimization algorithms and a new leader in the leaderboard.
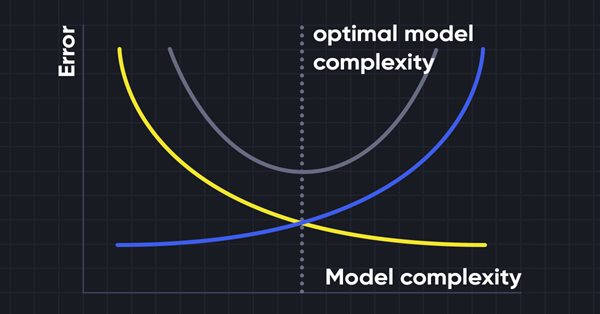
Data Science and Machine Learning (Part 10): Ridge Regression
Ridge regression is a simple technique to reduce model complexity and prevent over-fitting which may result from simple linear regression
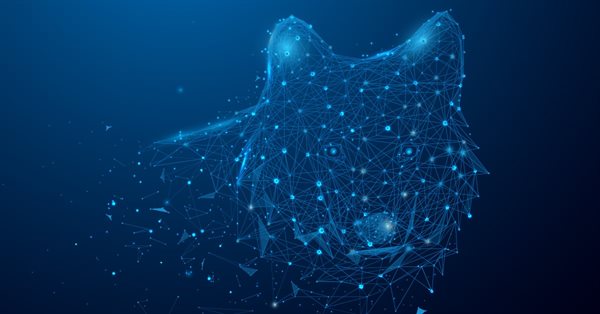
Population optimization algorithms: Grey Wolf Optimizer (GWO)
Let's consider one of the newest modern optimization algorithms - Grey Wolf Optimization. The original behavior on test functions makes this algorithm one of the most interesting among the ones considered earlier. This is one of the top algorithms for use in training neural networks, smooth functions with many variables.
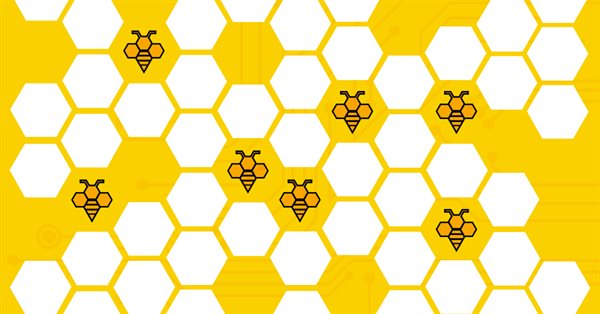
Population optimization algorithms: Artificial Bee Colony (ABC)
In this article, we will study the algorithm of an artificial bee colony and supplement our knowledge with new principles of studying functional spaces. In this article, I will showcase my interpretation of the classic version of the algorithm.
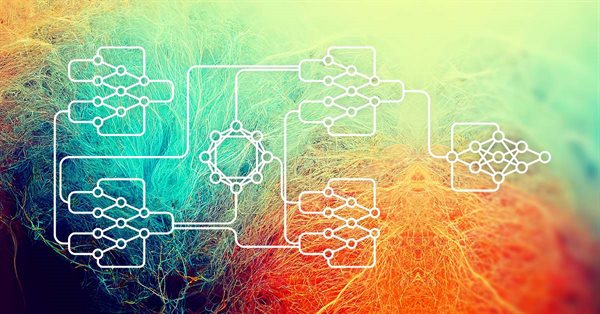
Neural networks made easy (Part 32): Distributed Q-Learning
We got acquainted with the Q-learning method in one of the earlier articles within this series. This method averages rewards for each action. Two works were presented in 2017, which show greater success when studying the reward distribution function. Let's consider the possibility of using such technology to solve our problems.
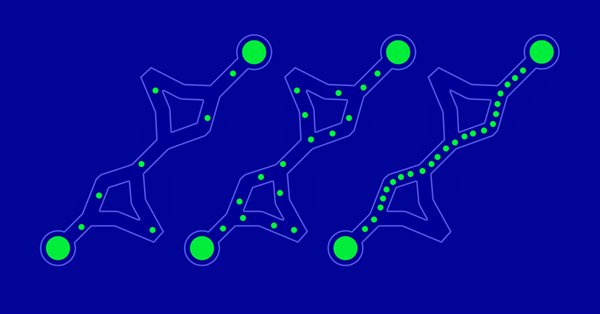
Population optimization algorithms: Ant Colony Optimization (ACO)
This time I will analyze the Ant Colony optimization algorithm. The algorithm is very interesting and complex. In the article, I make an attempt to create a new type of ACO.
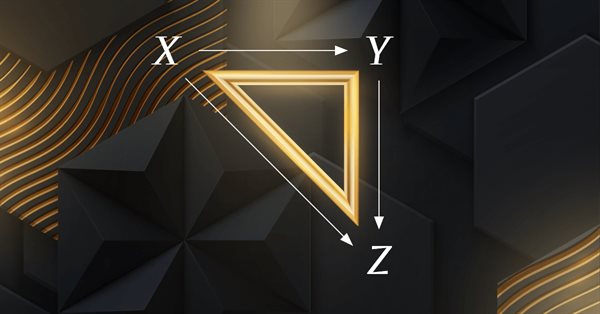
Category Theory in MQL5 (Part 1)
Category Theory is a diverse and expanding branch of Mathematics which as of yet is relatively uncovered in the MQL community. These series of articles look to introduce and examine some of its concepts with the overall goal of establishing an open library that attracts comments and discussion while hopefully furthering the use of this remarkable field in Traders' strategy development.
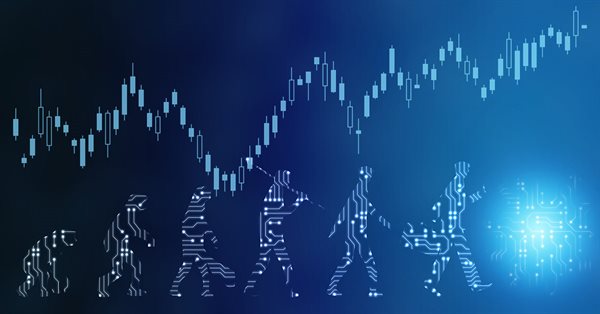
Neural networks made easy (Part 31): Evolutionary algorithms
In the previous article, we started exploring non-gradient optimization methods. We got acquainted with the genetic algorithm. Today, we will continue this topic and will consider another class of evolutionary algorithms.
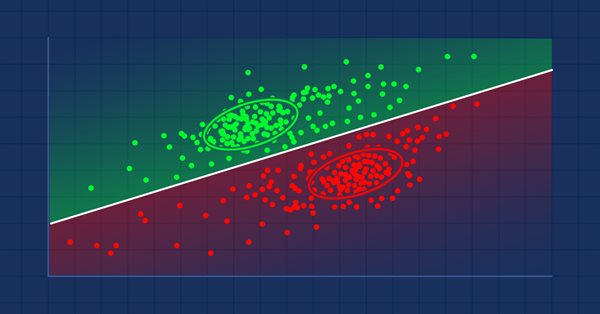
MQL5 Wizard techniques you should know (Part 04): Linear Discriminant Analysis
Todays trader is a philomath who is almost always looking up new ideas, trying them out, choosing to modify them or discard them; an exploratory process that should cost a fair amount of diligence. These series of articles will proposition that the MQL5 wizard should be a mainstay for traders in this effort.
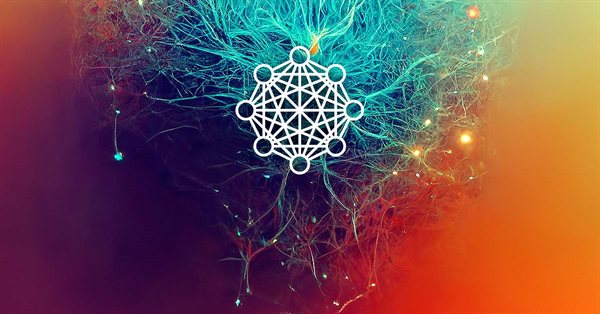
Neural networks made easy (Part 30): Genetic algorithms
Today I want to introduce you to a slightly different learning method. We can say that it is borrowed from Darwin's theory of evolution. It is probably less controllable than the previously discussed methods but it allows training non-differentiable models.
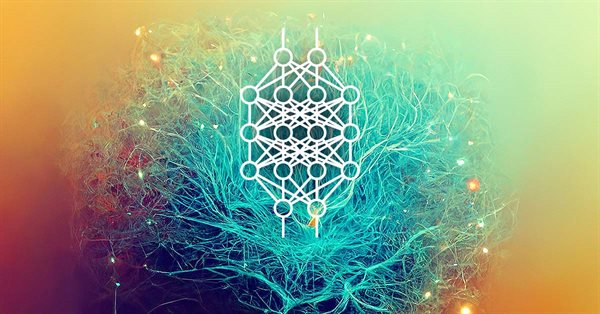
Neural networks made easy (Part 29): Advantage Actor-Critic algorithm
In the previous articles of this series, we have seen two reinforced learning algorithms. Each of them has its own advantages and disadvantages. As often happens in such cases, next comes the idea to combine both methods into an algorithm, using the best of the two. This would compensate for the shortcomings of each of them. One of such methods will be discussed in this article.