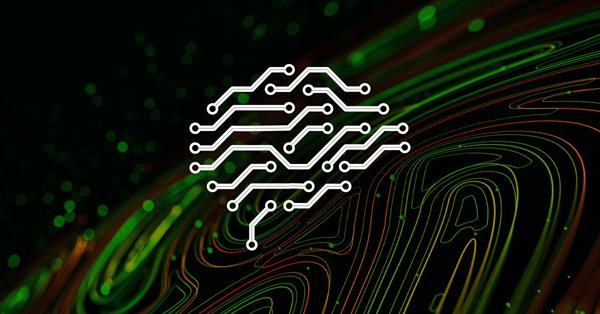
Experiments with neural networks (Part 2): Smart neural network optimization
Introduction
In the previous article Experiments with neural networks (Part 1): Revisiting geometry, I shared my neural network-related observations and experiments. Namely, I considered the question about what kind of data to pass to a neural network and demonstrated a simple example involving a perceptron to obtain a profitable trading system. I was successful at some points but also faced some difficulties, which are to be tackled here. Besides, I also want to move on to more complex neural networks. To achieve this, I will use the library from the article Programming a deep neural network from scratch using MQL language. The author described in great detail its working principles, so I will focus only on the main aspects. The main objective of this article is the development of a full-fledged trading robot based on a neural network and using MetaTrader 5 without third-party software.
Basics and examples
The author of the above library uses a deep neural network, but I propose to start small and build a network with the 4-4-3 structure. In total, we need (4 * 4) + 4 + (4 * 3) + 3 = 35 weights and bias values.
You can download the modified library below. I deliberately left all the changes in the code commented out so that you can see how to create custom neural networks.
Weight and bias values:
input double w0=1.0; input double w1=1.0; input double w2=1.0; input double w3=1.0; input double w4=1.0; input double w5=1.0; input double w6=1.0; input double w7=1.0; input double w8=1.0; input double w9=1.0; input double w10=1.0; input double w11=1.0; input double w12=1.0; input double w13=1.0; input double w14=1.0; input double w15=1.0; input double b0=1.0; input double b1=1.0; input double b2=1.0; input double b3=1.0; input double x0=1.0; input double x1=1.0; input double x2=1.0; input double x3=1.0; input double x4=1.0; input double x5=1.0; input double x6=1.0; input double x7=1.0; input double x8=1.0; input double x9=1.0; input double x10=1.0; input double x11=1.0; input double x12=1.0; input double x13=1.0; input double x14=1.0; input double x15=1.0; input double s0=1.0; input double s1=1.0; input double s2=1.0;
W and X are weights, B and S are bias parameters.
Include the neural network library:
#include <DeepNeuralNetwork2.mqh> int numInput=4; int numHiddenA = 4; int numOutput=3; DeepNeuralNetwork dnn(numInput,numHiddenA,numOutput);
Next, we will consider two examples from my previous article, namely one shape and one pattern with slope angles. Let's also look at the results of the library author's strategy. Finally, check all this on different neural networks - 4-4-3 and 4-4-4-3. In other words, we develop six EAs at once.
Pass the butterfly (envelope). Figure EA:
int error=CandlePatterns(ind_In1[1], ind_In1[10], ind_In2[1], ind_In2[10], _xValues);// Call the function int CandlePatterns(double v1,double v2,double v3,double v4,double &xInputs[])// Function { xInputs[0] = (v1-v2)/Point(); xInputs[1] = (v3-v4)/Point(); xInputs[2] = (v1-v4)/Point(); xInputs[3] = (v3-v2)/Point(); return(1); }
Pass the pattern with slope angles (four MA 1 and MA 24 indicator slope angles). Angle EA:
int error=CandlePatterns(ind_In1[1], ind_In1[5], ind_In1[10], ind_In2[1], ind_In2[5], ind_In2[10], _xValues);// Call the function int CandlePatterns(double v1,double v2,double v3,double v4,double v5,double v6,double &xInputs[])// Function { xInputs[0] = (((v1-v2)/Point())/5); xInputs[1] = (((v1-v3)/Point())/10); xInputs[2] = (((v4-v5)/Point())/5); xInputs[3] = (((v4-v6)/Point())/50); return(1); }
After using the strategy tester, set the optimization values for weights and biases from -1 to 1 with the step of 0.1. Get 3.68597592780611e+51 passes. Move on to the next section.
Solving optimization issues
When using the EA as described above, the strategy tester will conduct a little more than 10,000 passes in the (Slow complete algorithm) mode, which in our case is too small to optimize the neural network. I think, the (Fast genetic algorithm) mode is of no use here.
The main idea is to use only one variable for passes, like a counter of some sort. The remaining parameters of weights and biases are to be set inside the EA. For these purposes, I decided to try using a random number generator. In addition, it is necessary to remember which options we have already used in optimization.
The random number generator function:
double RNDfromCI(double minp, double maxp) { if(minp == maxp) return (minp); double Minp, Maxp; if(minp > maxp) { Minp = maxp; Maxp = minp; } else { Minp = minp; Maxp = maxp; } return (NormalizeDouble(double(Minp + ((Maxp - Minp) * (double)MathRand() / 32767.0)),1)); }
To remember the parameters of the pass, several options were considered. In particular, the array inside the EA is not suitable because it is reset to zero at each EA initialization. The global variables of the terminal are also not suitable due to the large amount of data. It is necessary to take out the results of the completed passes beyond the optimization process with the possibility of reading the data. I decided to use CSV files.
Let's introduce one variable for optimization:
input int Passages = 10000;
Optimize the Passages parameter from 1 to the maximum in increments of 1. Find out the maximum number of passes in the mode with one variable on a trial basis. It amounts to 100,000,000. I think, this is sufficient.
Initially, there was an idea to divide the EA into two - one for optimizing, the second for trading. But I think, a single EA will be more convenient. Add the mode switch:
input bool Optimization = true;
The main optimization code is located in the OnInit() EA initialization function:
if (Optimization==true){ int r=0; int q=0; while(q!=1 && !IsStopped()) { string str; while(r!=1 && !IsStopped()) { sw0=DoubleToString(RNDfromCI(Min, Max),1); sw1=DoubleToString(RNDfromCI(Min, Max),1); sw2=DoubleToString(RNDfromCI(Min, Max),1); sw3=DoubleToString(RNDfromCI(Min, Max),1); sw4=DoubleToString(RNDfromCI(Min, Max),1); sw5=DoubleToString(RNDfromCI(Min, Max),1); sw6=DoubleToString(RNDfromCI(Min, Max),1); sw7=DoubleToString(RNDfromCI(Min, Max),1); sw8=DoubleToString(RNDfromCI(Min, Max),1); sw9=DoubleToString(RNDfromCI(Min, Max),1); sw10=DoubleToString(RNDfromCI(Min, Max),1); sw11=DoubleToString(RNDfromCI(Min, Max),1); sw12=DoubleToString(RNDfromCI(Min, Max),1); sw13=DoubleToString(RNDfromCI(Min, Max),1); sw14=DoubleToString(RNDfromCI(Min, Max),1); sw15=DoubleToString(RNDfromCI(Min, Max),1); sb0=DoubleToString(RNDfromCI(Min, Max),1); sb1=DoubleToString(RNDfromCI(Min, Max),1); sb2=DoubleToString(RNDfromCI(Min, Max),1); sb3=DoubleToString(RNDfromCI(Min, Max),1); sx0=DoubleToString(RNDfromCI(Min, Max),1); sx1=DoubleToString(RNDfromCI(Min, Max),1); sx2=DoubleToString(RNDfromCI(Min, Max),1); sx3=DoubleToString(RNDfromCI(Min, Max),1); sx4=DoubleToString(RNDfromCI(Min, Max),1); sx5=DoubleToString(RNDfromCI(Min, Max),1); sx6=DoubleToString(RNDfromCI(Min, Max),1); sx7=DoubleToString(RNDfromCI(Min, Max),1); sx8=DoubleToString(RNDfromCI(Min, Max),1); sx9=DoubleToString(RNDfromCI(Min, Max),1); sx10=DoubleToString(RNDfromCI(Min, Max),1); sx11=DoubleToString(RNDfromCI(Min, Max),1); ss0=DoubleToString(RNDfromCI(Min, Max),1); ss1=DoubleToString(RNDfromCI(Min, Max),1); ss2=DoubleToString(RNDfromCI(Min, Max),1); if(StringFind(sw0,".", 0) == -1 ){sw0=sw0+".0";} if(StringFind(sw1,".", 0) == -1 ){sw1=sw1+".0";} if(StringFind(sw2,".", 0) == -1 ){sw2=sw2+".0";} if(StringFind(sw3,".", 0) == -1 ){sw3=sw3+".0";} if(StringFind(sw4,".", 0) == -1 ){sw4=sw4+".0";} if(StringFind(sw5,".", 0) == -1 ){sw5=sw5+".0";} if(StringFind(sw6,".", 0) == -1 ){sw6=sw6+".0";} if(StringFind(sw7,".", 0) == -1 ){sw7=sw7+".0";} if(StringFind(sw8,".", 0) == -1 ){sw8=sw8+".0";} if(StringFind(sw9,".", 0) == -1 ){sw9=sw9+".0";} if(StringFind(sw10,".", 0) == -1 ){sw10=sw10+".0";} if(StringFind(sw11,".", 0) == -1 ){sw11=sw11+".0";} if(StringFind(sw12,".", 0) == -1 ){sw12=sw12+".0";} if(StringFind(sw13,".", 0) == -1 ){sw13=sw13+".0";} if(StringFind(sw14,".", 0) == -1 ){sw14=sw14+".0";} if(StringFind(sw15,".", 0) == -1 ){sw15=sw15+".0";} if(StringFind(sb0,".", 0) == -1 ){sb0=sb0+".0";} if(StringFind(sb1,".", 0) == -1 ){sb1=sb1+".0";} if(StringFind(sb2,".", 0) == -1 ){sb2=sb2+".0";} if(StringFind(sb3,".", 0) == -1 ){sb3=sb3+".0";} if(StringFind(sx0,".", 0) == -1 ){sx0=sx0+".0";} if(StringFind(sx1,".", 0) == -1 ){sx1=sx1+".0";} if(StringFind(sx2,".", 0) == -1 ){sx2=sx2+".0";} if(StringFind(sx3,".", 0) == -1 ){sx3=sx3+".0";} if(StringFind(sx4,".", 0) == -1 ){sx4=sx4+".0";} if(StringFind(sx5,".", 0) == -1 ){sx5=sx5+".0";} if(StringFind(sx6,".", 0) == -1 ){sx6=sx6+".0";} if(StringFind(sx7,".", 0) == -1 ){sx7=sx7+".0";} if(StringFind(sx8,".", 0) == -1 ){sx8=sx8+".0";} if(StringFind(sx9,".", 0) == -1 ){sx9=sx9+".0";} if(StringFind(sx10,".", 0) == -1 ){sx10=sx10+".0";} if(StringFind(sx11,".", 0) == -1 ){sx11=sx11+".0";} if(StringFind(ss0,".", 0) == -1 ){ss0=ss0+".0";} if(StringFind(ss1,".", 0) == -1 ){ss1=ss1+".0";} if(StringFind(ss2,".", 0) == -1 ){ss2=ss2+".0";} str=sw0+","+sw1+","+sw2+","+sw3+","+sw4+","+sw5+","+sw6+","+sw7+","+sw8+","+sw9+","+sw10+","+sw11+","+sw12+","+sw13+","+sw14+","+sw15 +","+sb0+","+sb1+","+sb2+","+sb3 +","+sx0+","+sx1+","+sx2+","+sx3+","+sx4+","+sx5+","+sx6+","+sx7+","+sx8+","+sx9+","+sx10+","+sx11 +","+ss0+","+ss1+","+ss2; if (VerifyPassagesInFile(str)==true) { ResetLastError(); filehandle = FileOpen(OptimizationFileName,FILE_WRITE|FILE_READ|FILE_CSV|FILE_COMMON|FILE_ANSI, ";"); if(filehandle!=INVALID_HANDLE) { Print("FileOpen OK"); FileSeek(filehandle, 0, SEEK_END); FileWrite(filehandle,Passages,sw0,sw1,sw2,sw3,sw4,sw5,sw6,sw7,sw8,sw9,sw10,sw11,sw12,sw13,sw14,sw15, sb0,sb1,sb2,sb3, sx0,sx1,sx2,sx3,sx4,sx5,sx6,sx7,sx8,sx9,sx10,sx11, ss0,ss1,ss2); FileClose(filehandle); weight[0]=StringToDouble(sw0); weight[1]=StringToDouble(sw1); weight[2]=StringToDouble(sw2); weight[3]=StringToDouble(sw3); weight[4]=StringToDouble(sw4); weight[5]=StringToDouble(sw5); weight[6]=StringToDouble(sw6); weight[7]=StringToDouble(sw7); weight[8]=StringToDouble(sw8); weight[9]=StringToDouble(sw9); weight[10]=StringToDouble(sw10); weight[11]=StringToDouble(sw11); weight[12]=StringToDouble(sw12); weight[13]=StringToDouble(sw13); weight[14]=StringToDouble(sw14); weight[15]=StringToDouble(sw15); weight[16]=StringToDouble(sb0); weight[17]=StringToDouble(sb1); weight[18]=StringToDouble(sb2); weight[19]=StringToDouble(sb3); weight[20]=StringToDouble(sx0); weight[21]=StringToDouble(sx1); weight[22]=StringToDouble(sx2); weight[23]=StringToDouble(sx3); weight[24]=StringToDouble(sx4); weight[25]=StringToDouble(sx5); weight[26]=StringToDouble(sx6); weight[27]=StringToDouble(sx7); weight[28]=StringToDouble(sx8); weight[29]=StringToDouble(sx9); weight[30]=StringToDouble(sx10); weight[31]=StringToDouble(sx11); weight[32]=StringToDouble(ss0); weight[33]=StringToDouble(ss1); weight[34]=StringToDouble(ss2); r=1; q=1; } else { Print("FileOpen ERROR, error ",GetLastError()); q=0; } } } } }
Let me explain the code. Teaching the EA to wait for its turn to open a file turned out to be an exciting task. I encountered the issue of opening one file simultaneously in the optimization mode while using several CPU cores at once. This issue was solved in the first 'while' loop. The EA does not exit the OnInit() function until the file is opened for writing. It turned out that EAs are launched one by one during optimization.
While testing is underway on the first core, the EA can open a file for writing on the second one. Further on, assign random parameters in the range of Min and Max to all weights and biases. If the number turns out to be round, add .0 to it. Add all the values into a single 'str' string. Check the string using the VerifyPassagesInFile(str) function to see if there is the same string in the file. If not, then write it to the end of the file and fill in the weight[] arrays with the obtained random values of weights and biases.
The code of the function for checking parameters for their similarity to the previous ones:
bool VerifyPassagesInFile(string st){ string str=""; string str1=""; string str2=""; string str3=""; string str4=""; string str5=""; string str6=""; string str7=""; string str8=""; string str9=""; string str10=""; string str11=""; string str12=""; string str13=""; string str14=""; string str15=""; string str16=""; string str17=""; string str18=""; string str19=""; string str20=""; string str21=""; string str22=""; string str23=""; string str24=""; string str25=""; string str26=""; string str27=""; string str28=""; string str29=""; string str30=""; string str31=""; string str32=""; string str33=""; string str34=""; string str35=""; string str36=""; if (FileIsExist(OptimizationFileName)== true ){ ResetLastError(); filehandle = FileOpen(OptimizationFileName,FILE_WRITE|FILE_READ|FILE_CSV|FILE_COMMON|FILE_ANSI, ";"); if(filehandle!=INVALID_HANDLE) { Print("FileCreate OK"); } else Print("FileCreate ERROR, error ",GetLastError()); FileClose(filehandle); } ResetLastError(); filehandle = FileOpen(OptimizationFileName,FILE_WRITE|FILE_READ|FILE_CSV|FILE_COMMON|FILE_ANSI, ";"); if(filehandle!=INVALID_HANDLE) { Print("FileOpen OK"); } else Print("FileOpen ERROR, error ",GetLastError()); //--- read the data from the file while(!FileIsEnding(filehandle) && !IsStopped()) { str1=FileReadString(filehandle); str2=FileReadString(filehandle); str3=FileReadString(filehandle); str4=FileReadString(filehandle); str5=FileReadString(filehandle); str6=FileReadString(filehandle); str7=FileReadString(filehandle); str8=FileReadString(filehandle); str9=FileReadString(filehandle); str10=FileReadString(filehandle); str11=FileReadString(filehandle); str12=FileReadString(filehandle); str13=FileReadString(filehandle); str14=FileReadString(filehandle); str15=FileReadString(filehandle); str16=FileReadString(filehandle); str17=FileReadString(filehandle); str18=FileReadString(filehandle); str19=FileReadString(filehandle); str20=FileReadString(filehandle); str21=FileReadString(filehandle); str22=FileReadString(filehandle); str23=FileReadString(filehandle); str24=FileReadString(filehandle); str25=FileReadString(filehandle); str26=FileReadString(filehandle); str27=FileReadString(filehandle); str28=FileReadString(filehandle); str29=FileReadString(filehandle); str30=FileReadString(filehandle); str31=FileReadString(filehandle); str32=FileReadString(filehandle); str33=FileReadString(filehandle); str34=FileReadString(filehandle); str35=FileReadString(filehandle); str36=FileReadString(filehandle); str=str2+","+str3+","+str4+","+str5+","+str6+","+str7+","+str8+","+str9+","+str10+","+str11 +","+str12+","+str13+","+str14+","+str15+","+str16+","+str17+","+str18+","+str19+","+str20+","+str21 +","+str22+","+str23+","+str24+","+str25+","+str26+","+str27+","+str28+","+str29+","+str30+","+str31+","+str32+","+str33+","+str34+","+str35+","+str36; if (str==st){FileClose(filehandle); return(false);} } FileClose(filehandle); return(true); }
Here we first of all check if our FileIsExist(OptimizationFileName) file exists. If not, create it. Next, read the string in the while(!FileIsEnding(filehandle) && !IsStopped()) loop to the str1- strN variables. Put everything together to the 'str' variable. Compare each 'str' string with the incoming 'st' string at the function input. At the end, return the result, whether there is a match or not.
Resulting EA parameters:
"------------Open settings----------------";
Optimization - mode switch, optimize or trade;
Min - minimum value of the weights and biases parameters;
Max - maximum value of the weights and biases parameters;
OptimizationFileName - file name for writing parameters during optimization and reading in trading mode;
Passages - value of the passes. The optimization parameter from 1 to 100,000,000 maximum in increments of 1;
LL - Softmax function provides 3 results based on 100% sum. 0.6 equals to a value above 60%;
"------------Lots settings----------------";
SetFixLotOrPercent - select lot or deposit percentage;
LotsOrPercent - lot or percentage depending on SetFixLotOrPercent;
"------------Other settings---------------";
MaxSpread - maximum spread for opening an order;
Slippage - slippage;
Magic - magic number;
EAComment - comments to orders;
As you might know, MetaTrader 5 creates files in its sandbox by default.
The path to the files:
C:\Users\Your Username\AppData\Roaming\MetaQuotes\Terminal\Common\Files
Optimization
Let's perform the optimization. Period: from 2018.07.12 to 2021.07.12. EURUSD. H1, Open prices. Number of test runs: 10,000.
Mode: (Complex Criterion max). Angle EA 4-4-3
Mode: (Complex Criterion max). Angle EA 4-4-4-3
Mode: (Complex Criterion max). Figure EA 4-4-3
Mode: (Complex Criterion max). Figure EA 4-4-4-3
Mode: (Complex Criterion max). Original EA 4-4-3. Library author's strategy.
Mode: (Complex Criterion max). Original EA 4-4-4-3. Library author's strategy.
After optimization (for example 10,000 passes), we can continue filling our file with new parameters by starting a new optimization. Do not forget to save the strategy tester optimization report with the required Passages parameters beforehand. We also need to clear the terminal history, otherwise the tester will display the results of the already completed optimization. I do this with a .bat type script.
del C:\Users\Your Username\AppData\Roaming\MetaQuotes\Terminal\36A64B8C79A6163D85E6173B54096685\Tester\cache\*.* /q /f /s
for /d %%i in (C:\Users\Your Username\AppData\Roaming\MetaQuotes\Terminal\36A64B8C79A6163D85E6173B54096685\Tester\cache\*) do rd /s /q "%%i"
del C:\Users\Your Username\AppData\Roaming\MetaQuotes\Terminal\36A64B8C79A6163D85E6173B54096685\Tester\logs\*.* /q /f /s
for /d %%i in (C:\Users\Your Username\AppData\Roaming\MetaQuotes\Terminal\36A64B8C79A6163D85E6173B54096685\Tester\logs\*) do rd /s /q "%%i"
Replace (Your Username) and (36A64B8C79A6163D85E6173B54096685) with your own ones. You can open it with a regular text editor. The cleanup script is attached below.
Using optimization results
To check the optimization results, set the Optimization switch to 'false' and set the required pass index in the Passages parameter. To do this, simply double-click on the necessary result.
The code for uploading weights and biases parameters for testing:
if (FileIsExist(OptimizationFileName)==false){ int id = 0; int i = 0; string f1,f2,f3,f4,f5,f6,f7,f8,f9,f10,f11,f12,f13,f14,f15,f16,f17,f18,f19,f20,f21,f22,f23,f24,f25,f26,f27,f28,f29,f30,f31,f32,f33,f34,f35,f36; int handle = FileOpen(OptimizationFileName,FILE_WRITE|FILE_READ|FILE_CSV|FILE_COMMON|FILE_ANSI, ";"); if(handle!=INVALID_HANDLE) {Print("Loading optimization file."); while(!FileIsEnding(handle) && !IsStopped()) { f1=FileReadString(handle); f2=FileReadString(handle); f3=FileReadString(handle); f4=FileReadString(handle); f5=FileReadString(handle); f6=FileReadString(handle); f7=FileReadString(handle); f8=FileReadString(handle); f9=FileReadString(handle); f10=FileReadString(handle); f11=FileReadString(handle); f12=FileReadString(handle); f13=FileReadString(handle); f14=FileReadString(handle); f15=FileReadString(handle); f16=FileReadString(handle); f17=FileReadString(handle); f18=FileReadString(handle); f19=FileReadString(handle); f20=FileReadString(handle); f21=FileReadString(handle); f22=FileReadString(handle); f23=FileReadString(handle); f24=FileReadString(handle); f25=FileReadString(handle); f26=FileReadString(handle); f27=FileReadString(handle); f28=FileReadString(handle); f29=FileReadString(handle); f30=FileReadString(handle); f31=FileReadString(handle); f32=FileReadString(handle); f33=FileReadString(handle); f34=FileReadString(handle); f35=FileReadString(handle); f36=FileReadString(handle); if (StringToInteger(f1)==Passages){ weight[0]=StringToDouble(f2); Print(weight[0]); weight[1]=StringToDouble(f3); Print(weight[1]); weight[2]=StringToDouble(f4); Print(weight[2]); weight[3]=StringToDouble(f5); weight[4]=StringToDouble(f6); weight[5]=StringToDouble(f7); weight[6]=StringToDouble(f8); weight[7]=StringToDouble(f9); weight[8]=StringToDouble(f10); weight[9]=StringToDouble(f11); weight[10]=StringToDouble(f12); weight[11]=StringToDouble(f13); weight[12]=StringToDouble(f14); weight[13]=StringToDouble(f15); weight[14]=StringToDouble(f16); weight[15]=StringToDouble(f17); weight[16]=StringToDouble(f18); weight[17]=StringToDouble(f19); weight[18]=StringToDouble(f20); weight[19]=StringToDouble(f21); weight[20]=StringToDouble(f22); weight[21]=StringToDouble(f23); weight[22]=StringToDouble(f24); weight[23]=StringToDouble(f25); weight[24]=StringToDouble(f26); weight[25]=StringToDouble(f27); weight[26]=StringToDouble(f28); weight[27]=StringToDouble(f29); weight[28]=StringToDouble(f30); weight[29]=StringToDouble(f31); weight[30]=StringToDouble(f32); weight[31]=StringToDouble(f33); weight[32]=StringToDouble(f34); weight[33]=StringToDouble(f35); weight[34]=StringToDouble(f36); FileClose(handle); break; } } FileClose(handle); } else{ PrintFormat("Could not open file %s, error code = %d",OptimizationFileName,GetLastError()); return(INIT_FAILED); } }else{ PrintFormat("Could not open file %s, error code = %d",OptimizationFileName,GetLastError()); return(INIT_FAILED); }
Read the file when the Optimization switch is disabled. Compare the value of the first column with the value of the Passages parameter. If there is a match, assign the values of the weights and biases parameters to our weight[] arrays. Thus, we can test the best results.
Carry out forward testing of the obtained results to find the best three. In my case, the selection criteria are the maximum profit factor and the number of transactions being more than 100:
- Test interval: from 2021.07.12 to 2022.07.12;
- Mode: (Every tick based on real ticks);
- Initial deposit: 10,000;
- Timeframe: H1;
- Fixed lot 0.01;
- Angle EA 4-4-3.
Test 1:
Test 2:
Test 3:
- Test interval: from 2021.07.12 to 2022.07.12;
- Mode: (Every tick based on real ticks);
- Initial deposit: 10,000;
- Timeframe: H1;
- Fixed lot 0.01;
- Angle EA 4-4-4-3.
Test 1:
Test 2:
Test 3:
- Test interval: from 2021.07.12 to 2022.07.12;
- Mode: (Every tick based on real ticks);
- Initial deposit: 10,000;
- Timeframe: H1;
- Fixed lot 0.01;
- Figure EA 4-4-3.
Test 1:
Test 2:
Test 3:
- Test interval: from 2021.07.12 to 2022.07.12;
- Mode: (Every tick based on real ticks);
- Initial deposit: 10,000;
- Timeframe: H1;
- Fixed lot 0.01;
- Figure EA 4-4-4-3.
Test 1:
Test 2:
Test 3:
Next, test the original strategy of the library author in the neural networks 4-4-3 and 4-4-4-3.
- Test interval: from 2021.07.12 to 2022.07.12;
- Mode: (Every tick based on real ticks);
- Initial deposit: 10,000;
- Timeframe: H1;
- Fixed lot 0.01;
- Original EA 4-4-3.
Test 1:
Test 2:
Test 3:
- Test interval: from 2021.07.12 to 2022.07.12;
- Mode: (Every tick based on real ticks);
- Initial deposit: 10,000;
- Timeframe: H1;
- Fixed lot 0.01;
- Original EA 4-4-4-3.
Test 1:
Test 2:
Test 3:
As a result, the Angle EA 4-4-3 and Angle EA 4-4-4-3 strategies have worked better than Figure EA 4-4-3 and Figure EA 4-4-4-3. I think, the reason lies in their use of non-standard approaches to market analysis. Optimization on 2 cores takes about 20 minutes over a period of 3 years. Also, after the experiments, a number of questions arise that need to be addressed:
- Performing optimization on a large period.
- Increasing the number of passes.
- Deciding on the best strategy for moving forward.
- Developing an algorithm for simultaneously working with a certain database of trading optimization results. I have already started to think about it.
- Developing an algorithm for simultaneous optimization and trading.
- Using different timeframes to find the best result.
- Combining two or more neural networks with different data sets in one EA.
Of course, the full test requires a deeper training. But the obtained results speak for themselves. In addition, such experiments require large computational resources.
Conclusion
In the current article, we have moved on to more complex neural networks. A lot of work has been done to identify the necessary data to be passed to a neural network. But the possibilities do not end there. We need to try to pass data from a greater number of indicators and use complex links. I hope this will lead us to new successes in the development of a profitable trading robot. As it turns out, MetaTrader 5 can do without third-party software. In addition, I have developed a very interesting optimization algorithm that will expand our capabilities.
As usual, I will leave it up to you to carry out deeper optimization and forward testing.
Attachments:
Angle EA 4-4-3 - МA1 and МA24 indicator angle slopes strategy, neural network 4-4-3. Angle EA 4-4-4-3 - МA1 and МA24 indicator angle slopes strategy, neural network 4-4-4-3. Figure EA 4-4-3 - МA1 and МA24 indicator butterfly (envelope) strategy, neural network 4-4-3. Figure EA 4-4-4-3 - МA1 and МA24 indicator butterfly (envelope) strategy, neural network 4-4-4-3. Original EA 4-4-3 - candle size percentage strategy, neural network 4-4-3. Original EA 4-4-4-3 - candle size percentage strategy, neural network 4-4-4-3. Clear.bat - script for cleaning terminal files (Log and Cache). DeepNeuralNetwork – 4-4-4-3 neural network library. DeepNeuralNetwork2 – 4-4-3 neural network library.
Translated from Russian by MetaQuotes Ltd.
Original article: https://www.mql5.com/ru/articles/11186





- Free trading apps
- Over 8,000 signals for copying
- Economic news for exploring financial markets
You agree to website policy and terms of use
Roman,
thanks so much for your hard work! its amazing.
not sure if its possible considering your times and schedules... is it possible to add a logic to these EA?
so it would take trade on BUY ONLY , SHORT ONLY, BOTH? like the built in feature that MT4 has? couldn't find the builtin global setting in MT5.
I would like to get an extensive backtest but with having this additional logic, as Im trying to have biases for certain amount of time and stay long only , or short only for certain assets.
cheers.
Roman,
thanks so much for your hard work! its amazing.
not sure if its possible considering your times and schedules... is it possible to add a logic to these EA?
so it would take trade on BUY ONLY , SHORT ONLY, BOTH? like the built in feature that MT4 has? couldn't find the builtin global setting in MT5.
I would like to get an extensive backtest but with having this additional logic, as Im trying to have biases for certain amount of time and stay long only , or short only for certain assets.
cheers.
Thanks for the feedback. Write to ls. Everything is decided.
Hi Roman,
This is a great article and I am attempting to thoroughly understand it in anticipation of incorporating it into my existing EA. I hope you will be releasing further articles on this subject.
First, in your Angle 4-4-4-3.mq5 this test is checked against false
if (FileIsExist(OptimizationFileName)==false){
whereas in the Original EA 4-4-4-3 it is checked against true
if (FileIsExist(OptimizationFileName)==true){
More importantly, I am a complete newbie regarding DNN's; this is my first exposure to Neural Networks.
my plan is to use multiple strategies for evaluating buy conditions. Am I correct in assuming that each strategy might need a separate DNN or can the DNN expanded to provide evaluation of all strategies at the same time? In thinking about this, it seems that a Risk Reward function is needed to properly evaluate the best strategy to select for specific conditions, e.g. trending or flat markets. Is what I am considering result in a significantly larger and more complex network?
I have also developed a complex StopLoss function, for which I am considering a second separate instance of the DNN in order to maximize profits. Is this a better approach to including the inputs into one larger DNN.
Any comments you may have will be greatly Appreciated
CapeCoddah
Hi Roman,
This is a great article and I am attempting to thoroughly understand it in anticipation of incorporating it into my existing EA. I hope you will be releasing further articles on this subject.
First, in your Angle 4-4-4-3.mq5 this test is checked against false
if (FileIsExist(OptimizationFileName)==false){
whereas in the Original EA 4-4-4-3 it is checked against true
if (FileIsExist(OptimizationFileName)==true){
More importantly, I am a complete newbie regarding DNN's; this is my first exposure to Neural Networks.
my plan is to use multiple strategies for evaluating buy conditions. Am I correct in assuming that each strategy might need a separate DNN or can the DNN expanded to provide evaluation of all strategies at the same time? In thinking about this, it seems that a Risk Reward function is needed to properly evaluate the best strategy to select for specific conditions, e.g. trending or flat markets. Is what I am considering result in a significantly larger and more complex network?
I have also developed a complex StopLoss function, for which I am considering a second separate instance of the DNN in order to maximize profits. Is this a better approach to including the inputs into one larger DNN.
Any comments you may have will be greatly Appreciated
CapeCoddah
Hi. You can use the 8-4-3 or 16-4-3 network to expand your strategy. So you can add conditions. To do this, you need to modify the files. The network has 3 output values Sell, Buy and close. I think there is no need to separate buying and selling.
Hi Roman,
I started with your code, Angle 4443, but I soon realized that there is a glaring issue with your assumption of random testing, namely random testing requires a huge data set,namely 10 to 55th power to completely optimize the results. A 10,000 element data set only has a remote possibility of identifying a decent solution for each of the 55 neurons. But with Genetic optimization, the use of the best results combined with random mutations should provide faster initial identification of good results although probably not the most optimum. Consequently, I returned to the original work and chose a 4453 network and tried optimizing using EURUSD H4 with the time period from 2021 01 01 to 2023 01 01. I obtained some interesting results using my older 4 core cpu. First the complete run requires 75000 iterations and over 200 hours to complete. but I was able to identify good solutions after only 4 -8 hours, total equity of 2700 to 2900, based on an initial equity of 1000. In the last run, that ran almost 2 days, the equity reached 3336. I duplicated your test period and achieved new equity of 2788, although your test period was within my optimization period. I was using the original calculations as they seemed to work best. However, short gains achieved a 68% wins whereas longs only had about 45%. IN the last long run, there were 40,500 optimizations with 37,400 trades breaking even or producing gains whereas only 33150 trades produced a loss.
I did not loook at the money management aspect of the Original system. When I tried your Angle system on H4, the results bere abysmal. It looked like the stop loss function was failing miserably, probably due to the different time frame. Nearly all the runs ended with almost all losses.
I now planto run some sensitivity optimizations to see how changing the number of neurons in each layer affects the optimizations and also see how a 3 layer DNN compares to a4 layer one.
CapeCoddah